Blog
Getting through the last mile of generative AI agent development
There’s been a huge explosion in large language models (LLMs) over the past two years. It can be hard to keep up – much less figure out where the real value is happening. The performance of these LLMs is impressive, but how do they deliver consistent and reliable business results?
OpenAI demonstrated that very, very large models, trained on very large amounts of data, can be surprisingly useful. Since then, there’s been a lot of innovation in the commercial and open-source spaces; it seems like every other day there’s a new model that beats others on public benchmarks.
These days, most of the innovation in LLMs isn’t even really coming from the language part. It’s coming in three different places:
- Tool use: That’s the ability of the LLM to basically call functions. There’s a good argument to be made that tool use defines intelligence in humans and animals, so it’s pretty important.
- Task-specific innovations: This refers to innovations that deliver considerable improvements in some kind of very narrow domain, such as answering binary questions or summarizing research data.
- Multimodality: This is the ability of models to incorporate images, audio, and other kinds of non-text data as inputs.
New capabilities – and challenges
Two really exciting things emerge out of these innovations. First, it’s much easier to create prototypes of new solutions – instead of needing to collect data to make a Machine Learning (ML) model, you can just write a specification, or in other words, a prompt. Many solution providers are jumping on that quick prototyping process to roll out new features, which simply “wrap” a LLM and a prompt. Because the process is so fast and inexpensive, these so-called “feature factories” can create a bunch of new features and then see what sticks.
Second, making LLMs useful in real time relies on the LLM not using its “intrinsic knowledge” – that is, what it learned during training. It is more valuable instead to feed it contextually relevant data, and then have it produce a response based on that data. This is commonly called retrieval augmented generation, or RAG. So as a result, there are many companies making it easier to put your data inside the LLM – connecting it to search engines, databases, and more.

The thing about these rapidly developed capabilities is that they always place the burden of making the technology work on you and your organization. You need to evaluate if the LLM-based feature works for your business. You need to determine if the RAG-type solution solves a problem you have. And you need to figure out how to drive adoption. How do you then evaluate the performance of those things? And how many edge cases do you have to test to make sure it is dependable?
This “last mile” in the AI solution development and deployment process costs time and resources. It also requires specific expertise to get it right.
Getting over the finish line with a generative AI agent
High-quality LLMs are widely available. Their performance is dramatically improved by RAG. And it’s easier than ever to spin up new prototypes. That still doesn’t make it easy to develop a customer-facing generative AI solution that delivers reliable value. Enabling all of this new technology - namely, LLMs capable of using contextually relevant tools at their disposal - requires expertise to make sure that it works, and that it doesn’t cause more problems than it solves.
It takes a deep understanding of the performance of each system, how customers interact with the system, and how to reliably configure and customize the solution for your business. This expertise is where the real value comes from.
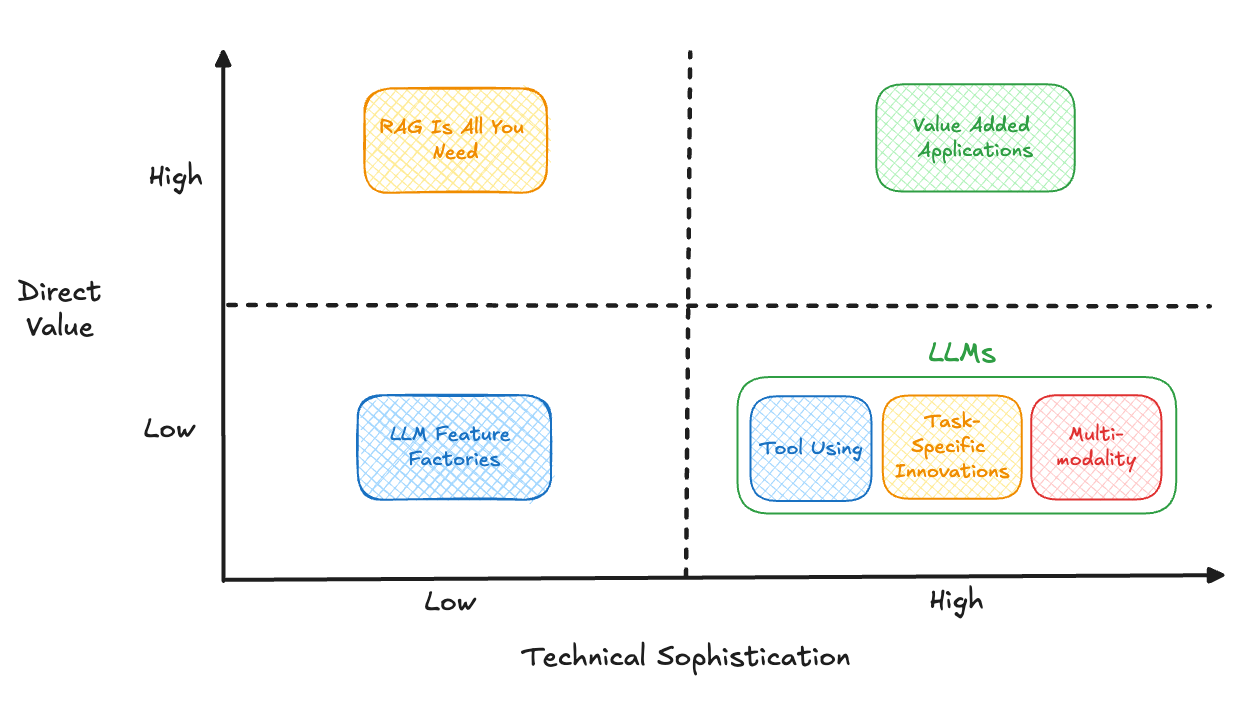
Plenty of solution providers can stand up an AI agent that uses the best LLMs enhanced with RAG to answer customers’ questions. But not all of them cover that last mile to make everything work for contact center purposes, and to work well, such that you can confidently deploy it to your customers, without worrying about your AI agent mishandling queries and causing customer frustration.
Generative AI services provided by the major public cloud providers can offer foundational capabilities. And feature factories churn out a lot of products. But neither one gets you across the finish line with a generative AI agent you can trust. Developing solutions that add value requires investing in input safety, output safety, appropriate language, task adherence, solution effectiveness, continued monitoring and refining, and more. And it takes significant technical sophistication to optimize the system to work for real consumers.
That should narrow your list of viable vendors for generative AI agents to those that don’t abandon you in the last mile.
A new era of unprecedented capacity in the contact center
Ever heard the phrase, "Customer service is broken?"
It's melodramatic, right? —something a Southern lawyer might declaim with a raised finger. Regardless, there’s some truth to it, and the reason is a deadly combination of interaction volume and staffing issues. Too many inbound interactions, too few people to handle them. The demands of scale do, in fact, break customer service.
This challenge of scaling up is a natural phenomenon. You find it everywhere, from customer service to pizza parlors.
Too much appetite, too little dough
If you want to scale a pizza, you have to stretch the dough, but you can't stretch it infinitely. There’s a limit. Stretch it too far, and it breaks.
Customer service isn't exactly physical, but physical beings deliver it— the kind who have bad days, sickness, and fatigue. When you stretch physical things too far (like balloons, hamstrings, or contact center agents), they break. In contact centers, broken agents lead to broken customer service.
Contact centers are currently stretched pretty thin. Sixty-three percent of them face staffing shortages. Why are they struggling? Some cite rising churn rates year after year. Others note shrinking agent workforces in North America and Europe. While workers flee agent jobs for coding classes, pastry school, and duck farming, customer request volumes are up. In 2022, McKinsey reported that 61% of customer care leaders claimed a growth in total calls.
To put it in pizza terms (because why not?), your agent dough ball is shrinking even as your customers' insatiable pizza appetite expands.
What’s a contact center to do? There are two predominant strategies right now:
- reduce request volumes (shrink the appetite)
- stretch your contact center’s service capacity (expand the dough)
Contact centers seem intent on squeezing more out of their digital self-service capabilities in an attempt to contain interactions and shrink agent queues. At the same time, they’re feverishly investing in technology to expand capacity with performance management, process automation and real-time agent support.
But even with both of these strategies working at full force, contact centers are struggling to keep up. Interaction volume continues to increase, while agent turnover carries on unabated. Too much appetite. Not enough dough to go around.
How do we make more dough?
Here’s the harsh reality – interaction volume isn’t going to slow down. Customers will always need support and service, and traditional self-service options can’t handle the scope and complexity of their needs. We’ll never reduce the appetite for customer service enough to solve the problem.
We need more dough. And that means we need to understand the recipe for customer service and find a way to scale it. The recipe is no secret. It’s what your best agents do every day:
- Listen to the customer
- Understand their needs
- Propose helpful solutions
- Take action to resolve the issue
The real question is, how do we scale the recipe up when staffing is already a serious challenge?
Scaling up the recipe for customer service
We need to scale up capacity in the contact center without scaling up the workforce. Until recently, that idea was little more than a pipe dream. But the emergence of generative AI agents has created new opportunities to solve the long-running problem of agent attrition and its impact on CX.
Generative AI agents are a perfect match for the task. Like your best human agents, they can and should listen, understand, propose solutions, and take action to resolve customers’ issues. When you combine these foundational capabilities into a generative AI agent to automate customer interactions, you expand your contact center’s capacity – without having to hire additional agents.
Here’s how generative AI tools can and should help you scale up the recipe for customer service:
- Generative AI should listen to the customer
Great customer service starts with listening. Your best agents engage in active listening to ensure that they take in every word the customer is saying. Transcription solutions powered by generative AI should do the same. The most advanced solutions combine speed and exceptional accuracy to capture conversations in the moment, even in challenging acoustic environments.
- Generative AI should understand the customer’s needs
Your best agents figure out what the customer wants by listening and interpreting what the customer says. An insights and summarization solution powered by generative AI can also determine customer intent, needs, and sentiment. The best ones don’t wait until after the conversation to generate the summary and related data. They do it in real time.
- Generative AI should propose helpful solutions
With effective listening and understanding capabilities in place, generative AI can provide real-time contextual guidance for agents. Throughout a customer interaction, agents perform a wide range of tasks – listening to the customer, interpreting their needs, accessing relevant information, problem-solving, and crafting responses that move the interaction toward resolution. It’s a lot to juggle. Generative AI that proposes helpful solutions at the right time can ease both the cognitive load and manual typing burden on agents, allowing them to focus more effectively on the customer.
- Generative AI should take action to resolve customers’ issues
This is where generative AI combines all of its capabilities to improve customer service. It can integrate the ingredients of customer care—listening, understanding, and proposing—to safely and autonomously act on complex customer interactions. More than a conversational bot, it can resolve customers’ issues by proposing and executing the right actions, and autonomously determining which backend systems to use to retrieve information and securely perform issue-resolving tasks.
Service with a stretch: Expanding your ball of dough
Many contact centers are already using generative AI to listen, understand, and propose. But it’s generative AI’s ability to take action based on those other qualities that dramatically stretches contact center capacity (without breaking your agents).
A growing number of brands have already rolled out fully capable generative AI agents that handle Tier 1 customer interactions autonomously from start to finish. That does more than augment your agents’ capabilities or increase efficiency in your workflows. It expands your frontline team without the endless drain of recruiting, onboarding, and training caused by high agent turnover.
A single generative AI agent can handle multiple interactions at the same time. And when paired with a human agent who provides direct oversight, a generative AI agent can achieve one-to-many concurrency even with voice interactions. So when inbound volume spikes, your generative AI agent scales up to handle it.
More dough. More capacity. All without stretching your employees to the breaking point. For contact center leaders, that really might be as good as pizza for life.
Want more? Read our eBook on the impact of agent churn
Agent churn rates are historically high, and the problem persists no matter what we throw at it — greater schedule flexibility, gamified performance dashboards, and even higher pay.
Instead of incremental changes to timeworn tools, what if we could bypass the problem altogether?
Download the Agent Churn: Go Through It or Around It? eBook to learn why traditional strategies for agent retention aren't working, and how generative AI enables a radical new paradigm.
Lessons learned from Altice’s successful digital messaging platform switch
Navigating any legacy platform transition can be nerve-wracking with little wiggle room for mistakes. As organizations increasingly adopt AI-powered solutions to enhance their customer experience, smoothing the process of switching platforms and optimizing user interactions requires careful planning, prioritization, and collaboration.
In the second installment of our two-part series based on a discussion between Melissa Price, VP of Customer Experience for Digital Self-Serve at Altice and Justin Mulhearn, Head of Solutions Engineering at ASAPP, we delve into the nuances of digital messaging platform transitions. Together, they share insights on overcoming challenges such as timing constraints, balancing automation with human support, and ensuring a smooth shift to a more advanced AI native platform. Through their experiences, they outline best practices for achieving long-term success while keeping user needs at the forefront.
Read Part 1: Why Altice switched messaging platforms: addressing flexibility, access, and support
* Minor edits have been made to the transcript for clarity and readability.
Adapting AI-powered messaging for user needs
Nick (moderator): We spoke earlier in the week, Melissa, about some of the ways we phased out the switch from your previous digital messaging platform to an AI native platform, or even just thinking about how to start and how to optimize over time.
I'd love for you to talk a little more in detail about how we got there, some of the things that were surprising, or moments when you had to prioritize this first, then get to other things later, while keeping the longer-term plan in mind.
Melissa: So some of the areas we delved into first were really about ensuring we're providing the right intent identifications, as we talked about earlier, and that we're in all the right places. The entry points were important to us to make sure we're there when customers need us and not just in the background, hoping the customer discovers us.
Being present in those moments that matter is something we continue to refine.
Whether that's in the app as backup support, leveraging chat there, or through our website, making chat available there. One example on the website is when customers are logged out and, say, they forgot their username or password and can’t log in to pay their bill. Being there in the moment to offer chat as the next line of support helps resolve the login issue or route them to an agent to resolve the issue more directly.
Balancing automation and human support
Melissa: Those were the types of things we wanted to uncover—what opportunities exist to drive more containment, where we can offer a quick reply or bot-based solution to the customer. Or if it reaches the next level of support, how do we identify those opportunities and get them directly to an agent?
Containment was definitely one of our goals that we reached, but containment in the right places and in the right way, I would say, not to misinterpret that stat. In some cases, containment is not a good option, and we need to rely on our chat agents to deliver the best experience.
Then there’s the surfacing of the entry point. Those are two big areas I’d highlight: intent identification and, up front, making sure customers can get to the right flow and resolution path as quickly as possible. That's also part of the consideration in terms of what's driving the AHT reduction.
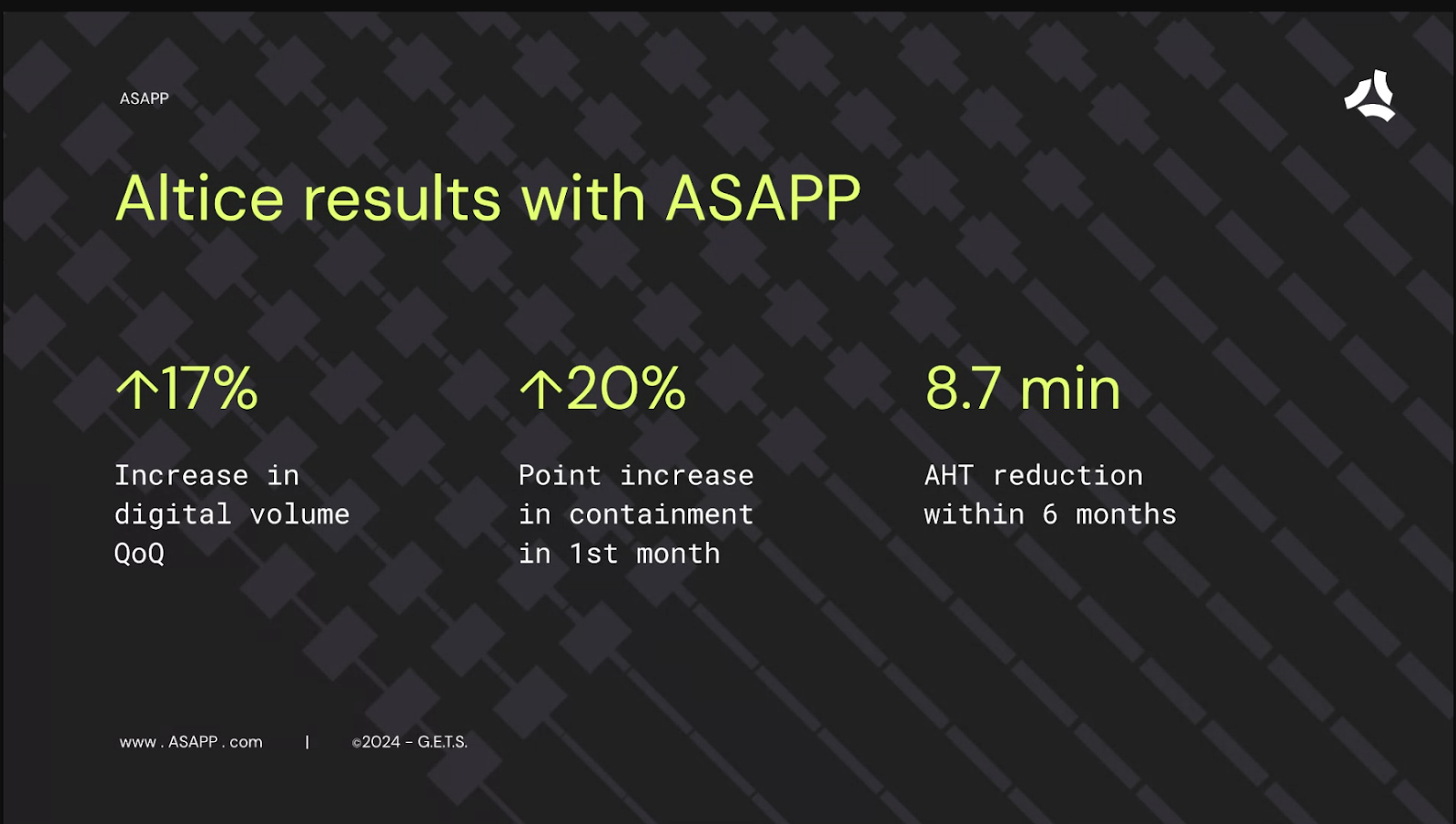
Nick: Yeah. It goes to the idea of focusing on the long-term gains. When you're optimizing and not pushing containment when it's not the right situation, that makes them come back to the channel, enjoy your brand, and have a delightful experience. So, thank you for sharing that.
Tips for navigating digital messaging platform transitions
Nick: But as I mentioned earlier, there's a lot of inertia. We know that moving a platform can be scary. Oftentimes, you're trying to manage timing considerations. So, I just want to say that we are mindful of the position you're in.
We've built it to allow you to get quick value and set up in under eight weeks. I know that’s a difficult thing for some other platforms to achieve, but you made the transition recently, Melissa. Justin, you've seen this a bunch of times. I’ll start with you, Justin. Do you have a few things in mind or some pointers on how to make these kinds of transitions easier, or even things people in our audience considering a platform switch should be thinking about?
Justin: Yeah, every situation's different, but thinking about some of the themes I’ve seen, the first piece of advice I would give is, at least in the beginning, skip the channels that aren't getting much use. I remember a couple of years ago when Google Business Messages was coming on the scene to compete with Apple Messages for Business, and everyone was excited about Facebook Messages as a support channel, and the same for Twitter DMs. If I look at 2024, those probably aren’t the areas getting the most attention. Google Messages is either turned off or soon to be defunct.
So I think about prioritization. If your customer base is in Latin America, WhatsApp should be your focus. If they’re primarily in the United States, maybe that’s not something you do on day one.
It’s about ordering priorities correctly and doing what will give you the biggest bang for your buck.
I would also say, as Melissa mentioned earlier regarding intents, you don’t necessarily need to replicate all your old flows when moving to a new platform. There’s definitely a place for classic deterministic flows. For something simple like, “What’s my bill balance?” you don’t need an LM to handle that. Sometimes, you may just want to navigate through a mini-system.
But as you look at your old deterministic flows, maybe you’ve got fifty in the old platform, but only need to bring over ten. For the rest, you can look to generative AI to handle those. Use that as an opportunity to save work and uplevel the experience.
The last thing would be embracing AI and machine learning for efficiency. Of course, I’m thinking about ASAPP’s platform here. There are many capabilities baked in, like giving agents a recommended response at the next turn of the conversation, or passing a question to a bot through automation.
There are opportunities like that, which are basically a training difference. It’s worth spending the time and giving agents the opportunity to use those tools so you can see that big efficiency bump as you transition.
Nick: Melissa, if I remember correctly, when we were engaging in that transition, timing was pretty important. I think we had a tight deadline. Could you tell us a bit more about that? And if you were giving advice to someone in the audience who’s in the same shoes you were in a year and a half ago, what kind of advice would you give them?
Timing for a successful digital messaging platform launch
Melissa: Yeah, I mean, that’s one of the more nerve-wracking things when you're transitioning — making sure you hit that deadline because you’re cutting off your old platform, and moving on to the new one, with very little wiggle room for mistakes.
What was important to us as we thought about the planning behind it was making sure that, between ASAPP, my team on the digital side, and our IT teams, we were all aligned on that plan.
We were all aligned on the timing and what the level of effort would be to do that. Grounding ourselves first on what was truly MVP for this launch, and making sure we took into consideration if it was just a like-for-like? Or if there was room for a like-for-better? Do we have room for like-for-better? So those were some of the things we were looking at, and then what the use cases were if there was an opportunity to augment the platform a little bit more on day one.
But then when it came to actual execution and delivery, just really making sure that you’re running point and the partnership - there is the important piece again to highlight that. But they said one of the things that we appreciated was the extra level of effort that kinda went into them, like, really leaning in on that transition plan and making sure that we were able to meet that deadline. It came from our teams as well.
People wear many hats when it came to that last two to three weeks time period to make sure that we got all of our testing done and passed on time. And, I mean, happy to report, we launched with no major defects. There was one defect that we had post launch, and it was a small one. So it was really because of the collaboration across the teams.
Nick: I love to hear that. I mean, it really was a collaborative effort. I love hearing stories of customers saying that we're a good partner in helping with that. I think it touches on something that Justin mentioned earlier. It's not just the product and the features.
It's actually the partner that you get in the platform vendor.
I think it's a big part of how we think of things and how we support our customers.
Why Altice switched messaging platforms: addressing flexibility, access, and support
Delivering a seamless digital customer experience is no small task—especially when existing platforms fall short. From limited automation capabilities to challenges with accessibility and support, organizations often encounter roadblocks that hinder both customer satisfaction and operational efficiency. When these gaps become too significant, making a platform transition becomes necessary.
In this first installment of our two-part series based on a discussion between Melissa Price, VP of Customer Experience for Digital Self-Serve at Altice and Justin Mulhearn, Head of Solutions Engineering at ASAPP, we explore the realities of switching messaging platforms. They share firsthand insights into the key pain points that led Altice to make a change, the biggest hurdles organizations face in these transitions, and how to approach the decision-making process strategically.
Read Part 2: Lessons learned from Altice’s successful digital messaging platform switch
* Minor edits have been made to the transcript for clarity and readability.
Switching digital messaging platforms: Altice’s experience
Nick (moderator): Melisa, you made the switch a little over a year ago. You were using another platform, and I understand Altice had some issues with it. I'd love to hear about some of the recurring frustrations you faced—or were there a handful of last straws that made you say, ‘Okay, we’ve known this was a problem, but now we have to make a change’?
Melissa: Yeah. Happy to share that. There are three areas specifically that I'll highlight.
One was around just the limited flexibility. Limited flexibility in terms of some of the insights that we were able to gather, and being able to go deeply enough to uncover the root of some of these opportunities, and how we would continue to grow in the chat space and deliver better experiences. And then how we present that to our customers and where we surface chat.
The other one was really around the access. Limited access in terms of being able to generate more flexibility for my team to then be able to make quick, continuous improvement efforts through the chat flows. And just making sure that we had the ability to really test and learn, and really make these real-time changes.
And then that last one, I would say, is just the engagement model.
If we were gonna start fresh with a new partner, we wanted to make sure that we had a better engagement model at the onset of it, and somebody who’s really looking at this as a partnership.
Nick: Justin, I know you've helped a bunch of customers make platform switches to ASAPP. Does this resonate? What else is common, or what are some of the other things you see in other platforms that hold them back from success?
Justin: Yeah, this definitely resonates. There are many inefficiencies and problems that we see as we’re working with these legacy platforms and programs. But to Melissa's point, oftentimes it's just about the account support. Sometimes it’s not even the technology.
But if your customer success team isn't there with you every single week, bringing you new features or really working with you on a continual basis, odds are the program’s not gonna reach its potential.
In terms of the programs themselves, this is both operational and technological.
I’ve seen a lot of programs out there that were never really optimized for digital and are still coming in with a voice mindset - If you have a program where you're taking chats, and it's a one-to-one concurrency like you had with voice, you’re basically getting no value out of the program.
But in a lot of programs, they tend to top out at a 1.2 to 1.3 level concurrency, and never really get beyond that. I think a lot of that does have to do with the underlying technology of the platforms themselves. From a basic perspective, you can have multiple concurrent conversations going on, but mental task switching between different subjects can be pretty difficult. So you really need the tooling and other support to help you shift rapidly back and forth between conversations as an agent.
The importance of context in customer experience
Justin: And I’ll also say that, from the end customer's perspective, when I see these legacy programs where there’s no context being passed from a bot to a live agent, that always drives me nuts. That drives me nuts as a customer as well.
And then, oftentimes, I find that even when it gets to the live agent, the agents aren’t empowered to do the same things that they could do on the phone, which is just the most disappointing. “Hey, I just spent ten minutes getting to this point in the conversation only to find out that the agent can't actually help me.”
I think, Melissa, you were talking a little bit about that earlier this week. How have you dealt with that agent empowerment piece?
Melissa: I think context is so important.
As you look at every handoff part of the process, making sure that you're delivering it through the lens of where the customer came from, and having a common understanding of what may have been attempted before. That's an area we're continuing to work on, but not something that would be solved overnight. But, the context is so important, and then how that ties in not just to the chat platform, but also the tools that our agents would use, whether it be on the chat side or whether it be on the voice side.
Keys to success in digital customer experience
Nick: Melissa, you’ve touched on some key aspects of what makes digital success possible. On the flip side, when you were considering making a switch to a new platform, what were the main things you were looking for in terms of both the engagement model and other key details to ensure success?
Melissa: We have a team that really is data focused, data led, and customer focused.
But I think when we’re looking at another partner for a chat solution, it was really important that the ideas and insights didn’t just come from my team, but also from whoever we’d be partnering with.
It was important to ensure that there were other insights brought forth proactively to help further understand different ways of thinking about the problems to solve. How do we uncover the root of some of these issues? What drivers may be behind containment, low containment, or poor experiences, as surfaced through NPS or other feedback from customers?
So, we were really trying to look at different angles to uncover opportunities and make sure we’re addressing the root causes. And then, partnering closely on finding a more optimized solution, based on key learnings from other clients or customers, with the potential to carry those insights into our work as well.
Nick: I know we talked earlier in the week about not just optimizing for digital, but even within digital, which I think really gets into how you best personalize and make the experience right for the customer in that specific moment and situation.
Melissa: Yeah, and if I could just share one specific example, it may seem small, but it can have an impact. When the customer is first interacting and engaging with us, initiating a chat, the intent is key. So, being able to uncover the right intent. We give customers the option to either select a list of canned intents based on our understanding of the context and entry point they may be coming from, or they have the free text option. But, it's about finding the right balance between how much we lean on the free text versus how much we’re continually updating and refining the canned intent list.
Those are things that we continue to cycle through to make sure we're delivering the best experience upfront, recognizing that when the customer’s trying to interact with us, we want to get them to the right place as soon as possible.
Watch the rest of the webinar.
Not all contact center automation is the same
At ASAPP we develop AI models to improve agent performance in the contact center. Many of these models directly assist contact center agents by automating parts of their workflow. For example, the automated responses generated by AutoCompose - part of our ASAPPMessaging platform - suggest to an agent what to say at a given point during a customer conversation. Agents often use our suggestions by clicking and sending them.
How we measure performance matters
While usage of the suggestions is a great indicator of whether the agents like the features, we’re even more interested in the impact the automation has on performance metrics like agent handle time, concurrency, and throughput. These metrics are ultimately how we measure agent performance when evaluating the impact of a product like the AutoCompose capabilities of ASAPPMessaging, but these metrics can be affected by things beyond AutoCompose usage, like changes in customer intents or poorly-planned workforce management.
To isolate the impact of AutoCompose usage on agent efficiency, we prefer to measure the specific performance gains from each individual usage of AutoCompose. We do this by measuring the impact of automated responses on agent response time, because response time is more invariant to intent shifts and organizational effects than handle time, concurrency and throughput.
By doing this, we can further analyze:
- The types of agent utterances that are most often automated
- The impact of the automated responses when different types of messages are used (in terms of time savings)
Altogether, this enables us to be data-driven about how we improve models and develop new features to have maximum impact.
Going beyond greeting and closing messages
When we train AI models to automate responses for agents, the models look for patterns in the data that can predict what to say next based on past conversation language. So the easiest things for models to learn well are the types of messages that occur often and without much variation across different types of conversations, e.g. greetings and closings. Agents typically greet and end a conversation with a customer the same way, perhaps with some specificity based on the customer’s intent.

Most AI-driven automated response products will correctly suggest greeting and closing messages at the correct time in the conversation. This typically accounts for the first 10-20% of automated response usage rates. But when we evaluate the impact of automating those types of messages, we see that it’s minimal.
To understand this, let’s look at how we measure impact. We compare agents’ response times when using automated responses against their response times when not using automated responses. The difference in time is the impact—it’s the time savings we can credit to the automation.

Without automation, agents are not manually typing greeting and closing messages for every conversation. Rather they’re copying and pasting from notepad or word documents containing their favorite messages. Agents are effective at this because they do it several times per conversation. They know exactly where their favorite messages are located, and they can quickly copy and paste them into their chat window. Each greeting or closing message might take an agent 2 seconds. When we automate those types of messages, all we are actually automating is the 2-second copy/paste. So when we see automation rates of 10-20%, we are likely only seeing a minimal impact on agent performance.
The impact lies in automating the middle of the conversation.
If automating the beginnings and endings of conversations is not that impactful, what is?

Automating the middle of the conversation is where response times are naturally slowest and where automation can yield the most agent performance impact.
- Heather Reed, Product Manager, ASAPP
The agent may not know exactly what to say next, requiring time to think or look up the right answers. It’s unlikely that the agent has a script readily available for copying or pasting. If they do, they are not nearly as efficient as they are with their frequently used greetings and closings.
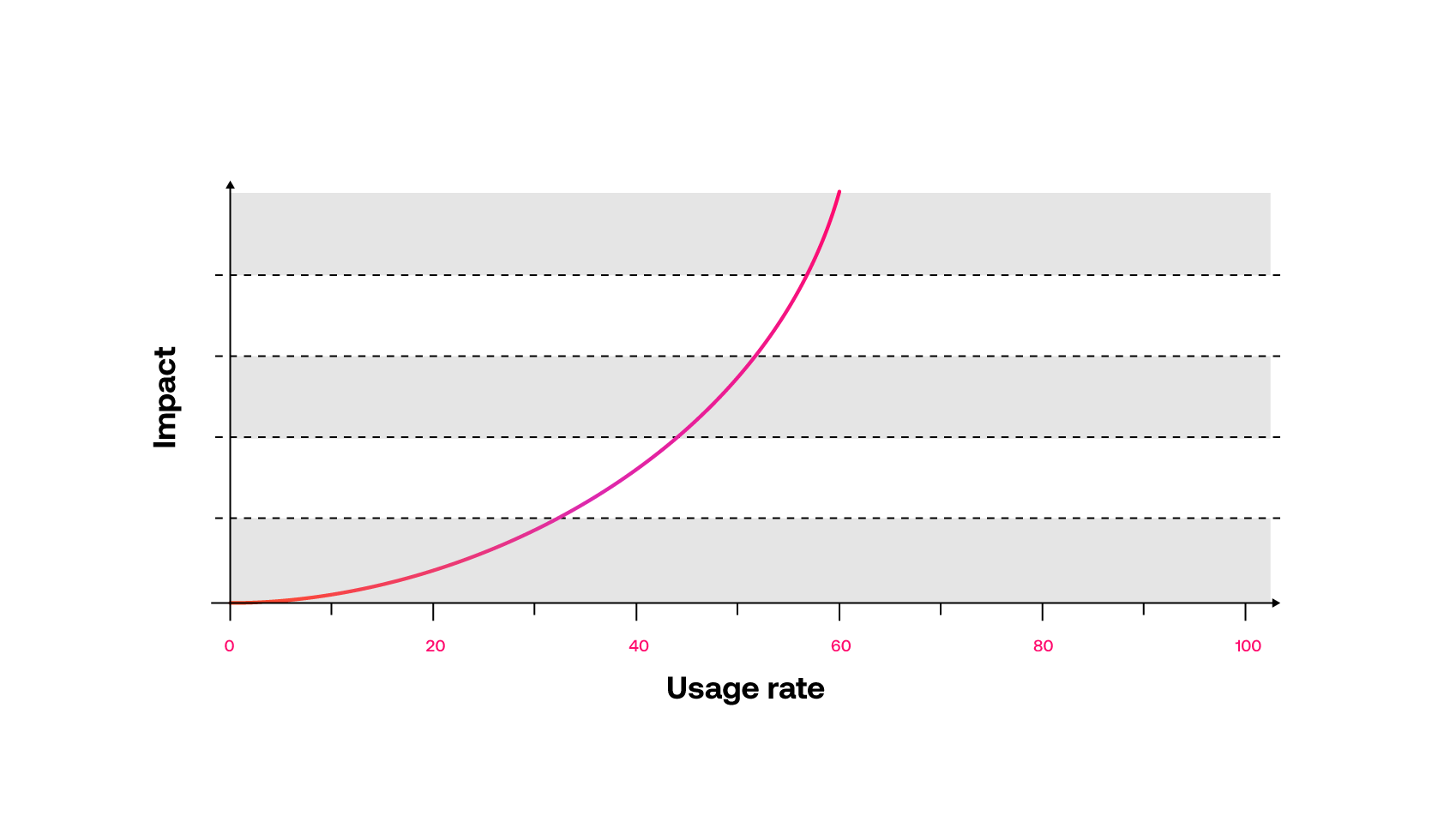
Where it was easy for AI models to learn the beginnings and endings of conversations, because they most often occur the same way, the exact opposite is true of the middle parts of conversations. Often, this is where the most diversity in dialog occurs. Agents handle a variety of customer problems, and they solve them in a variety of ways. This results in extremely varied language throughout the middle parts of conversations, making it hard for AI models to predict what to say at the right time.
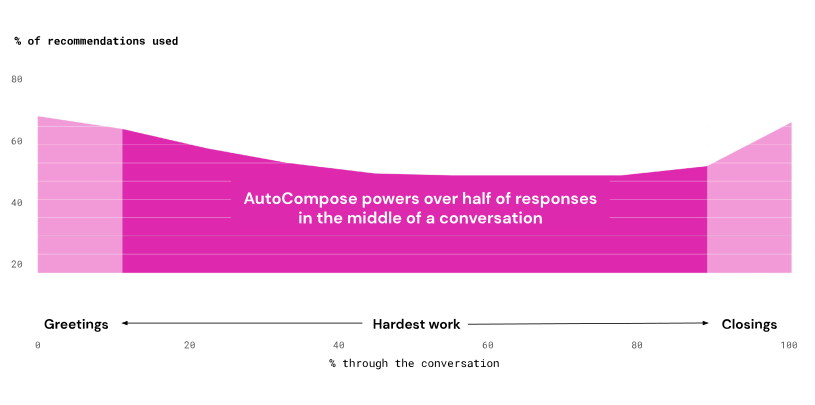
ASAPP’s research delivers the biggest improvements
Whole interaction models are exactly what the research team at ASAPP specializes in developing. And it’s the reason that AutoCompose is so effective. If we look at AutoCompose usage rates throughout a conversation, we see that while there is a higher usage at the beginnings and endings of conversations, AutoCompose still automates over half of agent responses in between.
The low response times in the middle of conversations are where we see the biggest improvements in agent response time. It’s also where the biggest opportunities for improvements are realized.
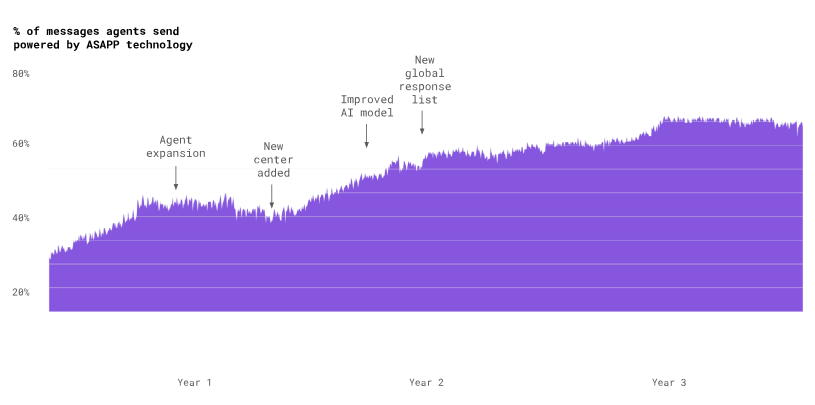
Whole interaction automation shows widespread improvements
ASAPP’s current automated response rate is about 80%. It has taken a lot of model improvements, new features, and user-tested designs to get there. But now agents effectively use our automated responses in the messaging platform to reduce handle times by 25%, enabling an additional 15% of agent concurrency, for a combined improvement in throughput of 53%. The AI model continues to get better with use, improving suggestions, and becoming more useful to agents and customers.
Ensuring generative AI agent success with human-in-the-loop
A bridge to the future
Talk of Generative AI transforming contact centers is everywhere. From boardrooms of the world’s largest enterprises to middle management and frontline agents, there’s a near-universal consensus: the impact will be massive. Yet, the path from today’s reality to that future vision is full of challenges.
The key to bringing customer-facing Gen AI to production today lies in a human-in-the-loop workflow, where AI agents consult human advisors whenever needed—just as frontline agents might seek guidance from a tier 2 agent or supervisor. This approach both unlocks benefit today while providing a self-learning mechanism driving greater automation in the future.
Challenges today
We've spilled our fair share of digital ink on how Generative AI marks a step change in automation and how it will revolutionize the contact center. But let's take a moment to acknowledge the key challenges that must be overcome to realize this vision.
- Safety: The stochastic nature of AI means it can "hallucinate"—producing incorrect or misleading outputs.
- Authority: Not every customer interaction is ready for full automation; certain decisions still require human judgment.
- Customer request: Regardless of how advanced an AI agent is, some customers will insist on speaking with a human agent.
- Access: Many systems used by agents today to resolve customer issues lack APIs and are thus inaccessible to Gen AI agents.
Safety: Constant vigilance
AI's ability to generate human-like responses makes it powerful, but this capability comes with risks. Gen AI systems are inherently probabilistic, meaning they can occasionally hallucinate—providing incorrect or misleading information. These mistakes, if unchecked, can erode customer trust at best and damage a brand at worst.
When evaluating a Gen AI system, the critical question to ask is not “will the system hallucinate?” It will. The critical question is, “will hallucinated output get sent to my customers?”
With GenerativeAgent® every outgoing message is evaluated by an output safety module that, among other things, checks for hallucinations. If a possible hallucination is detected, the message is assigned to a human-in-the-loop advisor for review. This safeguard prevents the dissemination of erroneous information while allowing the virtual agent to maintain control of the interaction.
In systems without this kind of human oversight, mistakes could lead to escalations or, worse, unresolved customer issues, or worse yet, damaged customer relationships or brand. By integrating a human-in-the-loop workflow, businesses can mitigate the risks inherent to this technology while maximizing the business benefits it offers.
Authority: Responsible delegation
Deploying customer-facing Gen AI in a contact center doesn’t mean relinquishing all decision-making power to the AI. There are situations where human judgment is crucial, particularly when the stakes are high for both the business and the customer.
Take, for example, a customer requesting a payment extension or a final review of their loan application. These are pivotal decisions that involve weighing factors like risk, compliance, and customer loyalty. While a Gen AI agent can automate much of the interaction leading up to these moments, the final decision still requires human judgment.
With a human-in-the-loop framework, this is no longer a limitation. The AI agent can seamlessly consult an advisor for approval while maintaining control of the customer interaction, much like a Tier 1 agent consulting a supervisor. This approach provides flexibility—automating what can be automated while reserving human intervention for critical decisions. For the business, this boosts automation rates, reduces escalations, and empowers advisors to focus on high-impact judgments where their expertise is essential.

Customer request: Last ditch containment
No matter how advanced Gen AI virtual agents become, some customers will still ask to speak with a live agent—at least for now. Of all the challenges outlined, this one is the most intractable, driven by customer habits and expectations rather than technology or process.
Human-in-the-loop offers a smart alternative. Rather than instantly escalating to a live agent when requested, the Gen AI agent could inform the customer of the wait time and propose a review by a human advisor in the meantime. If the advisor can help the Gen AI agent resolve the issue quickly, the escalation can be avoided entirely—delivering a faster resolution without disrupting the flow of the interaction.
While not every customer will accept this deflection, it can significantly reduce this type of escalation and address one of the toughest barriers to full automation.
Access: Ready for today, better tomorrow
Many systems that agents rely on today lack APIs, putting a large volume of customer issues beyond the reach of automation.
Rather than dismissing these cases, a human-in-the-loop advisor can bridge this gap—handling tasks on behalf of the Gen AI agent in systems that lack APIs.
The value extends beyond expanding automation. It highlights areas where developing APIs or streamlining access to legacy systems could deliver significant ROI. What was once hard to justify becomes clear: introducing new APIs directly reduces the advisor hours spent compensating for their absence.
Conclusion
Generative AI is poised to reshape the contact center, but unlocking its full potential requires a tightly integrated human-in-the-loop workflow. This framework ensures that customer-facing Gen AI is ready for production today while providing a self-learning mechanism driving to greater automation gains tomorrow.
While AI technology continues to advance, the role of human advisors is not diminishing—it’s evolving. By embracing this collaboration, businesses can strike the right balance between automation and human judgment, leading to more efficient operations and better customer experiences.
In future posts on human-in-the-loop, we’ll dive deeper into how to enhance collaboration between AI and human agents, and explore the evolving role of humans in AI-driven contact centers.
AI security and AI safety: Navigating the landscape for trustworthy generative AI
AI security & AI safety
In the rapidly evolving landscape of generative AI, the terms "security" and "safety" often crop up. While they might sound synonymous, they represent two distinct aspects of AI that demand attention for a comprehensive and trustworthy AI system. Let's dive into these concepts and explore how they shape the development and deployment of generative AI, using real-world examples from contact centers to make sense of these crucial elements. To start, here is a quick overview video on AI security and AI safety:
AI security: The shield against malicious threats
When we think about AI security, it's crucial to differentiate between novel AI-specific risks and security risks that are common across all types of applications, not just AI.
The reality is that over 90% of AI security efforts are dedicated to addressing critical basics and foundational security controls. These include data protection, encryption, data retention, PII redaction, authorization, and secure APIs. It’s important to understand that while novel AI-specific threats like prompt injection - where a malicious actor manipulates input to retrieve unauthorized data or inject system commands - do exist, they represent a smaller portion of the overall security landscape.
Let's consider a contact center chatbot powered by AI. A user might attempt to embed harmful scripts within their query, aiming to manipulate the AI into disclosing sensitive customer information, like social security numbers or credit card details. While this novel threat is significant, the primary defense lies in robust foundational security measures. These include input validation, strong data protection, employing encryption for sensitive information, and implementing strict authorization and data access controls.
Secure API access is another essential cornerstone. Ensuring that all API endpoints are authenticated and authorized prevents unauthorized access and data breaches. In addition to these basics, implementing multiple layers of defense helps mitigate novel threats. Input safety mechanisms can detect and block exploit attempts, preventing abuse like prompt leaks and code injections. Advanced Web Application Firewalls (WAFs) also play a vital role in defending against injection attacks, similar to defending against common application threats like SQL injection.
Continuous monitoring and logging of all interactions with the AI system is very important in detecting any suspicious activities. For example, an alert system can flag unusual API access patterns or data requests by an AI system, enabling rapid response to potential threats. Furthermore, a solid incident response plan is indispensable. It allows the security team to swiftly contain and mitigate the impact of any security events or breaches.
So while novel AI-specific risks do pose a threat, the lion’s share of AI security focuses on foundational security measures that are universal across all applications. By getting the basics right we build a robust shield around our AI systems, ensuring they remain resilient against both traditional and emerging threats.
AI safety: The guardrails for ethical and reliable AI
While AI security acts as a shield, AI safety functions like guardrails, ensuring the AI operates ethically and reliably. This involves measures to prevent unintended harm, ensure fairness, and adhere to ethical guidelines.
Imagine a scenario where an AI Agent in a contact center is tasked with prioritizing customer support tickets. Without proper safety measures, the AI could inadvertently favor tickets from specific types of customers, perhaps due to biased training data that inadvertently emphasizes certain demographics or issues. This could result in longer wait times and dissatisfaction for overlooked customers. To combat this, organizations should implement bias mitigation techniques, such as diverse training datasets. Regular audits and red teaming are essential to identify and rectify any inherent biases, promoting fair and just AI outputs. Establishing and adhering to ethical guidelines further ensures that the AI does not produce unfair or misleading prioritization decisions.
An important aspect of AI safety is addressing AI hallucinations, where the AI generates outputs that aren't grounded in reality or intended context. This can result in the AI fabricating information or providing incorrect responses. For instance, a customer service AI Agent might confidently present incorrect policy details if it isn't properly trained and grounded. Output safety layers and content filters play a crucial role here, monitoring outputs to catch and block any harmful or inappropriate content.
Implementing a human-in-the-loop process adds another layer of protection. Human operators can be called on to intervene when necessary, ensuring critical decisions are accurate and ethical. For example, contact center human agents can be the final step of authorization before performing a critical task, or providing additional insight when the AI system produces incorrect output or does not have enough information to support a user.
The intersection of security and safety
Though AI security and AI safety address different aspects of AI operation, they often overlap. A breach in AI security can lead to safety concerns if malicious actors manage to manipulate the AI's outputs. Conversely, inadequate safety measures can expose the system to security threats by allowing the AI to make incorrect or dangerous decisions.
Consider a scenario where a breach allows unauthorized access to the contact center’s AI system. The attackers could manipulate the AI to route calls improperly, causing delays and customer frustration. Conversely, if the AI's safety protocols are weak, it might inaccurately redirect emergency calls to non-critical queues, posing serious risks. Therefore, a balanced approach that addresses both security and safety is essential for developing a trustworthy generative AI solution.
Balanced approach for trustworthy AI
Understanding the distinction between AI security and AI safety is pivotal for building robust AI systems. Security measures protect the AI system from external threats, ensuring the integrity, confidentiality, and availability of data. Meanwhile, safety measures ensure that the AI operates ethically, producing accurate outputs.
By focusing on both security and safety, organizations can mitigate risks, enhance user trust, and responsibly unlock the full potential of generative AI. This dual focus ensures not only the operational integrity of AI systems but also their ethical and fair use, paving the road for a future where AI technologies are secure, reliable, and trustworthy.
Three reasons you're not seeing the value you were promised from your digital chat platform.
Are you not getting the value that you were hoping for from your digital chat platform?
If so, this blog and our recent on-demand webinar are for you.
We recently had a great webinar featuring Melissa Price, VP, CX Digital Self-Service at Altice USA and the experts at ASAPP. In it, we talked about what is holding people back from seeing the value they want out of their existing digital chat platform, what they should look for in a new one, and how they can make that migration as painless as possible.
The conversation is ripe with solutions for some of the widespread pain points CX leaders are experiencing with their digital chat platforms.
Takeaways from the Webinar
3 Underlying Challenges Holding Platforms Back
In the webinar, we discussed three critical issues that often prevent customer engagement platforms from succeeding:
- Fragmented Customer Journeys: Disconnected touchpoints lead to inconsistent customer experiences. Automation on many platforms is not customer-friendly or efficient, often leading to frustration rather than satisfaction.
- Lack of Adaptability: Many platforms suffer from a lack of ongoing innovation and adaptability. Without continuous improvement loops and the support to keep up with evolving needs, platforms become outdated quickly and fail to deliver the optimized experiences necessary for customer retention and satisfaction. This static approach that many platforms offer contrasts sharply with the dynamic nature of customer service needs.
- Limited Effectiveness of Bolted-on AI: Many legacy chat providers rely on bolted-on AI solutions rather than being AI-native, resulting in subpar suggested responses, ultimately failing to deliver the additional efficiencies, predictive insights, and automation that AI should provide.
How Modern Chat Platforms, Like ASAPP, Address These Problems
Moder, AI-Native® digital chat platforms, like ASAPPMessaging tackles these challenges head-on:
- Seamless customer experience Integration: ASAPP Messaging unifies customer interactions, ensuring a cohesive journey.
- Continuously improving AI-Enhanced Workflows: By automating routine tasks, AI frees agents to focus on complex issues, enhancing both efficiency and satisfaction. The AI continually learns and adapts with use, leading to smarter workflows and ongoing improvements.
- Advanced AI Capabilities: The platform’s AI not only predicts customer needs but also provides real-time support to agents, enhancing decision-making.
What to Look for in a Digital Chat Platform
Choosing the right digital chat platform can significantly impact your operational efficiency and customer satisfaction. Here are some key factors to consider:
- Driving Digital Adoption:
- Encourage Digital Shift: Prioritize a platform that promotes digital engagement over traditional voice channels, which are typically more expensive.
- Cost Efficiency: Digital interactions are far more cost-effective than voice calls.
- Reliability: Ensure the platform has a strong track record with minimal outages.
- Customer Behavior Insights: Research the factors that drive customers to choose digital channels over voice to tailor your strategy accordingly.
- User Experience for Agents and Customers:
- Customer-Focused Design: A platform with excellent user experience (UX) will make customers more aware of and inclined to use chat services.
- Agent Empowerment: Good UX for agents is essential to drive adoption and enhance agent productivity through automation.
- Service-Oriented: The technology should be built to serve both agents and customers, focusing on empowerment rather than control.
- AI-Native and Continuous Innovation:
- Harnessing AI: Choose a platform that is AI-native and continuously innovates, especially given the current surge in AI advancements.
- Custom Insights with GenAI: Platforms that leverage Generative AI can provide customized insights and maintain a competitive edge.
- Experimentation and Adaptability: Opt for providers who constantly experiment with both internal and leading external AI models, rather than relying on standard models driven by financial incentives.
Real Results with Altice
Melissa Price, VP of Customer Experience for Digital Self Serve at Altice, shared compelling results achieved through the implementation of ASAPP’s digital chat platform. She highlighted results, including:
- Increased Efficiency: Significant reduction in handling times and increased resolution rates.
- Enhanced Customer Satisfaction: Improved experiences leading to higher customer satisfaction scores.
- Value of AI-Native Solutions: The AI-native approach of ASAPP was crucial in achieving these outcomes, providing deep insights and automation that traditional platforms couldn’t match.
Switching Platforms
There are short term challenges of switching platforms. But, the long-term benefits—including enhanced operational efficiencies, better customer experiences, and staying ahead of technological advancements—far outweigh the initial transition hurdles.
How to make Migration as painless as possible?
To ensure a smooth migration to a new digital chat platform, start by focusing on thorough planning, selecting the right platform with excellent UX and reliability, and leveraging AI for continuous innovation. Engage stakeholders, provide comprehensive training, and implement in phases to minimize disruptions. For a more comprehensive guide, watch the on-demand recording.
Watch the Webinar On-Demand
Curious about how Altice and ASAPP are transforming customer engagement? Watch our on-demand webinar to gain valuable insights into their groundbreaking strategies and real-world successes. It’s a must-watch if your current digital chat platform isn't meeting your expectations.
Best-of-Breed vs. Omnichannel/CCaaS for the Digital Contact Center
Choosing the right CX software can be difficult. Learn how to think about choosing between a best-of-breed digital channel solution vs. an omnichannel solution.
Choosing the right CX software can be difficult. In this blog series, our goal is to give you helpful frameworks through which to think about how to purchase the right CX software to help your contact center improve customer experience, augment agent productivity, and reduce costs. (For a thorough breakdown of how to choose the right Transcription and Summarization Solutions, read our buyer’s guide here.)
This blog post covers how to think about acquiring the right Digital Customer Interaction Solution (DCIS) for your contact center. Should you choose a best-of-breed solution, specializing in digital channels, or an omnichannel/CCaaS solution, which offers both voice and digital channels?
Let’s dig in.
The Problem with the “All-in-One Solution”
Omnichannel Solutions, also commonly referred to as Contact Center-as-a-Service Solutions, offer an appealing promise: meet all your CX software needs across channels from just one vendor. The only problem? It’s rare to find an omnichannel solution that offers excellent technology for both voice and digital channels.
Most omnichannel solutions were built to optimize for voice channels and tacked on digital support only as an afterthought. As a result, omnichannel solutions tend to deliver subpar digital experiences, such as poor agent UX not optimized for chat workflows, limited bots, and low chat agent usage, frustrating both customers and agents.
It’s difficult to encourage customers to transition to digital support when you don’t have the technology to make their digital experience as useful and delightful as possible.
Of course, the lack of a comprehensive digital solution has significant consequences for your contact center’s operations.
Digital interactions are 1.5x to 4x cheaper than voice interactions. Contact centers understandably want to shift their mix to increase digital support. But without a digital chat platform that can reliably satisfy customers, it can be difficult to change customer behavior and preferences.
In sum, omnichannel solutions present themselves as an all-around answer, but in reality, their digital support options are severely lacking. What they offer in perceived convenience, they often trade in effectiveness.
Below, we break down how to think about best-of-breed vs. omnichannel solutions:
Interested in Learning More About Building a Digital-First Contact Center?
A digital-first contact center is essential to running a modern, efficient, and customer-friendly customer support operation.
Request a demo of ASAPP here to learn how ASAPP customers are:
- Automating Tier 1 interactions
- Experiencing happier, more productive agents
- Improving customer satisfaction
- Increasing revenue with contact center insights
- Drastically lowering operational costs
Conclusion
It’s natural to desire a single platform that can handle voice and digital contacts in one place. But there’s good reason to be skeptical of omni-channel platforms, too: by definition, omni-channel platforms lack specialization. It’s the classic jack of all trades, master of none problem.
Worse, because voice is often more profitable to omni-channel vendors than digital, companies that offer omnichannel platforms often invest the bulk of their development resources in voice over digital, allowing their digital technology to lag behind.
As more customers look to digital to interact with companies, it’s more important than ever that you get digital right. Consider choosing a vendor who specializes in digital channels rather than a broader omni-channel approach.
Who is ASAPP?
ASAPP is the AI-native® software for contact centers.
We help customer service leaders unlock their full value by minimizing costs & inefficiencies, improving agent compliance & productivity, and surfacing actionable insights while helping you deliver a great customer experience.
Our customers are large enterprises who care deeply about leveraging AI to transform CX by delivering unprecedented cost savings and maximizing customer delight.
What do we make?
We make a full suite of AI-native solutions designed specifically for the needs and nuances of the CX industry.
Why we are the right partner?
We strive to be the best technology partner you have ever had.
ASAPP is not new to the AI or the CX space. We have been building AI-native products for the contact center since 2014 and building our own LLMs since 2018. We invest heavily in our products and our workforce to bring our customers the best solutions on the market and the subject matter experts to ensure those customers are getting the maximum benefit.
We offer white-glove service and insight into contact centers’ best practices across industries, and our consultative nature drives transformative results. ASAPP is laser-focused on business outcomes, data usability, and helping you realize your desired customer experience.
If you are interested in how best-of-breed digital software for your contact center can benefit your organization, please click below to schedule a consultation:
Speak to an Expert
Omnichannel solutions present themselves as an all-around answer but in reality their digital support options are severely lacking. What they offer in convenience, they often trade in effectiveness.