Khash Kiani
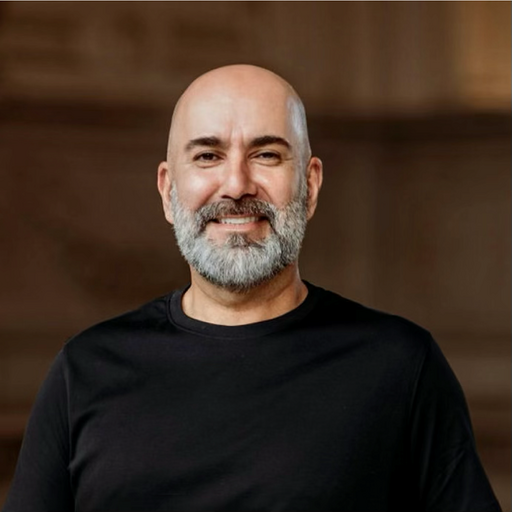
Khash Kiani is the Head of Security, Trust, and IT at ASAPP, where he ensures the security and integrity of the company's AI products and global infrastructure, emphasizing trust and safety for enterprise customers in regulated industries. Previously, Khash served as CISO at Berkshire Hathaway's Business Wire, overseeing global security and audit functions for B2B SaaS offerings that supported nearly 50% of Fortune 500 companies. He also held key roles as Global Head of Cybersecurity at Juul Labs and Executive Director and Head of Product Security at Micro Focus and HPE Software.
AI security and AI safety: Navigating the landscape for trustworthy generative AI
AI security & AI safety
In the rapidly evolving landscape of generative AI, the terms "security" and "safety" often crop up. While they might sound synonymous, they represent two distinct aspects of AI that demand attention for a comprehensive and trustworthy AI system. Let's dive into these concepts and explore how they shape the development and deployment of generative AI, using real-world examples from contact centers to make sense of these crucial elements. To start, here is a quick overview video on AI security and AI safety:
AI security: The shield against malicious threats
When we think about AI security, it's crucial to differentiate between novel AI-specific risks and security risks that are common across all types of applications, not just AI.
The reality is that over 90% of AI security efforts are dedicated to addressing critical basics and foundational security controls. These include data protection, encryption, data retention, PII redaction, authorization, and secure APIs. It’s important to understand that while novel AI-specific threats like prompt injection - where a malicious actor manipulates input to retrieve unauthorized data or inject system commands - do exist, they represent a smaller portion of the overall security landscape.
Let's consider a contact center chatbot powered by AI. A user might attempt to embed harmful scripts within their query, aiming to manipulate the AI into disclosing sensitive customer information, like social security numbers or credit card details. While this novel threat is significant, the primary defense lies in robust foundational security measures. These include input validation, strong data protection, employing encryption for sensitive information, and implementing strict authorization and data access controls.
Secure API access is another essential cornerstone. Ensuring that all API endpoints are authenticated and authorized prevents unauthorized access and data breaches. In addition to these basics, implementing multiple layers of defense helps mitigate novel threats. Input safety mechanisms can detect and block exploit attempts, preventing abuse like prompt leaks and code injections. Advanced Web Application Firewalls (WAFs) also play a vital role in defending against injection attacks, similar to defending against common application threats like SQL injection.
Continuous monitoring and logging of all interactions with the AI system is very important in detecting any suspicious activities. For example, an alert system can flag unusual API access patterns or data requests by an AI system, enabling rapid response to potential threats. Furthermore, a solid incident response plan is indispensable. It allows the security team to swiftly contain and mitigate the impact of any security events or breaches.
So while novel AI-specific risks do pose a threat, the lion’s share of AI security focuses on foundational security measures that are universal across all applications. By getting the basics right we build a robust shield around our AI systems, ensuring they remain resilient against both traditional and emerging threats.
AI safety: The guardrails for ethical and reliable AI
While AI security acts as a shield, AI safety functions like guardrails, ensuring the AI operates ethically and reliably. This involves measures to prevent unintended harm, ensure fairness, and adhere to ethical guidelines.
Imagine a scenario where an AI Agent in a contact center is tasked with prioritizing customer support tickets. Without proper safety measures, the AI could inadvertently favor tickets from specific types of customers, perhaps due to biased training data that inadvertently emphasizes certain demographics or issues. This could result in longer wait times and dissatisfaction for overlooked customers. To combat this, organizations should implement bias mitigation techniques, such as diverse training datasets. Regular audits and red teaming are essential to identify and rectify any inherent biases, promoting fair and just AI outputs. Establishing and adhering to ethical guidelines further ensures that the AI does not produce unfair or misleading prioritization decisions.
An important aspect of AI safety is addressing AI hallucinations, where the AI generates outputs that aren't grounded in reality or intended context. This can result in the AI fabricating information or providing incorrect responses. For instance, a customer service AI Agent might confidently present incorrect policy details if it isn't properly trained and grounded. Output safety layers and content filters play a crucial role here, monitoring outputs to catch and block any harmful or inappropriate content.
Implementing a human-in-the-loop process adds another layer of protection. Human operators can be called on to intervene when necessary, ensuring critical decisions are accurate and ethical. For example, contact center human agents can be the final step of authorization before performing a critical task, or providing additional insight when the AI system produces incorrect output or does not have enough information to support a user.
The intersection of security and safety
Though AI security and AI safety address different aspects of AI operation, they often overlap. A breach in AI security can lead to safety concerns if malicious actors manage to manipulate the AI's outputs. Conversely, inadequate safety measures can expose the system to security threats by allowing the AI to make incorrect or dangerous decisions.
Consider a scenario where a breach allows unauthorized access to the contact center’s AI system. The attackers could manipulate the AI to route calls improperly, causing delays and customer frustration. Conversely, if the AI's safety protocols are weak, it might inaccurately redirect emergency calls to non-critical queues, posing serious risks. Therefore, a balanced approach that addresses both security and safety is essential for developing a trustworthy generative AI solution.
Balanced approach for trustworthy AI
Understanding the distinction between AI security and AI safety is pivotal for building robust AI systems. Security measures protect the AI system from external threats, ensuring the integrity, confidentiality, and availability of data. Meanwhile, safety measures ensure that the AI operates ethically, producing accurate outputs.
By focusing on both security and safety, organizations can mitigate risks, enhance user trust, and responsibly unlock the full potential of generative AI. This dual focus ensures not only the operational integrity of AI systems but also their ethical and fair use, paving the road for a future where AI technologies are secure, reliable, and trustworthy.
Looking for an AI vendor you can trust? Not sure where to get started?
Watch our on-demand webinar to learn how