Ryan McDonald
.jpg)
Ryan McDonald, PHD, is Chief Scientist at ASAPP
Generative AI for Agent Augmentation: Agents Models not Language Models
Generative AI and Large Language Models (LLMs) have made massive waves in the consumer and enterprise technology news due to the remarkable capabilities of tools like ChatGPT and GPT4. The models provide fluent text that integrate reasoning into their responses based on the knowledge they have absorbed from the huge volume of general documents they are trained on. Not surprisingly, their use in chatbots has been one of the most active development areas for enterprise businesses.
While increasing containment of calls can save call centers dollars, the primary cost is still front line agents who must handle the most challenging calls and customers (otherwise a bot would have handled it). At ASAPP, we already have many tools that assist the agent when handling a live call. AutoCompose helps agents craft messages and significantly increases throughput in the call center while increasing CSAT in tandem. AutoSummary helps automate dispositioning steps for agents. Both use Generative AI models, in some cases for approximately five years.
However, agents spend their time doing much more than just writing messages to customers. They must execute actions on the customer’s behalf (e.g., change a seat on a flight or schedule a technician visit) as well as follow flows and instructions in knowledge base articles to be compliant when handling issues with safety or business regulations. To do this agents use a large number of tools. These tools are rarely homogeneous but are a frankenstack of vendors and user interfaces. On top of that, agents handling digital calls are usually managing more than one issue at a time, which leads to a huge number of applications open at once. Any model that focuses only on the text of a conversation and not all the actions the agent is executing is leaving a huge amount of headroom on the floor. For many of our customers agents can spend upwards of 60% of their time on tools outside of the conversation!!
Thus, to truly augment the agent a model must not just be a Language Model – it must be an Agent Model. That is, it needs to be a multimodal model that operates not just on the text of the conversation, but also on all the information the agent is currently interacting with as well as information hidden in business documents and logic that are salient for the issues at hand. At ASAPP, we have already invested in understanding the data stream of all agent actions and have used that data stream to build multimodal models that can improve augmentation for an agent. There is an amazing synergy when using this data. First, conditioning on the agent action data stream allows us to better improve our predictions of what the agent should say and do next. Conversely, information from the conversation feeds into what actions the agent should do, i.e., ‘I need to book a flight from New York to San Fran tomorrow in the morning’ allows the model to predict a flight search action, populate the origin city with ‘New York’, the destination to ‘San Francisco’ and the date as a day from today and execute that command.
Varying levels of experience with internal tools will impact how consistently advisors are solving customer problems. We commonly see less tenured representatives reaching out to their colleagues more often after getting stuck using an internal tool, spending more time searching for knowledge base articles, and switching back and forth more often between screens when handling workflows. Agent models can help newer agents become more comfortable and guide them to more effectively use their tools.
A core aspect of ASAPP’s mission is to ‘multiply agent productivity’. This can only be achieved in its fullest with Agent Models and not just Language Models.
Generative AI: When to Go Wide and When to Go Deep
Generative AI is everywhere, and you might be feeling the pressure from your colleagues or managers to explore how to incorporate Generative AI into your role or business. I’ve been seeing a lot of speculation about ChatGPT’s capabilities and what it can and cannot do. As a research scientist with years of experience in academic and industrial research with large language models, I wanted to dig into some of these notions:
First, ChatGPT is not a product, it’s an engine – and a really good one. However, a valuable solution still needs more in order to make a difference and drive business value in almost every case. This includes the UX (UI, latency, runtime constraints) and critical ML capabilities like data collection, data processing and selection, continuous training frameworks, optimizing models for outcomes (beyond next word prediction) and deployment (measurement, A/B tests, telemetry).
Second, while GPT does amazing things like write poetry, pass medical exams or write code (just to name a few), in CX we need solutions that solve specific problems like improving automated dispositioning or real-time agent augmentation. GPT models can be impressive, but when it comes to user experience and business outcomes, Vertical generative AI models that are trained on human data in a dynamic environment specifically for the task at hand typically outperform larger generic algorithms. In ASAPP’s case, this means solving customer experience pain points and building technology to make agents more productive.
Lastly, while we don’t use ChatGPT at ASAPP, we do train large language models and have deployed them for years. We don’t pre-train them on the web, but we do pre-train them on our customer data, which is quite sizable. From there, we then train them to solve specific tasks optimizing the model for specific KPIs and business outcomes we care about and need to solve for our customers — not just general AI. This includes purpose-built vertical AI technology for contact centers and CX. Vertical AI allows enterprises to transform by automating workflows, multiplying agent productivity and generating customer intelligence to provide optimal CX.
Interested in learning more about ChatGPT or how large language models might benefit your business? Drop us a line.
How task-oriented dialog helps empower agents
Why I joined ASAPP: Taking AI to new levels in enterprise solutions
I have spent the past 20 years working in natural language processing and machine learning. My first project involved automatically summarizing news for mobile phones. The system was sophisticated for its time, but it amounted to a number of brittle heuristics and rules. Fast forward two decades and techniques in natural language processing and machine learning have become so powerful that we use them every day—often without realizing it.
After finishing my studies, I spent the bulk of these 20 years at Google Research. I was amazed at how machine learning went from a promising tool to one that dominates almost every consumer service. At first, progress was slow. A classifier here or there in some peripheral system. Then, progress came faster, machine learning became a first class citizen. Finally, end-to-end learning started to replace whole ecosystems that a mere 10 years before were largely based on graphs, simple statistics and rules-based systems.
After working almost exclusively on consumer facing technologies. I started shifting my interests towards enterprise. There were so many interesting challenges that arose in this space. The complexity of needs, the heterogeneity of data and often the lack of clean, large-scale training sets that are critical to machine learning and natural language processing. However, there were properties that made enterprise tractable. While the complexity of tasks was high, the set of tasks any specific enterprise engaged in was finite and manageable. The users of enterprise technology are often domain experts and can be trained. Most importantly, these consumers of enterprise technology were excited to interact with artificial intelligence in new ways— if it could deliver on its promise to improve the quality and efficiency of their efforts.
This led me to ASAPP.
I am firm in my belief that to take enterprise AI to the next level a holistic approach is required. Companies must focus on challenges with systemic inefficiencies and develop solutions that combine domain expertise, machine learning, data science and user experience (UX) in order to elevate the work of practitioners. The goal is to improve and augment sub-tasks that computers can solve with high precision in order to enable experts to spend more time on more complex tasks. The core mission of ASAPPis exactly in line with this, specifically directed towards customer service, sales and support.
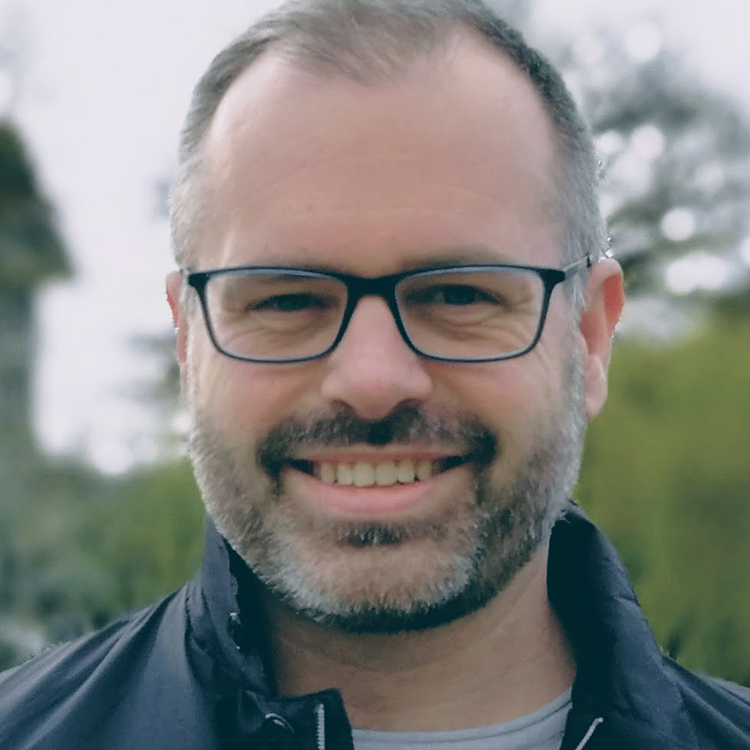
To take enterprise AI—and customer experience—to the next level a holistic approach is required.
Ryan McDonald, PhD
The customer experience is ripe for AI to elevate to the next level. Everyone has experienced bad customer service, but also amazing customer service. How do we understand choices that the best agents make? How do we recognize opportunities where AI can automate routine and monotonous tasks? Can AI help automate non deterministic tasks? How can AI improve the agent experience leading to less burn out, lower turnover and higher job satisfaction? This is in an industry that employs three million people in the United States alone but suffers from an average of 40 percent attrition—one of the highest rates of any industry.
ASAPP is focusing its efforts singularly on the Customer Experience and there are enough challenges here to last a lifetime. But, ASAPP also recognizes that this is the first major step on a longer journey. This is evident in the amazing research group that ASAPP has put together. They are not just AI in name, but also in practice. Our research group consists of machine learning and language technology leaders, many of whom publish multiple times a year. We also have some of the best advisors in the industry from universities like Cornell and MIT. This excites me about ASAPP. It is the perfect combination of challenges and commitment to advanced research that is needed in order to significantly move the needle in customer experience. I’m excited for our team and this journey.