Blog
Bringing State of the Art Speech Transcription to CX
Automatic Speech Recognition (ASR) has been a cornerstone capability for voice contact centers for years, enabling agents to review what was just said, or to review older calls to better gather context, and facilitating a whole suite of quality assurance and analytics capabilities. Because ASAPP specializes in serving large enterprise customers with a plethora of data, we’re always looking for ways to improve the scalability and performance of our speech-to-text models; even small wins in accuracy, for example, can translate into huge gains for our customers. Accordingly, we’ve recently made a strategic switch from a hybrid ASR architecture to a more powerful end-to-end neural model. Since adopting this new model we’ve been able to reduce the lower median latency of our model by over 50%, increase the accuracy, and lower the cost of running the model.
To understand why we made this strategic technological shift and how we achieved these results it helps to understand the status quo in real time transcription for contact centers. Often a hybrid model is used which combines separate complementary components. The first component is an acoustic model that translates the raw audio signal into phonemes, the basic units of human speech. Unfortunately the audio data alone can’t be used to construct a sentence of words, since phonemes can be combined in many different ways to construct words. To solve these ambiguities, a lexicon is used to map phonemes to possible words, and a third component, a language model, picks the most likely phrase or sentence from several candidates. This type of pipeline of separate components has been used for decades.
While hybrid architectures have been the standard, they have their limitations. First, because the acoustic model has been trained separately from the language model, they are not quite as powerful as a single larger model. In our new end-to-end architecture, the encoder gives a richer piece of data to the decoder than just phonemes; moreover the pieces of our architecture are all trained together, so they learn to work well together.
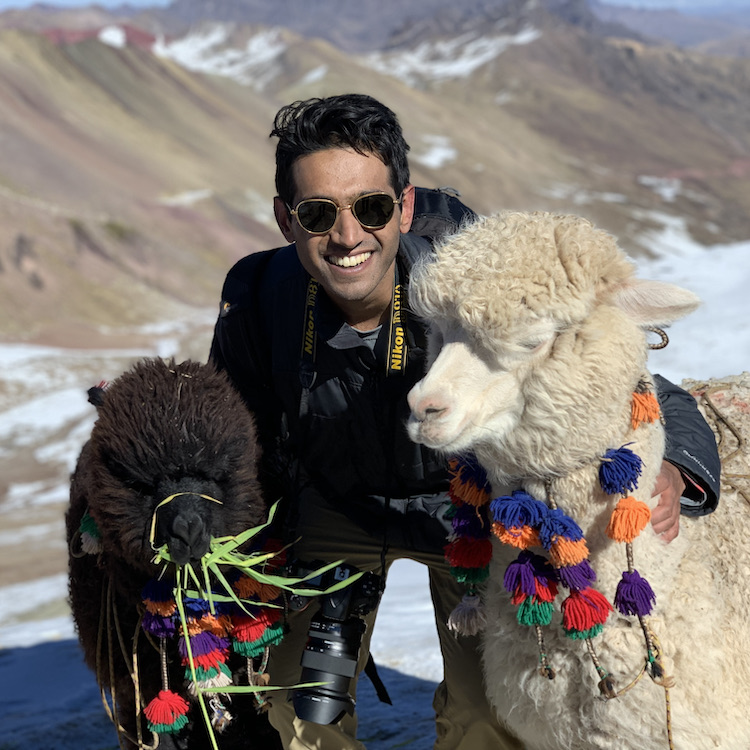
The nexus of GPU power, better modeling techniques, and bigger datasets enables better, faster models to serve our customers’ speech transcription needs
The separation of the model components in the legacy architecture has another constraint: it starts to get diminishing returns from more data. In contrast, our new integrated architecture requires more data, but also continues to improve more dramatically as we train it on new data. In other words, this new model is better able to take advantage of the large amounts of data that we encounter working with enterprise customers. Some of this data is text without audio or vice versa and leveraging it allows us to further boost model performance without expensive transcription annotation by humans. It’s worth noting the power of modern GPUs has catalyzed the success of these new techniques, enabling these larger jointly trained models to train on larger datasets in reasonable amounts of time.
Once trained, we can tally up all the metrics and see improvements across the board: The training process is simpler and easier to scale, it’s twice as cheap, and twice as fast*. The model also balances real time demand with historical accuracy: the model waits a few milliseconds to consider audio slightly into the future, giving it more context to predict the right words in virtually real time; finally the model contains a rescoring component that utilizes a larger window of audio to commit an even more accurate transcription to the historical record. Both our real time and historical transcription capabilities are advancing the state of the art.
ASAPP E2E Performance By the Numbers
This was not an easy task. ASAPP has a world class team that continuously looks for ways to improve our speech capabilities. The nexus of GPU power, better modeling techniques, and bigger datasets reduces the need for an external language model and enables them to train the whole thing end to end. These improvements translate into better, faster models that our customers can leverage for their speech transcription needs.
e ASAPP next generation E2E speech recognition system, and on scaling speech out to all of our customers.
Are you missing key revenue growth opportunities?
Is your customer experience always getting smarter?
Pressure to deliver better customer experiences at lower cost is a real challenge. Balancing these two competing priorities is driving innovation, with the real paradigm shift coming from innovations in machine learning and AI.
Automation already plays a role in reducing costs, but when the sole focus is on efficiency, the customer experience often suffers. You risk frustrating customers who express their dissatisfaction in social media and worse, leaving your brand. I think the future of customer experience is using AI to create a self-learning, digital-first business—one that gets smarter all the time—to address many challenging factors at once.
Machine learning is key. It deepens your understanding about customers to better identify where automation works best, and how to personalize interactions across channels. And it empowers agents with predictive knowledge to make them significantly more efficient and productive.
Automate away the routine, not the CX
Ideally, the goal of automation is to accelerate service delivery and resolution for customers, in ways that improve customer experience and lower costs. However, automation should not be exclusively about eliminating human involvement. Satisfying customers without live intervention needs to be part of it; but you also want labor-saving technology that makes live agents more efficient and effective.
Chatbots are great for automating simple tasks. But it would take an army of people to imagine and program all the possible scenarios to fully replicate the experience of speaking with a live agent. That’s why I think automation is most empowering with a system that continuously learns from agents the right thing to say and do in every situation. You can then apply those learnings to automate more and more interactions into predictive suggestions. And over time, those suggestions become actions that the system can automatically handle on behalf of an agent, leaving the agent to more complicated tasks that require a human touch.
In other words, automation becomes the brain of the process, not the process itself. Yes, it powers automated self-service. AND with predictive knowledge, it shortens the time it takes for agents to address issues, which means they can serve customers better, faster, and easier.
Grow smarter agents, smarter channels
Even if you automate away common tasks, many situations still need the human touch. You want those experiences to keep getting smarter as well. Empowering agents with machine learning predictive knowledge ensures they can handle any situation as effectively as your best agent. Real-time conversational analytics and machine learning fuel proactive suggestions that make agents more efficient at handling complex conversations, so every agent can address specialized topics and scenarios.
Intelligent, labor-saving technology also helps solve the common customer complaint about fragmented experiences. People get frustrated when they can’t get help through their preferred digital channels, and even more annoyed if they need to switch channels mid-conversation and have to start all over again.
An integrated, self-learning platform enables seamless continuity across all service channels. Digital messaging, in particular, allows customers to pause a conversation (and even jump from chat to texting or social media to chat), while keeping a continuous thread going until they get everything they need. A smart system ensures they don’t have to start over, saving time and effort for both customers and agents.
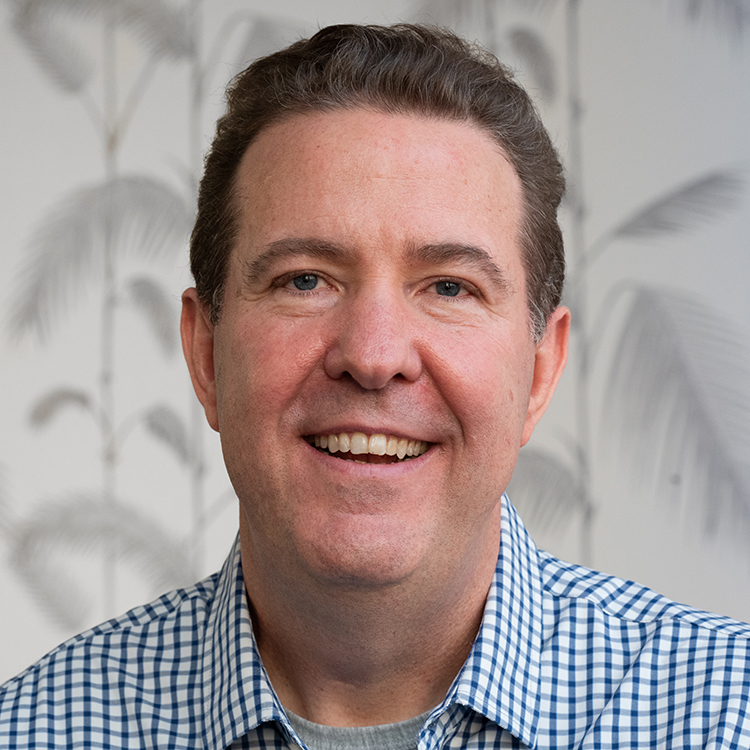
At every company I’ve ever worked, any time we delivered a great experience to a customer, their lifetime value went up. Delivering smarter, faster, more personal customer service is at the heart of every great customer experience.
Michael Lawder
Increase value with continuous learning
Enabling a customer service organization to continuously get smarter is one of the things I love most about AI. Over time, you keep learning new ways to automate for efficiency, new ways to help agents work more productively — and also new ways to extract value from a wealth of data.
An AI-driven system enables you to harness volumes of data from every conversation across every channel. It analyzes real-time voice transcription and text data from multi-channel digital messaging for increasingly valuable insights you can put to use. It also factors in voice of the customer data from across the enterprise for more informed decision-making.
Intelligent conversational analytics give you a competitive edge. You can better know your customers to provide more personalized support. You can equip agents to resolve issues faster. And you can ensure the knowledge of your best agents is available for everyone to use.
It’s the ultimate digital-first strategy, enabling companies to optimize customer service and CX in very focused ways that increase satisfaction and drive loyalty.
But wait, there’s more. Conversational insights also deliver value well beyond the contact center. Sales and marketing can gain substantially deeper understanding of customer concerns, buying patterns, and decision drivers. This enables the business to deliver more relevant and personalized predictive offers to increase revenue and marketing ROI.
Go big with transformative results
I’ve been in customer experience for over two decades, starting as a call center agent long ago, and only now am I seeing AI really deliver transformative results. ASAPP enables businesses to continuously get smarter, reinventing customer service in ways that translate into retention and brand loyalty to improve the bottom line.
Generic transcription models yield generic results
What’s so different about the ASAPP approach to customer experience?
Reducing the high cost of training NLP models with SRU++
Natural language models have achieved various groundbreaking results in NLP and related fields [1, 2, 3, 4]. At the same time, the size of these models have increased enormously, growing to millions (or even billions) of parameters, along with a significant increase in the financial cost.
The cost associated with training large models limits the research communities ability to innovate, because a research project often needs a lot of experimentation. Consider training a top-performing language model [5] on the Billion Word benchmark. A single experiment would take 384 GPU days (6 days * 64 V100 GPUs, or as much as $36,000 using AWS on-demand instances). That high cost of building such models hinders their use in real-world business, and makes monetization of AI & NLP technologies more difficult.

Our model obtains better perplexity and bits-per-character (bpc) while using 2.5x-10x less training time and cost compared to top-performing Transformer models. Our results reaffirm the empirical observations that attention is not all we need.
- Tao Lei, Research Leader and Scientist, ASAPP
The increasing computation time and cost highlight the importance of inventing computationally efficient models that retain top modeling power with reduced or accelerated computation.
The Transformer architecture was proposed to accelerate model training in NLP. Specifically, it is built entirely upon self-attention and avoids the use of recurrence. The rationale of this design choice, as mentioned in the original work, is to enable strong parallelization (by utilizing the full power of GPUs and TPUs). In addition, the attention mechanism is an extremely powerful component that permits efficient modeling of variable-length inputs. These advantages have made Transformer an expressive and efficient unit, and as a result, the predominant architecture for NLP.
A couple of interesting questions arises following the development of Transformer:
- Is attention all we need for modeling?
- If recurrence is not a compute bottleneck, can we find better architectures?
SRU++ and related work
We present SRU++ as a possible answer to the above question. The inspiration of SRU++ comes from two lines of research:
First, previous works have tackled the parallelization/speed problem of RNNs and proposed various fast recurrent networks [7, 8, 9, 10]. Examples include Quasi-RNN and Simple Recurrent Unit (SRU), both are highly-parallelizable RNNs. The advance eliminates the need of eschewing recurrences to trade training efficiency.
Second, several recent works have achieved strong results by leveraging recurrence in conjunction with self-attention. For example, Merity (2019) demonstrated a single-headed attention LSTM (SHA-LSTM) is sufficient to achieve competitive results on character-level language modeling task while requiring significantly less training time. In addition, RNNs have been incorporated into Transformer architectures, resulting in better results on machine translation and natural language understanding tasks [8, 12]. These results suggest that recurrence and attention are complementary at sequence modeling.
In light of the previous research, we enhance the modeling capacity of SRU by incorporating self-attention as part of the architecture. A simple illustration of the resulting architecture SRU++ is shown in Figure 1c.
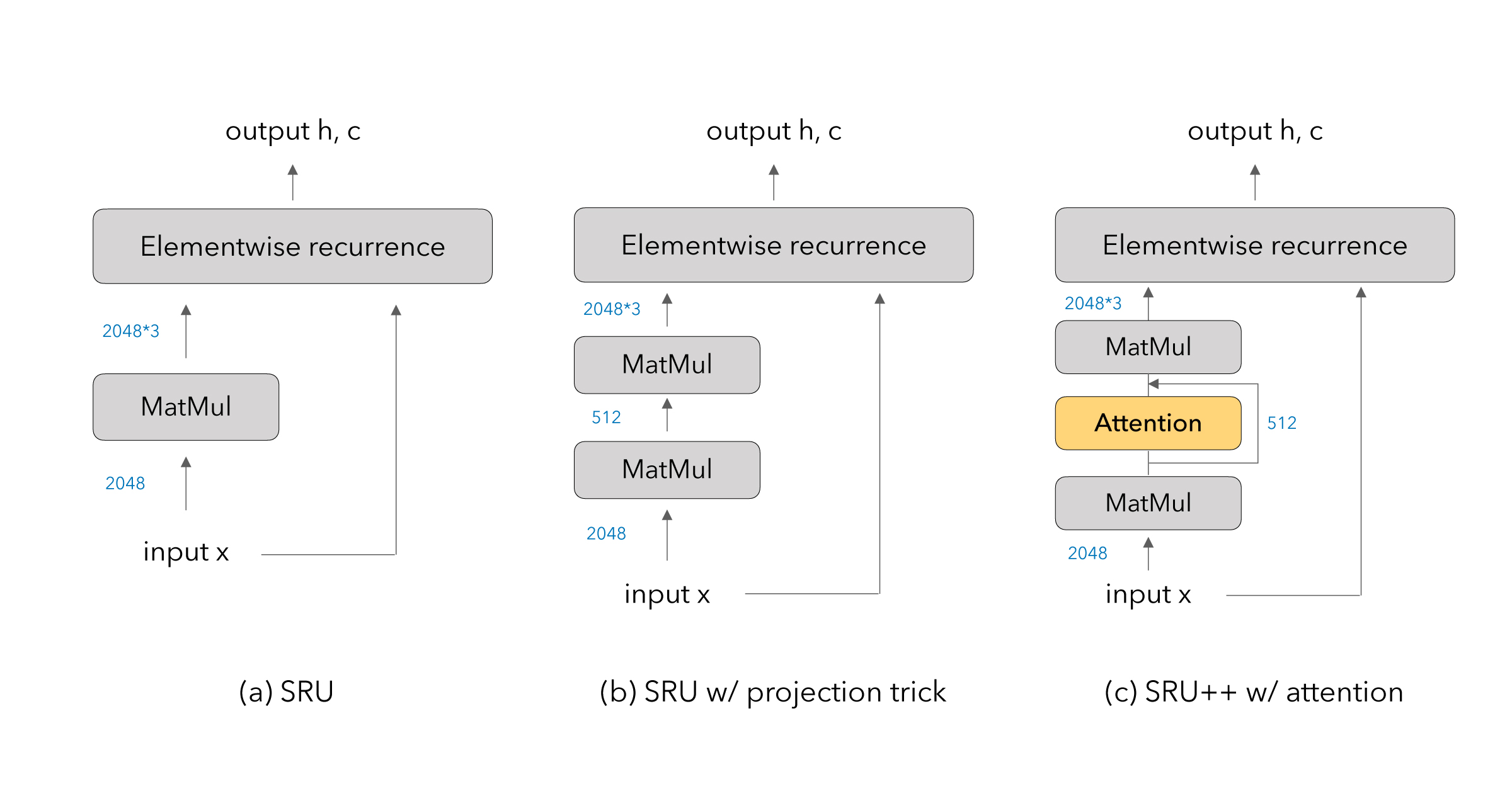
SRU++ replaces the linear mapping of the input (Figure 1a) by first projecting the input into a smaller dimension. An attention operation is then applied, followed by a residual connection. The dimension is projected back to the hidden size needed by the elementwise recurrence operation of SRU. In addition, not every SRU++ layer needs attention. When the attention is disabled in SRU++, the network reduces to a SRU variant using dimension reduction to reduce the number of parameters (Figure 1b).
Results
1. SRU++ is a highly-efficient neural architecture
We evaluate SRU++ on several language modeling benchmarks such as Enwik8 dataset. Compared to Transformer models such as Transformer-XL, SRU++ can achieve similar results using only a fraction of the resources. Figure 2 compares the training efficiency between the two with directly comparable training settings. SRU++ is 8.7x more efficient to surpass the dev result of Transformer-XL, and 5.1x more efficient to reach a BPC (bits-per-character) of 1.17.
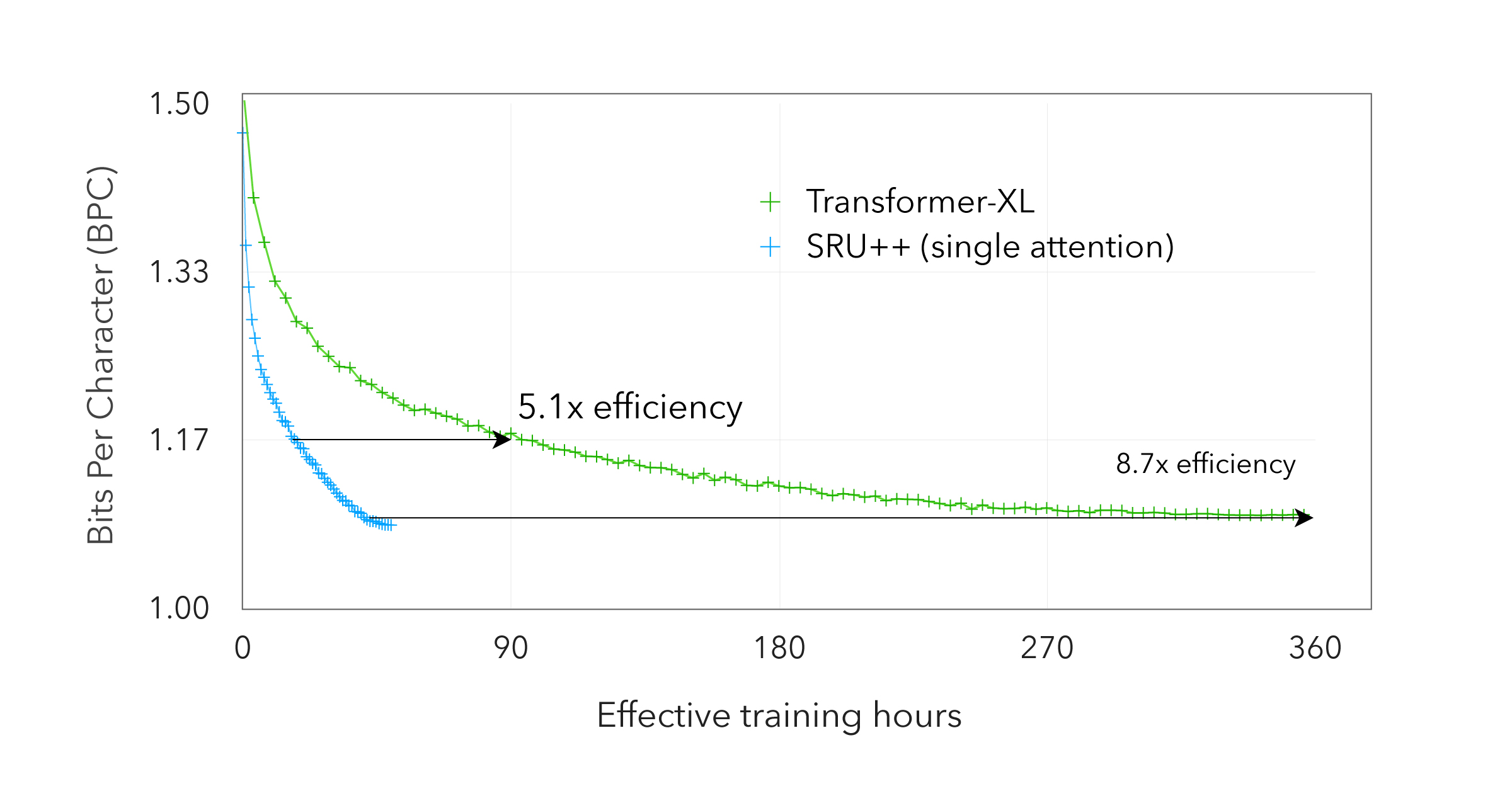

Table 1 further compares the training cost of SRU++ and reported costs of leading Transformer-based models on Enwik8 and Wiki-103 datasets. Our model can achieve over 10x cost reduction while still outperforming the baseline models on test perplexity or BPC.
2. Little attention is needed given recurrence
Similar to the observation of Merity (2019), we found using a couple of attention layers sufficient to obtain state-of-the-art results. Table 2 shows an analysis by only enabling the attention computation every k layers of SRU++.

Conclusion
We present a recurrent architecture with optional built-in self-attention that achieves leading model capacity and training efficiency. We demonstrate that highly expressive and efficient models can be derived using a combination of attention and fast recurrence. Our results reaffirm the empirical observations that attention is not all we need, and can be complemented by other sequential modeling modules.
For further reading, ASAPP also conducts research to reduce the cost of model inference. See our published work on model distillation and pruning for example.
What keeps your agents from providing great service?
A very different perspective on customer experience
Why companies who want true VoC need to engage the power of AI
The best businesses succeed by developing a holistic understanding of their customers. Most, if not all, consumer companies have a Voice of the Customer (VoC) program, intended to capture and analyze feedback, leveraging the insights to drive both strategic and operational improvements across the business. While the intent of these programs is critical to constant improvement, the tools that have been available to CX professionals fall short of delivering what they really need.
Surveys and samples only give a partial view
Many organizations build VoC programs solely on a “survey and score” foundation. When done right, surveys can play an important role in any VoC program. But due to their low average response rate and general bias, they provide organizations with a limited view of the overall customer experience and the quality of service that is being delivered by your organization.
An overreliance on surveys has other pitfalls, too. Relationship-based surveys, for example, evaluate general brand satisfaction, but often fail to provide clear feedback on the internal processes, people, and frontline events that contribute to customer experience. On the flip side, transaction-based surveys capture feedback in the moment, but tend to lose sight of what the overall relationship looks like from the customer’s point of view.
Other companies might record calls, then either listen to or transcribe a subset of these calls. This approach also limits analysis to a small sample of customer interactions.
Analyzing only a fraction of your calls fails to tell the whole story. Yet companies rely on this data to make important decisions about product, sales, and marketing initiatives as well as contact center operations.
What’s more, with both of these approaches, there can be a significant time lapse between capturing the data, gaining insight from that data, and putting that insight into action. The truth is that most of us in the customer experience world have never had a full view of the quality of service we are delivering to our customers, and the opportunities that exist to improve the way in which we serve our customers across the enterprise.
AI elevates VoC with new possibilities
Artificial intelligence fuels new options for gaining more comprehensive customer insight. And, for putting that insight into action. Forward thinking CX leaders are excited about mining this wealth of data and are heartened to learn that they won’t need an army of data scientists on staff to do it.
Highly accurate transcription is key
The best of these new solutions start with highly accurate real-time transcription of every call. Transcription is not the goal, but a means to an end. However the importance of the quality of transcription can’t be overstated, as this is the fuel for meaningful analysis. More on this here.

AI solutions that use machine learning models custom trained on a company’s lexicon are—not surprisingly—far more accurate than solutions using generic models trained on everyone’s data. Consequently, they can deliver far more value.
Michael Lawder
Getting this data in real time gives companies the opportunity to take action instantly instead of waiting weeks, months, or even longer to address customer needs. And having it for every call gives companies a much fuller customer perspective.
Rich actionable insights
The real value comes not in just getting the data, but in being able to put it to use in meaningful ways. Beyond accurately transcribing customer conversations, an AI-driven VoC program can:
- Analyze sentiment and even predict CSAT and NPS scores
- Capture customers problem statements
- Classify intent at a useful level of detail
- Spot correlations between things—for example: callbacks or sentiment by agent, intent, or length of call
- Highlight trends and anomalies in customer conversations
- Alert supervisors of coaching need by agent or topic
- Automate summary notes, providing cleaner data for analysis and better records for future customer contact
For the first time, you can effectively measure the quality of service you are delivering for every product, every interaction, every agent.
Cultivating VoC of this depth can do more than help manage and optimize CX operations. It has the power to influence business as a whole. CX leaders become the ultimate advocate for the customer, able to synthesize customer wants and needs as they relate to every stage of the customer journey. This elevates their stature in the organization, as they become trusted sources for insights that inform key decisions and strategy aimed to build customer loyalty and grow revenue. If you’d like to hear how companies in your industry are using AI-driven speech intelligence solutions in their VOC programs, drop us a line at ask@asapp.com.