Blog
To measure the performance of Conversational AI, we need more strict, better quality benchmarks
Introducing the Spoken Language Understanding Evaluation (SLUE) benchmark suite
Progress on speech processing has benefited from shared datasets and benchmarks. Historically, these have focused on automatic speech recognition (ASR), speaker identification, or other lower-level tasks. However, “higher-level” spoken language understanding (SLU) tasks have received less attention and resources in the speech community. There are numerous tasks at varying linguistic levels that have been benchmarked extensively for text input by the natural language processing (NLP) community – named entity recognition, parsing, sentiment analysis, entailment, summarization, and so on – but they have not been as thoroughly addressed for speech input.
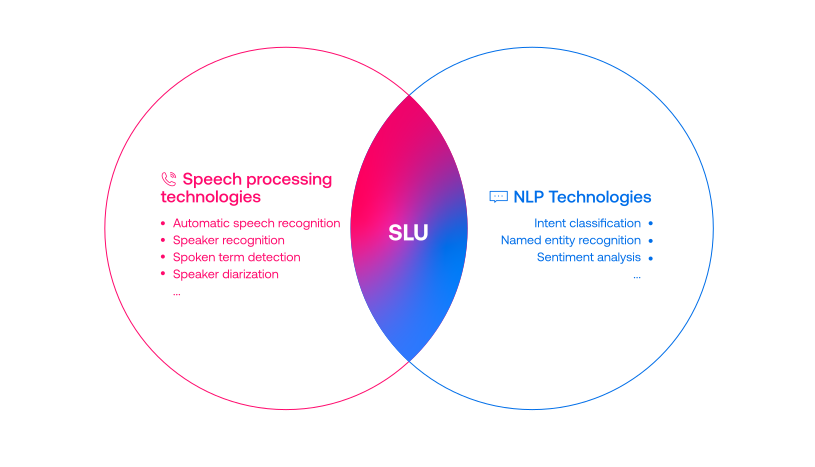
Consequently, SLU is at the intersection of speech and NLP fields but was not addressed seriously from either side. We think that the biggest reason for this disconnect is due to a lack of an appropriate benchmark dataset. This lack makes performance comparisons very difficult and raises the barriers of entry into this field. A high quality benchmark would allow both the speech and NLP community to address open research questions about SLU—such as which tasks can be addressed well by pipeline ASR+NLP approaches, and which applications benefit from having end to end or joint modeling. And, for the latter kind of tasks, how to best extract the needed speech information.

For conversational AI to advance, the broader scientific community must be able to work together and explore with easily accessible state-of-the-art baselines for fair performance comparisons.
We believe that for conversational AI to advance, the broader scientific community must be able to work together and explore with easily accessible state-of-the-art baselines for fair performance comparisons. A present lack of benchmarks of this kind is our main motivation in establishing the SLUE benchmark and its suite.
The first phase of SLUE
We are launching the first benchmark which considers ASR, NER, and SLU with a particular emphasis on low-resource SLU. For this benchmark, we contribute the following:
- New annotation of publicly available, natural speech data for training and evaluation on new tasks, specifically named entity recognition (NER) and sentiment analysis (SA), as well as new text transcriptions for training and evaluating ASR systems on the same data.
- A benchmark suite including a toolkit for reproducing state-of-the-art baseline models and evaluation, the annotated data, website, and leaderboard.
- A variety of baseline models that can be reproduced to measure the state of existing models on these new tasks.
- A small size labeled dataset to address a new algorithm and findings for low-resource SLU tasks
SLUE covers 2 SLU tasks (NER and SA) + ASR tasks. All evaluation in this benchmark starts with the speech as input whether it is a pipeline approach (ASR+NLP model) or end-to-end model that predicts results directly from speech.
The provided SLUE benchmark suite covers for downloading dataset, training state-of-the-art baselines and evaluation with high-quality annotation. In the website, we provide the online leaderboard to follow the up-to-date performance and we strongly believe that the SLUE benchmark makes SLU tasks much more easily accessible and researchers can focus on problem-solving.

Current leaderboard of the SLUE benchmark
Why it matters
Recent SLU-related benchmarks have been proposed with similar motivations to SLUE. However, those benchmarks cannot perform as comprehensively as SLUE due to the following reasons:
- Some of their tasks already achieve nearly perfect performance (SUPERB, ATIS), not enough to discriminate between different approaches.
- Other benchmark datasets consist of artificial (synthesized) rather than natural speech (SLURP), which don’t recreate real-world conditions
- There is no training audio available while only providing audio for evaluation (ASR-GLUE)
- Other benchmark datasets use short speech commands rather than longer conversational speech (SLURP, FSC)
- Have license constraints limiting their industry use (Switchboard NXT, FSC)
SLUE provides a comprehensive comparison between models without those shortcomings. An expected contribution to the SLUE benchmark would
- Track research progress on multiple SLU tasks,
- Facilitate the development of pre-trained representations by providing fine-tuning and eval sets for a variety of SLU tasks,
- Foster the open exchange of research by focusing on freely available datasets that all academic and industrial groups can easily use.
Motivated by the growing interest in SLU tasks and recent progress on pre-trained representations, we have proposed a new benchmark suite consisting of newly annotated fine-tuning and evaluation sets, and have provided annotations and baselines for new NER, sentiment, and ASR evaluations. For the initial study of the SLUE benchmark, we evaluated numerous baseline systems using current state-of-the-art speech and NLP models.
This work is open to all researchers in the multidisciplinary community. We welcome similar research efforts focused on low-resource SLU, so we can continue to expand this benchmark suite with more tests and data. To contribute or expand on our open-source dataset, please email or get in touch with us at sshon@asapp.com.
Additional Resources
- Attend our ICASSP 2022 session
- SPE-67.1: SLUE: NEW BENCHMARK TASKS FOR SPOKEN LANGUAGE UNDERSTANDING EVALUATION ON NATURAL SPEECH
- Presentation Time: Thu, 12 May, 08:00 – 08:45 New York Time (UTC -4)
- Attend our Interspeech 2022 special session “low-resource SLU”
- September 18-22, 2022, Incheon, South Korea
- Paper
- SLUE Benchmark Suite (Toolkit and dataset)
- Website and leaderboard
- Email me (sshon@asapp.com) or get in touch with us @ASAPP.
A contact center case study about call summarization strategies
All agents at a contact center are typically required to write summary – or disposition – notes for each conversation. These notes are intended to be used for several purposes. They provide context if the issue needs to be revisited in follow up calls. This avoids the need for the customer to repeat the problem and saves the agent time. Also, supervisors can use these notes to see how often certain situations arise and identify coaching opportunities. Good disposition notes will include the customer’s contact reason, key actions taken to solve it, and the conversation outcome.
The time required to take these notes is, on average, 10% of the actual call duration and agents may only capture some aspects of the conversation. This is why many contact center leaders are looking for ways to reduce the time spent writing these notes and increase their quality.
Findings from a large enterprise contact center
Like most contact centers, agents in this company were writing all notes at the end of each conversation. Aiming to increase agent’s utilization (i.e. the proportion of time agents are talking with a customer) they shifted to having their agents write the notes during, and not after, the conversation. They encourage them to write these notes in “natural pauses” inside the conversation. This way, agents reduce significantly the time between when a conversation ends and the next conversation starts.
In reviewing call data, we learned that in a big proportion of the voice calls, these natural pauses do not occur very often. To understand this, for each conversation, we first identify the time intervals in which the customer or the agent are talking. This can be observed in Figure 1 below. Based on these customer and agent turn intervals, we can identify the pauses in the conversation. For this analysis, we only keep the pauses which have a duration of at least 10 seconds.

As we show in the histogram from Figure 2, we estimate that half of the calls have less than one pause every two minutes and 13% of the calls have no pauses at all. Moreover, for most of those pauses, agents are busy actively working on the issue (looking for information, filling forms, etc.), so taking notes is not a possibility.

This means that when an agent takes notes in the middle of the conversation, they are usually creating an artificial pause. In other words, they are transferring the time it would have taken to take the notes at the end, to more time spent on the call with each customer. Moreover, when they don’t finish notes during the call, note-taking for that call spills over into the next call, which significantly increases the complexity for the agent.
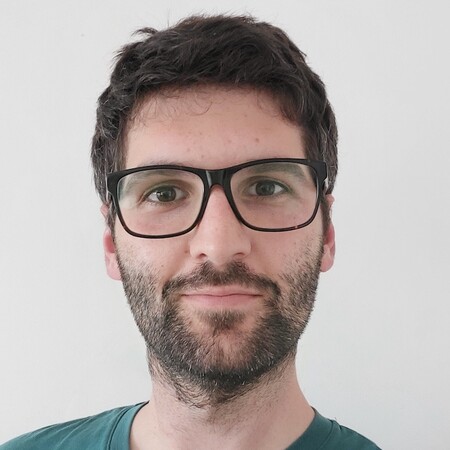
Having agents take notes during the conversation does not improve efficiency and may harm the overall customer experience.
On the customer side, pauses in the middle of the conversation likely have negative consequences. Our data consistently shows that conversations with longer response times are associated with a lower Customer Satisfaction (CSAT) score, as we show in Figure 3 (the CSAT is on a scale from 1 to 5 here). In addition to waiting through pauses, the overall time the customer (and the agent) spend on the call is longer.

The value of automating call summaries
We already showed that taking notes during the call does not improve agent efficiency and may harm the overall customer experience. On the other hand, automating conversation summaries can be a way to reduce or completely eliminate dispositioning time for the agents as well as increase the general quality of the summaries.
The customer in this case study is initially making the automated summaries visible in their agent desk, enabling the agents to review and edit.
This has significantly reduced the time agents devote to this task.
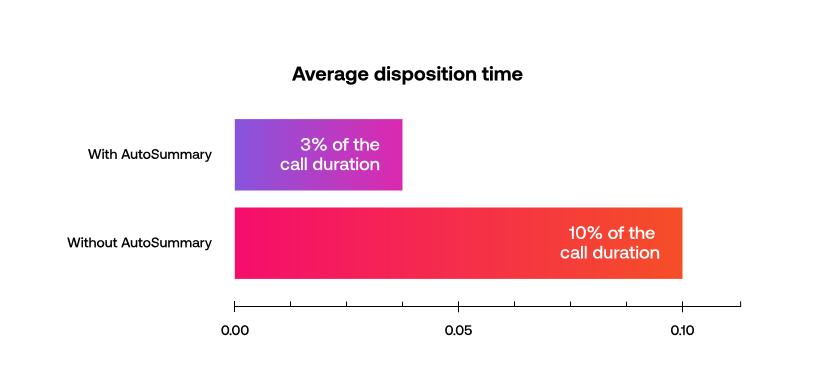
As confidence in the AutoSummary model grows, companies may opt to remove manual reviews completely from agents’ task list—and take the additional efficiency gains available. Other customers bypass this step and use AutoSummary without any agent engagement from the start.
Why your care strategy must consider issue complexity and urgency
A common trait of people working in technology is a desire to be able to cleanly categorize information, data, issues, etc. To be able to delineate between one bucket and another. We see this manifest in how companies think about customer conversations—should a conversation be automated? Yes or no? Does the customer need a live engagement for the entirety of the conversation vs. more asynchronous? Yes or no? But customer conversations aren’t actually so clearcut, and the needs don’t stay consistent as conversations and customer journeys go on.
At ASAPP, we have developed a fairly unique way of thinking about conversations. Rather than relying on a single intent to determine how the entire conversation should be handled, let’s look at each turn of the conversation to better inform what the next step should be. Every request has different needs, which change considerably based on various factors.
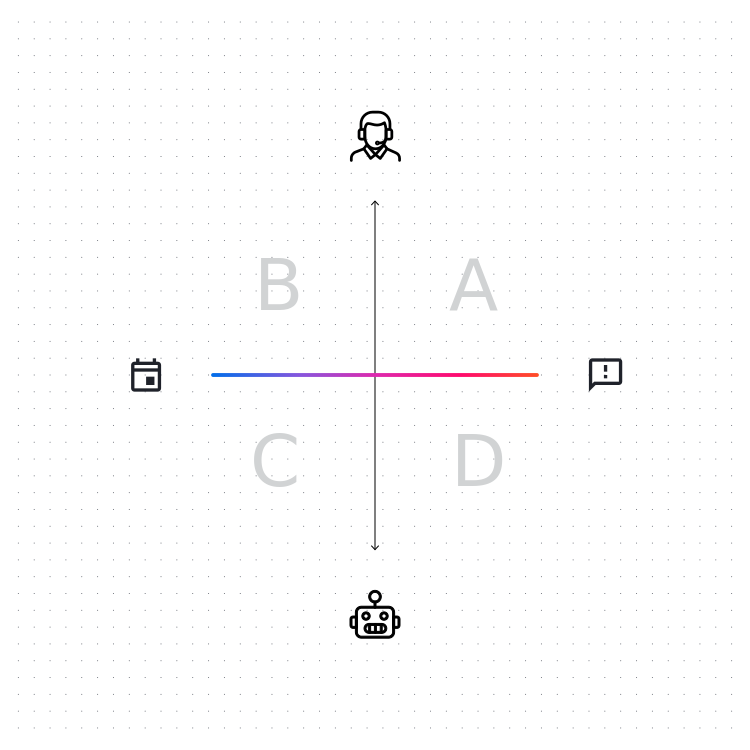
The above graph provides a nice illustration of how we can think about the issues. Along the y-axis, you have more complex vs. more simple interactions. At the bottom, you have conversations that are well served to be fully automated without any agent intervention. On the top, you have the opposite—conversations that benefit from having a skilled agent along for the ride. But those are extremes, most conversations fall in between the two, they require some human involvement and a bunch of automation. By thinking in very binary terms, automated or not automated, you lose out on all of the opportunities to reduce agent workload on a conversation by 20%, by 50%, by 75%. By treating each piece of a conversation as worthy of its own classification and diagnosis, you bring a lot of efficiency back into your business without risking frustrating your customer.
Now the x-axis, here we’re thinking about how routine vs. how urgent the issue is. It’s easy to think “we can serve customers asynchronously, they send an SMS. We get back to them when we get back to them, just like customers are used to interacting with friends and family.” But that leaves out a very important part of the picture. While many conversations are routine and can benefit from more asynchronous interactions, allowing companies to load balance workload on agents, there are cases where customers need urgent help—make a change to a flight about to take off, help resolve billing issue just before superbowl kick off, and in those cases, you don’t want to risk a customer not getting a response in time, especially not when so many conversions didn’t need that live resolution. Then there are cases just as with complexity vs. simplicity that are in between—an initial response might need help from a live agent, cutting off access to a bank account in the case of fraud, but the follow-ups and resolutions are well-served for asynchronous communication.
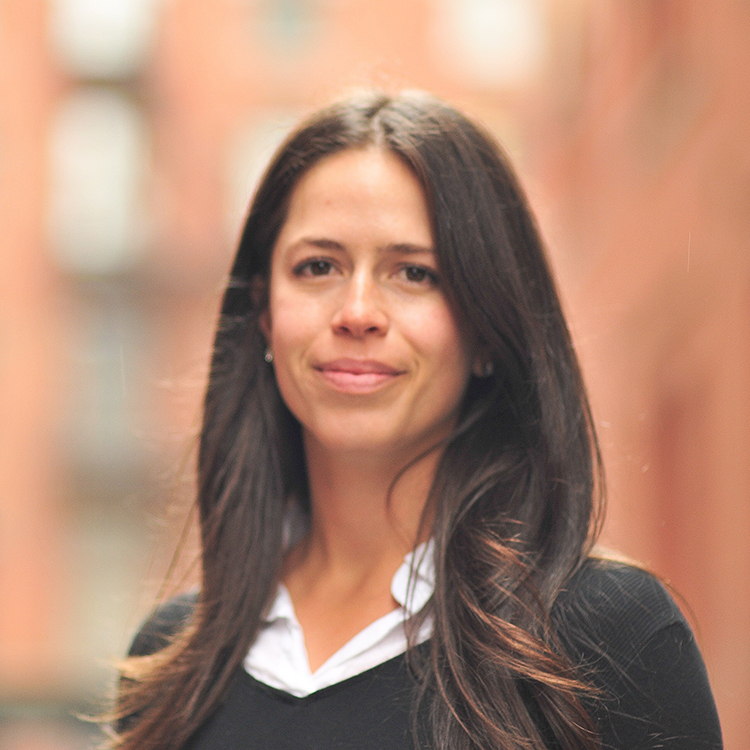
Customer interactions require different levels of attention. From simple routine issues to urgent complex requests, organizations must be able to seamlessly support every type of need, in the most efficient way possible, using the right mix of agent and automation.
Rachel Knaster
In addition to the content of what the customer is asking about, it’s important to take in every parameter you know about them and the context surrounding their issue. This goes far beyond simple intent classification. In order to determine the type of service customers need, you need to look at the entire weight of their requests. The best way to think about it is along axes of complexity and urgency.
Based on where they fall on this graph, customer interactions require different levels of attention. From simple routine issues (C) to urgent complex requests (A), organizations must be able to seamlessly support every type of need, in the most efficient way possible, using the right mix of agent and automation.
Is the customer’s question simple to solve? Then let’s automate it.
Is it complicated? Then let’s connect them with our frontline and have those agents do what they do best.
Is the issue one that can wait for an answer and more asynchronous by nature? Then let’s treat it that way.
Or is a customer’s flight about to take off and they need help? Let’s immediately connect them with someone.
These are fundamental questions contact centers should consider with every incoming request. There’s “no one size fits all” when it comes to CX strategy. Every interaction requires a different approach. so you can maximize throughput while keeping each customer satisfied.
Consider the graph above. Each quadrant represents a different category of request with its own unique considerations. In each case, the right mixture of live agent and AI, synchronous and asynchronous support can help solve the issue in the most optimal way possible. Here’s the ideal for each:
- Complex, urgent
- Agent-based, synchronous
- Low agent concurrency
- Automate part of agent workload
- Opportunity to mix voice and digital in same live conversation for faster resolution
- Complex, routine
- Agent-based, asynchronous
- Automate part of agent workload
- High agent concurrency
- Handoff to phone if required
- Simple, routine
- Fully automated interaction
- Low cost to serve
- Simple, urgent
- Fully automated, with fast escalation to live agent
- Complete history (context) of interaction required for agent
- Medium-high agent concurrency
- Automate part of agent workload
- Opportunity to mix voice and digital in same live conversation for faster resolution
While companies might prefer everything be automated or self service, that’s not always the most efficient way to solve an issue. Of course, neither is having your agents occupied addressing routine tasks all day. What’s needed is the right balance between the two—AI enhancing human performance so agents can handle more tasks and fully concentrate on those that need it. This is where more sophisticated machine learning offers incredible value.
There is an opportunity for AI to assist in every interaction, whether it’s handling the entire request or just part of the workload. While typically considered most helpful for automating simple tasks, the right AI models will improve over time, learning from customer interactions to assist with increasingly complex issues.
A single conversation can also become more simple or complex as it evolves, calling for changing levels of agent attention. For instance, now that the primary issue has been resolved, can the rest of this interaction be automated? Or has the issue escalated from automation to the need for an agent? Instant intent analysis provided by machine learning can help identify these occurrences to further optimize agent concurrency.
The truth is, sometimes the best thing is to have an agent live with just one customer, and sometimes it’s to have them handling multiple conversations. What’s important is for each organization to recognize the nuance and to build flexible solutions that adapt for the best outcomes to ensure operational performance is being enhanced, while never compromising on a personalized and connected experience for customers.
How to Understand Different Levels of AI Systems
AI systems have additional considerations over traditional software. A key difference is in the maintenance cost. Most of the cost of an AI system happens after the code has been deployed. ML models degrade over time without ongoing investment in data and hyperparameter tuning.
The cost structure of AI systems are directly affected by these design decisions; the level of service, and improvement over time are categorically different across different levels. Knowing the level of the AI system can help practitioners and customers predict how the system will change over time – whether it will continuously improve, remain the same, or even degrade.
Levels of AI Systems start at traditional software (Level 0) and progress up to fully Intelligent software (Level 4). Systems at Level 4 essentially maintain and improve on their own – they require negligible work. At ASAPP we call Level 4 AI Native®.
Moving up a level has trade-offs for practitioners and customers. For example, moving from Level 1 to Level 2 reduces ongoing data requirements and customization work, but introduces a self-reinforcing bias problem that could cause the system to degrade over time. Choosing to move up a level requires practitioners to recognize the new challenges, and the actions to take in designing an AI system.
While there are significant benefits in scalability (and typically performance/robustness/etc) in moving up levels, it’s important to say that most systems are best designed at Level 0 or Level 1. These levels are the most predictable: performance should remain roughly stable over time, and there are obvious mechanisms to improve performance (e.g. for Level 1, add more annotated training data).
AI Levels
Designing AI systems is different from traditional software development because the behavior of the system is learned – and can potentially change over time once deployed. When practitioners build AI systems, it can be useful to talk about their “level”, just like SAE has levels for self-driving cars.
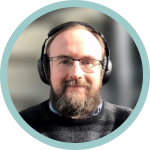
Moving up a level has trade-offs for practitioners and customers. This requires practitioners to recognize the new challenges, and the actions to take in designing an AI system
Michael Griffiths, Senior Director, Data Science, ASAPP
Level 0: Deterministic
No required training data, no required testing data
Algorithms that involve no learning (e.g. adapting parameters to data) are at level zero.
The great benefit of level 0 (traditional algorithms in computer science) is that they are very reliable and, if you solve the problem, can be shown to be the optimal solution. If you can solve a problem at level 0 it’s hard to beat. In some respect, all algorithms–even sorting algorithms (like binary search) – are “adaptive” to the data. We do not generally consider sorting algorithms to be “learning”. Learning involves memory–the system changing how it behaves in the future, based on what it’s learned in the past.
However, some problems defy a pre-specified algorithmic solution. The downside is that for problems that defy human understanding (either once, or in number) it can be difficult to perform well (e.g. speech to text, translation, image recognition, utterance suggestion, etc.).
Examples:
- Luhn Algorithm for credit card validation
- Regex-based systems (e.g. simple redaction systems for credit card numbers).
- Information retrieval algorithms like TFIDF retrieval or BM25.
- Dictionary-based spell correction.
Note: In some cases, there can be a small number of parameters to tune. For example, ElasticSearch provides the ability to modify BM25 parameters. We can regard these as tuning parameters, i.e. set and forget. This is a blurry line.
Level 1: Learned
Static training data, static testing data
Systems where you train the model in an offline setting and deploy to production with “frozen” weights. There may be an updating cadence to the model (e.g. adding more annotated data), but the environment the model operates in does not affect the model.
The benefit of level 1 is that you can learn and deploy any function at the modest cost of some training data. This is a great place to experiment with different types of solutions. And, for problems with common elements (e.g. speech recognition) you can benefit from diminishing marginal costs.
The downside is that customization to a single use case is linear in their number: you need to curate training data for each use case. And that can change over time, so you need to continuously add annotations to preserve performance. This cost can be hard to bear.
Examples:
- Custom text classification models
- Speech to text (acoustic model)
Level 2: Self-learning
Dynamic + static training data, static testing data
Systems that use training data generated from the system for the model to improve. In some cases, the data generation is independent of the model (so we expect increasing model performance over time as more data is added); in other cases, the model intervening can reinforce model biases and performance can get worse over time. To eliminate the chance of reinforcing biases, practitioners need to evaluate new models on static (potentially annotated) data sets.
Level 2 is great because performance seems to improve over time for free. The downside is that, left unattended, the system can get worse – it may not be consistent in getting better with more data. The other limitation is that some systems at level two might have limited capacity to improve as they essentially feed on themselves (generating their own training data); addressing this bias can be challenging.
Examples:
- Naive spam filters
- Common speech to text models (language model)
Level 3: Autonomous (or self-correcting)
Dynamic training data, dynamic test data
Systems that both alter human behavior (e.g. recommend an action and let the user opt-in) and learn directly from that behavior, including how the systems’ choice changes the user behavior. Moving from Level 2 to 3 potentially represents a big increase in system reliability and total achievable performance.
Level 3 is great because it can consistently get better over time. However, it is more complex: it might require truly staggering amounts of data, or a very carefully designed setup, to do better than simpler systems; its ability to adapt to the environment also makes it very hard to debug. It is also possible to have truly catastrophic feedback loops. For example, a human corrects an email spam filter – however, because the human can only ever correct misclassifications that the system made, it learns that all its predictions are wrong and inverts its own predictions.
Level 4: Intelligent (or globally optimizing)
Dynamic training data, dynamic test data, dynamic goal
Systems that both dynamically interact with an environment and globally optimizes (e.g. towards some set of downstream objectives), e.g. facilitating an agent while optimizing for AHT and CSAT, or optimizing directly for profit. For example, an AutoCompose system that optimizes for the best series of clicks to optimize the conversation.
Level 4 can be very attractive. However, it is not always obvious how to get there, and unless carefully designed, these systems can optimize towards degenerate solutions. Aiming them at the right problem, shaping the reward, and auditing its behavior are large and non-trivial tasks.
Why consider levels?
Designing and building AI systems is difficult. A core part of that difficulty is understanding how they change over time (or don’t change!): how the performance, and maintenance cost, of the system will develop.
In general, there is increasing value as you move up levels, e.g. one goal might be to move a system operating at Level 1 to be at Level 2 – but complexity (and cost) of system build also increases as levels go up. It can make a lot of sense to start with a novel feature at a “low” level, where the system behavior is well understood, and progressively increase the level – as understanding the failure cases of the system becomes more difficult as the level increases.
The focus should be on learning about the problem and the solution space. Lower levels are more consistent and can be much better avenues to explore possible solutions than higher levels, whose cost and variability in performance can be large hindrances.
This set of levels provides some core breakpoints for how different AI systems can behave. Employing these levels – and making trade-offs between levels – can help provide a shorthand for differences post-deployment.
Matrix Layout
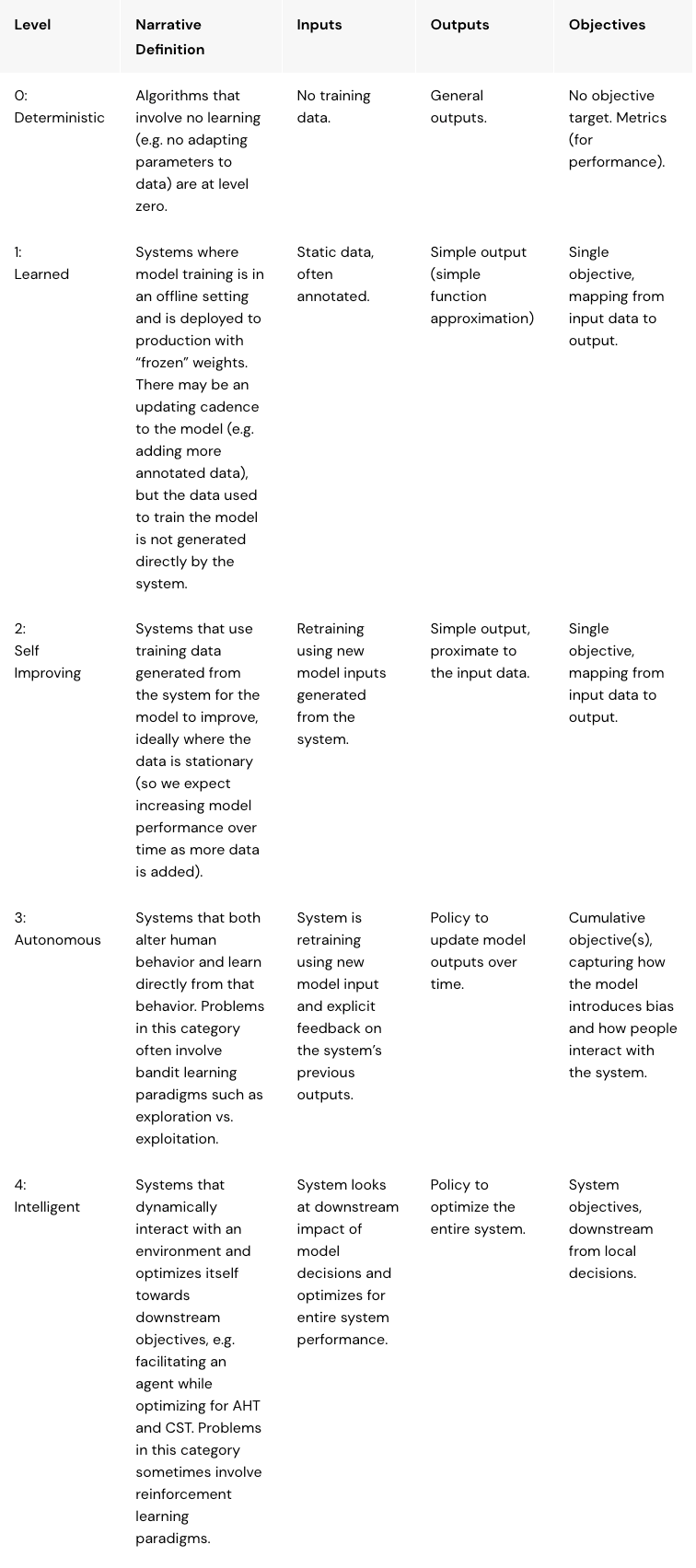
Balancing customer expectations with efficiency
Is your technology working against your agents?
Wav2vec could be more efficient, so we created our own pre-trained ASR Model for better Conversational AI.
In recent years, research efforts in natural language processing and computer vision have worked to improve the efficiency of pre-trained models to avoid the financial and environmental costs associated with training and fine-tuning them. For whatever reason, we have not seen such efforts in speech. In addition to saving costs associated with more efficient training of pre-trained models, for speech, efficiency gains could also mean greater performance for similar inference times.
Today, Wav2vec 2.0 (W2V2) is arguably the most popular approach for using self-supervised training in speech. It has received a lot of attention and follow-up works for applying pre-trained W2V2 models to various downstream applications including speech-to-text translation (Wang et al., 2021) and named entity recognition (Shon et al., 2021). Yet, we hypothesize that there are many sub-optimal design choices in the model architecture that make it relatively inefficient. To justify this hypothesis, we conducted a series of experiments on different components of the W2V2 model architecture and exposed the performance-efficiency tradeoff of the W2V2 model design space. Higher performance (lower word error rate in ASR) requires a large pre-trained model and comes with lower efficiency (inference speed). Can we achieve a better tradeoff (similar performance with higher inference speed)?
What do we propose instead? A more efficient pre-trained model that also achieves better performance through its efficiency gains.
Squeezed and Efficient Wav2vec (SEW)
Based on our observations, we propose SEW (Squeezed and Efficient Wav2vec) and SEW-D (SEW with Disentangled attention) which can achieve a much better performance-efficiency tradeoff—with 1.9x speedup during inference, our smaller SEW-D-mid achieves 13.5% WERR (word error rate reduction) compared to W2V2-base on academic datasets. Our larger SEW-D-base+ model performs close to W2V2-large while operating at the same speed as W2V2-base. It only takes 1/4 of the training epochs to outperform W2V2-base which significantly reduces the pre-training cost.
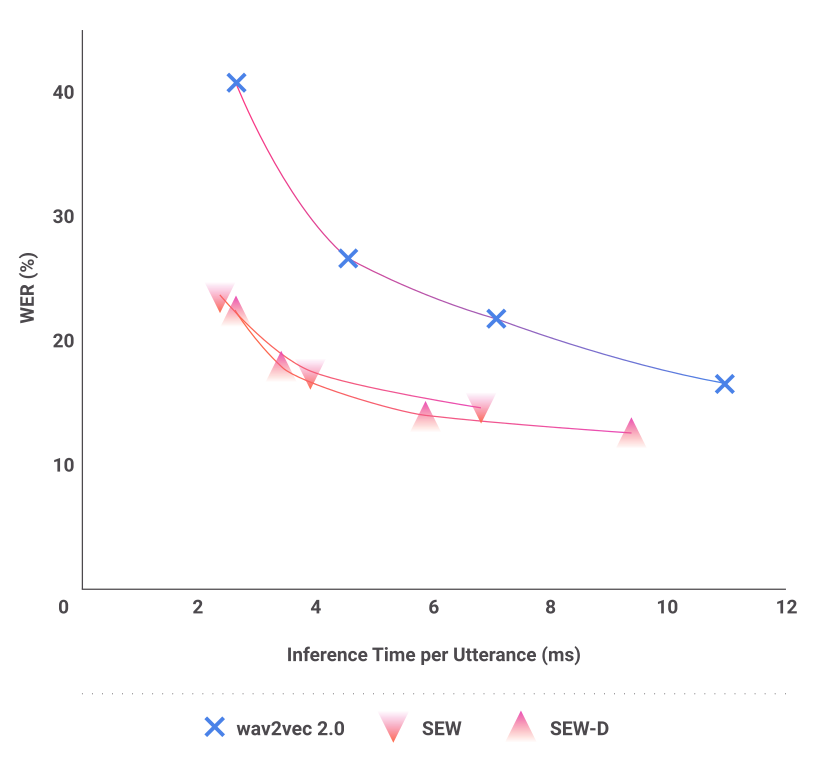
SEW differs from conventional W2V2 models in three major modifications.
First, we introduce a compact waveform feature extractor which allocates the computation across layers more evenly. This makes the model faster without sacrificing performance.
- Second, we propose a “squeeze context network” which downsamples the audio sequence and reduces the computation and memory usage.
- This allows us to use a larger model without sacrificing inference speed.
- Third, we introduce MLP predictor heads during pre-training which improve the performance without any overhead in the downstream application since they will be discarded after pre-training.

SEW-D further replaces the normal self-attention with disentangled self-attention proposed in DeBERTa (He et al., 2020) which achieves better performance with half of the number of parameters and a significant reduction in both inference time and memory footprint.
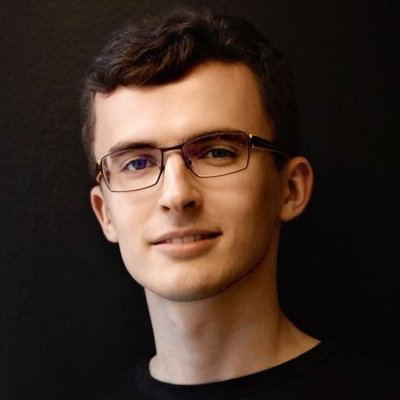
The SEW speech models by ASAPP are faster and require less memory, without sacrificing recognition quality. The architecture improvements proposed by the team are very easy to apply to other existing Wav2Vec-based models – essentially granting performance gains for free in applications such as automatic speech recognition, speaker identification, intent classification, and emotion recognition.
Anton Lozhkov
Why it matters
These pre-trained models open the door for cost savings and/or performance gains for a number of downstream models in automatic speech recognition, speaker identification, intent classification, emotion recognition, sentiment analysis and named entity recognition. The speedup of a pre-trained model can be directly transferred to the downstream models. Because the pre-trained model is smaller and faster, the fine-tuned downstream model is also smaller and faster. These efficiency gains not only reduce their training/fine-tuning time but also the actual observed latency in products. Conversational AI systems using the SEW pre-trained models will be able to better detect what consumers are saying, who’s saying what, how they feel, and to provide faster response times.
“The SEW speech models by ASAPP are faster and require less memory, without sacrificing recognition quality,” explains Anton Lozhkov, Machine Learning Engineer at Hugging Face. “The architecture improvements proposed by the team are very easy to apply to other existing Wav2Vec-based models – essentially granting performance gains for free in applications such as automatic speech recognition, speaker identification, intent classification, and emotion recognition.”
Want to utilize the pre-trained models from ASAPP? See our paper and open source code for more details. Moreover, our pre-trained models are now available in Hugging Face’s transformers library and model hub. Our paper is accepted and will appear at ICASSP 2022. Please feel free to reach out to the authors in the post-session during the conference.
Designed to be proficient on day 1
Across the globe thousands of customer care agents are starting their jobs today. For most, months of training lay ahead of them as they absorb a parade of policies, procedures, programs, and product details.
The lengthy onboarding process is a costly investment for both agent and employer because it’s typically time spent siphoning away your most seasoned agents from where they’re needed most and instead using them for training. Furthermore, when you consider the fact that agent churn can reach as high as 100%, you’ll soon find that an alarming percentage of an agent’s tenure is training, and not customer care. That’s why getting up to speed fast matters, and the two most-cited pain points are consistently the tools and the subject matter.
Must enterprise mean complicated?
Easily one of the biggest barriers for agents to achieve proficiency is the legacy CRM and chat software that sits in front of them. It’s typically a fossilized enterprise UI with little consideration for agent experience – not to mention customer experience.
Historically, there has been a perceived conflict between designing an enterprise UI that’s both performant and intuitive. The two were thought to be mutually exclusive because in an effort to maximize efficiency, speed, and accuracy, designers would emphasize information density, keyboard commands, hidden shortcuts, and sequences that created a painfully steep learning curve.
You’ll find this trend in professional tools and interfaces across finance, customer care, aviation, and beyond. While these interfaces do emphasize clarity, contrast, predictability, and priority they all require weeks or even months of training to be proficient.
A focus on the familiar
The ASAPP Product Design Team faced similar challenges as our Digital Interactions application grew to support a wide range of augmentation features. The powerful agent desk UI incorporates dozens of ML-driven features designed to help converse, investigate, solve, document—and service multiple customers simultaneously.
On the one hand, we have the opportunity and privilege of designing for a captive audience: a professional user. In a performance-based setting, you’d be correct in assuming that we’d focus on keyboard shortcuts, shortcodes, intelligent search, summarization, minimizing clicks – all of those tricks that, once learned, provide crucial efficiency gains. However, we also have to be careful to not alienate the novice user with a steep learning curve of advanced or hidden features, particularly when we consider the high cost of onboarding due to turnover. That means our application needs to be easy to onboard with a goal of being proficient on day 1 with not just the UI, but also the subject matter.
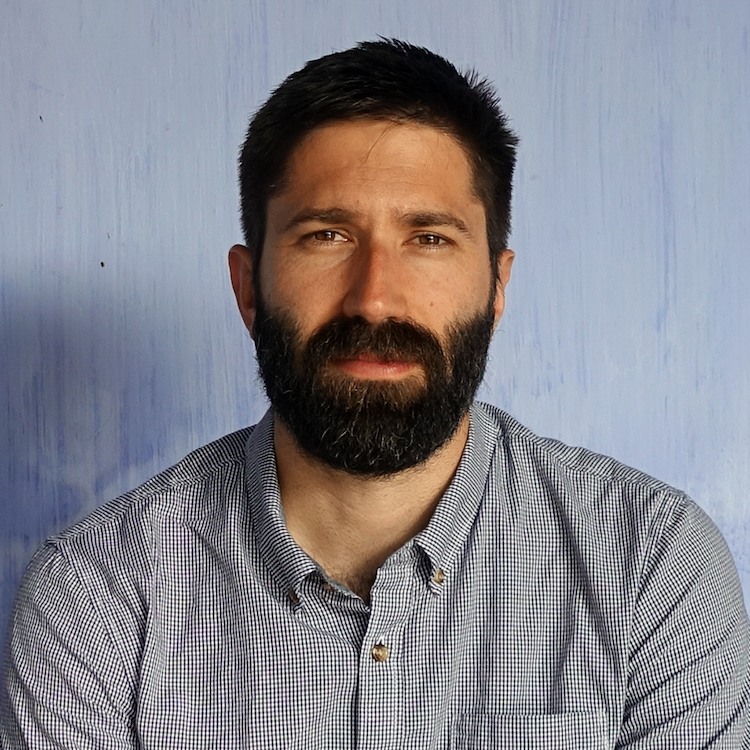
To minimize agent onboarding time we took inspiration from familiar consumer-grade UI.
In an effort to minimize agent onboarding time, the Design team focused on the familiar. We took inspiration from consumer-grade UI and affordances from phones, gaming, dashboards, alarm clocks, and more. The goal was to make new agents who sit down in front of our agent desk feel like they’ve used it before, because in many ways, they had. Not what you’d expect when you think of enterprise software.
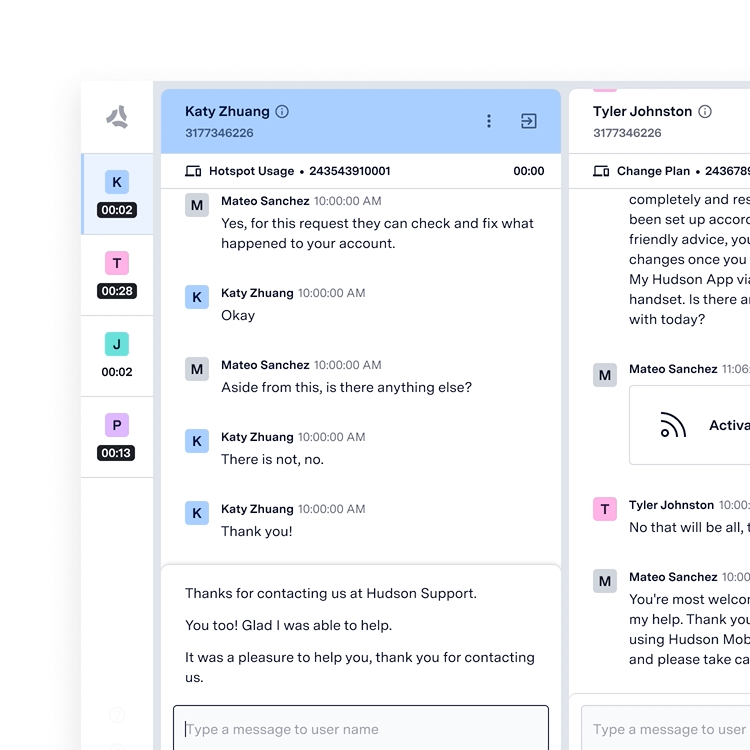
Progressive Timers

Phrase AutoComplete
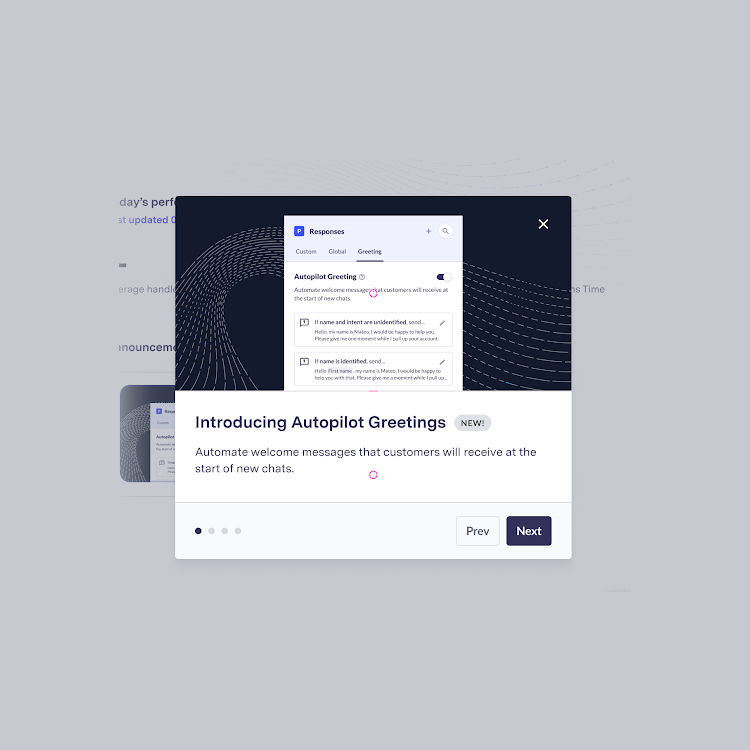
In-app onboarding

Automated workflow
Beyond the UI design, the team also focused on an interactive program of onboarding prompts and tasks that gradually familiarize the agent with the more advanced capabilities. This approach of progressive disclosure takes advantage of engagement-based tool-tips, shortcuts, in-app coaching, and personalization features.
The what, not just the how
Knowing the tools is only half the battle for new agents. They still need to become subject matter experts if they are to become truly proficient. That’s why ASAPP invests heavily in augmentation features that are designed to help even the most novice of agents to become seasoned experts.
For example, ASAPP jumpstarts an agent’s experience with AutoCompose, which recommends responses that are known to be effective in that specific situation – often sourced from the most trusted and successful agents.
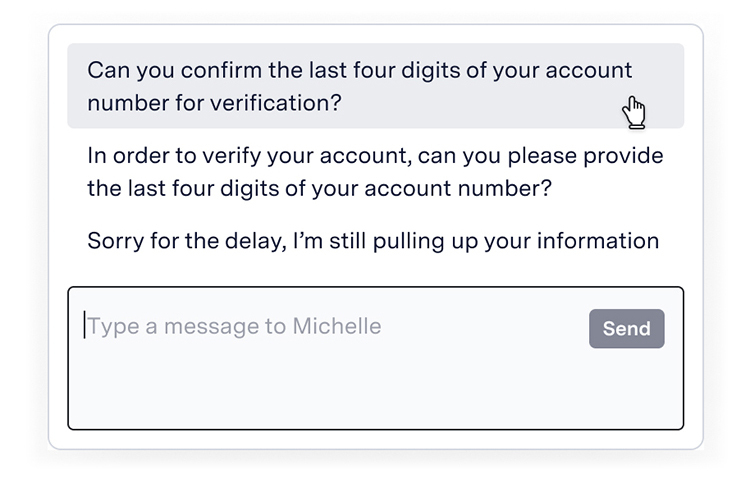

In addition, Knowledge Base recommendations provide agents timely reference content to help troubleshoot issues they’re unfamiliar with. It’s an ever-listening assistant, instantly putting resources at their fingertips. Both features draw from machine learning the actions and experience of the very best agents, quickly making new agents as effective as the most tenured.
An onboarding ally
In combining an intuitive user experience with intelligent recommendations, we’ve created an experience that is designed to make agents successful, faster. What’s more, when combined with an interactive, personalized onboarding program, we begin to shift much of the training from in-the-classroom to on-the-job, saving both time and money.