Blog
Is your technology working against your agents?
Wav2vec could be more efficient, so we created our own pre-trained ASR Model for better Conversational AI.
In recent years, research efforts in natural language processing and computer vision have worked to improve the efficiency of pre-trained models to avoid the financial and environmental costs associated with training and fine-tuning them. For whatever reason, we have not seen such efforts in speech. In addition to saving costs associated with more efficient training of pre-trained models, for speech, efficiency gains could also mean greater performance for similar inference times.
Today, Wav2vec 2.0 (W2V2) is arguably the most popular approach for using self-supervised training in speech. It has received a lot of attention and follow-up works for applying pre-trained W2V2 models to various downstream applications including speech-to-text translation (Wang et al., 2021) and named entity recognition (Shon et al., 2021). Yet, we hypothesize that there are many sub-optimal design choices in the model architecture that make it relatively inefficient. To justify this hypothesis, we conducted a series of experiments on different components of the W2V2 model architecture and exposed the performance-efficiency tradeoff of the W2V2 model design space. Higher performance (lower word error rate in ASR) requires a large pre-trained model and comes with lower efficiency (inference speed). Can we achieve a better tradeoff (similar performance with higher inference speed)?
What do we propose instead? A more efficient pre-trained model that also achieves better performance through its efficiency gains.
Squeezed and Efficient Wav2vec (SEW)
Based on our observations, we propose SEW (Squeezed and Efficient Wav2vec) and SEW-D (SEW with Disentangled attention) which can achieve a much better performance-efficiency tradeoff—with 1.9x speedup during inference, our smaller SEW-D-mid achieves 13.5% WERR (word error rate reduction) compared to W2V2-base on academic datasets. Our larger SEW-D-base+ model performs close to W2V2-large while operating at the same speed as W2V2-base. It only takes 1/4 of the training epochs to outperform W2V2-base which significantly reduces the pre-training cost.
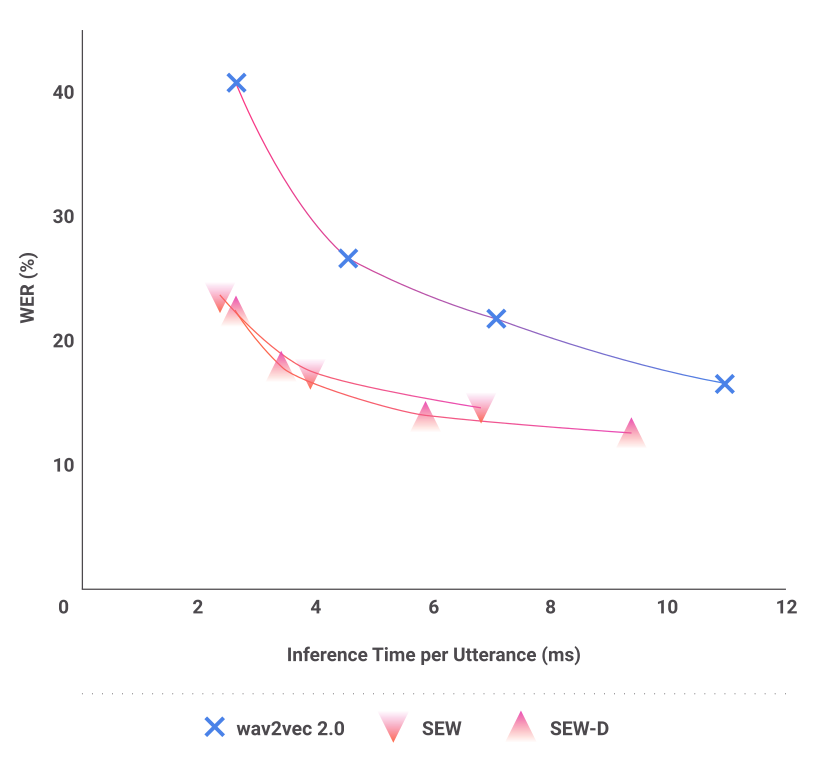
SEW differs from conventional W2V2 models in three major modifications.
First, we introduce a compact waveform feature extractor which allocates the computation across layers more evenly. This makes the model faster without sacrificing performance.
- Second, we propose a “squeeze context network” which downsamples the audio sequence and reduces the computation and memory usage.
- This allows us to use a larger model without sacrificing inference speed.
- Third, we introduce MLP predictor heads during pre-training which improve the performance without any overhead in the downstream application since they will be discarded after pre-training.

SEW-D further replaces the normal self-attention with disentangled self-attention proposed in DeBERTa (He et al., 2020) which achieves better performance with half of the number of parameters and a significant reduction in both inference time and memory footprint.
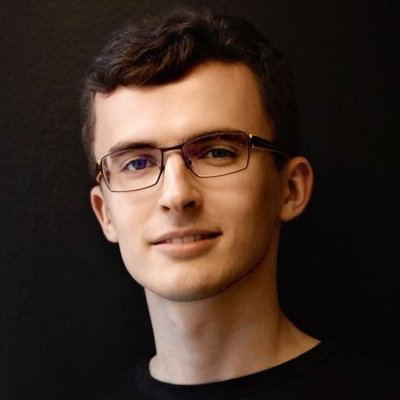
The SEW speech models by ASAPP are faster and require less memory, without sacrificing recognition quality. The architecture improvements proposed by the team are very easy to apply to other existing Wav2Vec-based models – essentially granting performance gains for free in applications such as automatic speech recognition, speaker identification, intent classification, and emotion recognition.
Anton Lozhkov
Why it matters
These pre-trained models open the door for cost savings and/or performance gains for a number of downstream models in automatic speech recognition, speaker identification, intent classification, emotion recognition, sentiment analysis and named entity recognition. The speedup of a pre-trained model can be directly transferred to the downstream models. Because the pre-trained model is smaller and faster, the fine-tuned downstream model is also smaller and faster. These efficiency gains not only reduce their training/fine-tuning time but also the actual observed latency in products. Conversational AI systems using the SEW pre-trained models will be able to better detect what consumers are saying, who’s saying what, how they feel, and to provide faster response times.
“The SEW speech models by ASAPP are faster and require less memory, without sacrificing recognition quality,” explains Anton Lozhkov, Machine Learning Engineer at Hugging Face. “The architecture improvements proposed by the team are very easy to apply to other existing Wav2Vec-based models – essentially granting performance gains for free in applications such as automatic speech recognition, speaker identification, intent classification, and emotion recognition.”
Want to utilize the pre-trained models from ASAPP? See our paper and open source code for more details. Moreover, our pre-trained models are now available in Hugging Face’s transformers library and model hub. Our paper is accepted and will appear at ICASSP 2022. Please feel free to reach out to the authors in the post-session during the conference.
Designed to be proficient on day 1
Across the globe thousands of customer care agents are starting their jobs today. For most, months of training lay ahead of them as they absorb a parade of policies, procedures, programs, and product details.
The lengthy onboarding process is a costly investment for both agent and employer because it’s typically time spent siphoning away your most seasoned agents from where they’re needed most and instead using them for training. Furthermore, when you consider the fact that agent churn can reach as high as 100%, you’ll soon find that an alarming percentage of an agent’s tenure is training, and not customer care. That’s why getting up to speed fast matters, and the two most-cited pain points are consistently the tools and the subject matter.
Must enterprise mean complicated?
Easily one of the biggest barriers for agents to achieve proficiency is the legacy CRM and chat software that sits in front of them. It’s typically a fossilized enterprise UI with little consideration for agent experience – not to mention customer experience.
Historically, there has been a perceived conflict between designing an enterprise UI that’s both performant and intuitive. The two were thought to be mutually exclusive because in an effort to maximize efficiency, speed, and accuracy, designers would emphasize information density, keyboard commands, hidden shortcuts, and sequences that created a painfully steep learning curve.
You’ll find this trend in professional tools and interfaces across finance, customer care, aviation, and beyond. While these interfaces do emphasize clarity, contrast, predictability, and priority they all require weeks or even months of training to be proficient.
A focus on the familiar
The ASAPP Product Design Team faced similar challenges as our Digital Interactions application grew to support a wide range of augmentation features. The powerful agent desk UI incorporates dozens of ML-driven features designed to help converse, investigate, solve, document—and service multiple customers simultaneously.
On the one hand, we have the opportunity and privilege of designing for a captive audience: a professional user. In a performance-based setting, you’d be correct in assuming that we’d focus on keyboard shortcuts, shortcodes, intelligent search, summarization, minimizing clicks – all of those tricks that, once learned, provide crucial efficiency gains. However, we also have to be careful to not alienate the novice user with a steep learning curve of advanced or hidden features, particularly when we consider the high cost of onboarding due to turnover. That means our application needs to be easy to onboard with a goal of being proficient on day 1 with not just the UI, but also the subject matter.
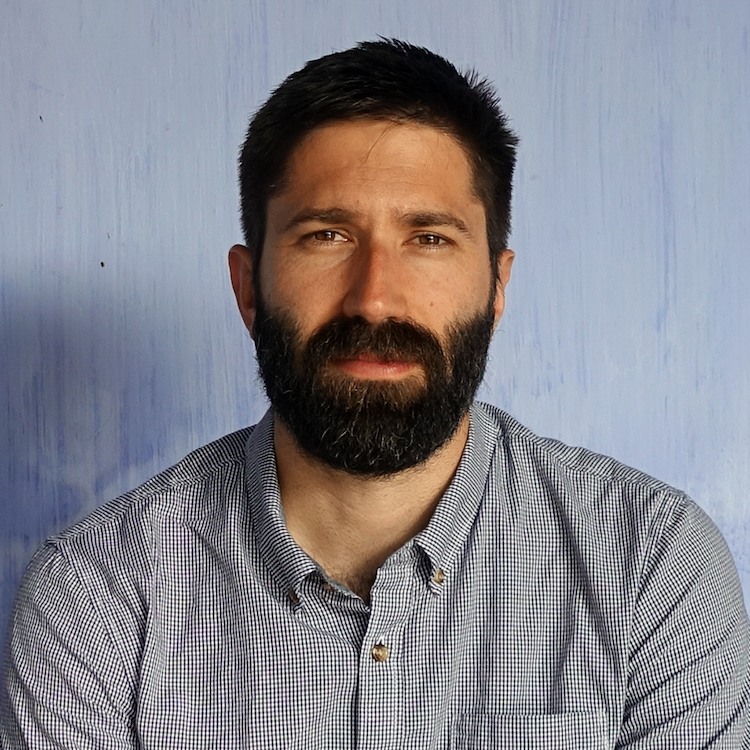
To minimize agent onboarding time we took inspiration from familiar consumer-grade UI.
In an effort to minimize agent onboarding time, the Design team focused on the familiar. We took inspiration from consumer-grade UI and affordances from phones, gaming, dashboards, alarm clocks, and more. The goal was to make new agents who sit down in front of our agent desk feel like they’ve used it before, because in many ways, they had. Not what you’d expect when you think of enterprise software.
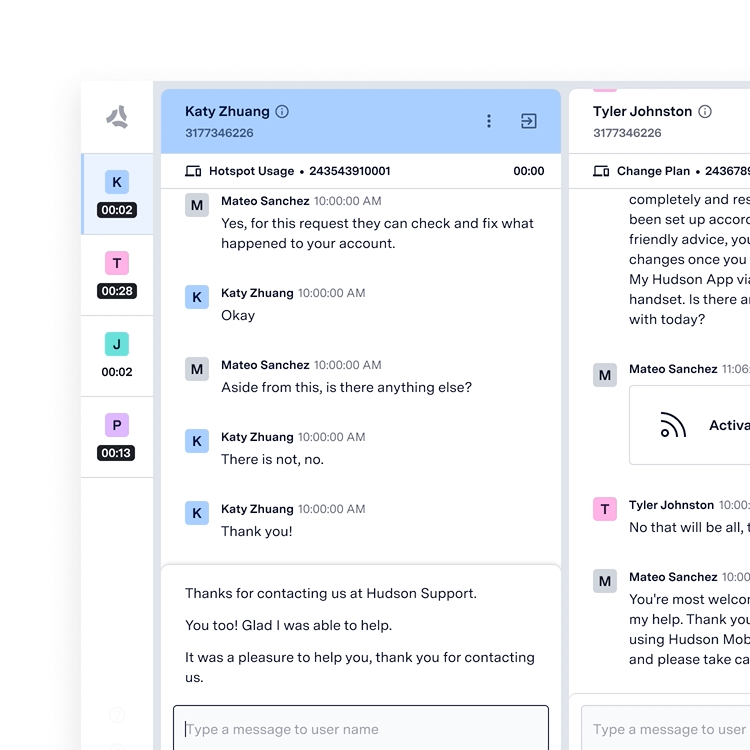
Progressive Timers

Phrase AutoComplete
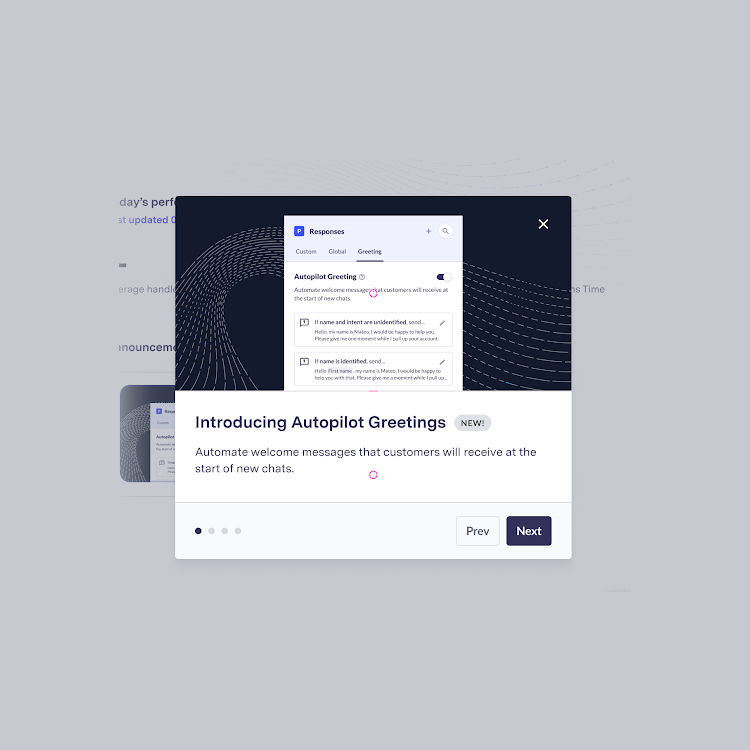
In-app onboarding

Automated workflow
Beyond the UI design, the team also focused on an interactive program of onboarding prompts and tasks that gradually familiarize the agent with the more advanced capabilities. This approach of progressive disclosure takes advantage of engagement-based tool-tips, shortcuts, in-app coaching, and personalization features.
The what, not just the how
Knowing the tools is only half the battle for new agents. They still need to become subject matter experts if they are to become truly proficient. That’s why ASAPP invests heavily in augmentation features that are designed to help even the most novice of agents to become seasoned experts.
For example, ASAPP jumpstarts an agent’s experience with AutoCompose, which recommends responses that are known to be effective in that specific situation – often sourced from the most trusted and successful agents.
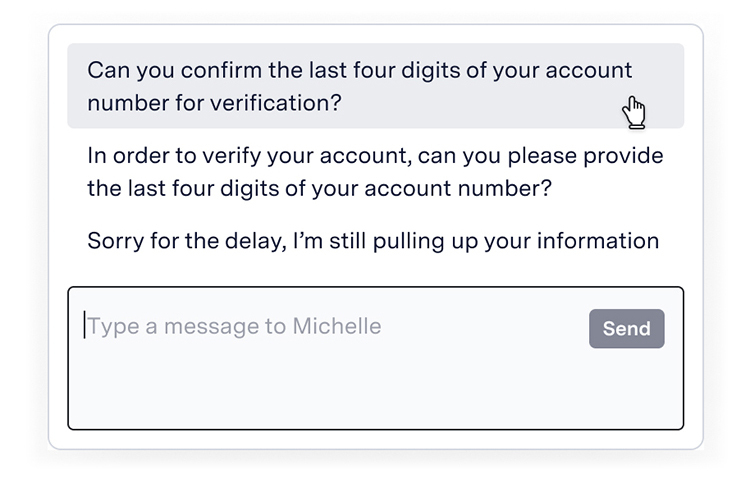

In addition, Knowledge Base recommendations provide agents timely reference content to help troubleshoot issues they’re unfamiliar with. It’s an ever-listening assistant, instantly putting resources at their fingertips. Both features draw from machine learning the actions and experience of the very best agents, quickly making new agents as effective as the most tenured.
An onboarding ally
In combining an intuitive user experience with intelligent recommendations, we’ve created an experience that is designed to make agents successful, faster. What’s more, when combined with an interactive, personalized onboarding program, we begin to shift much of the training from in-the-classroom to on-the-job, saving both time and money.
How anomaly detection helps you handle the unexpected
Every day, ASAPP customers handle tens of thousands of inquiries to their contact centers. We have a pretty solid understanding of what the majority of these requests are about. Most are routine issues that agents have experience dealing with. However, on any given day, there is the risk that a customer may be slammed with hundreds or thousands of calls about something out of the ordinary. These events might range from a popular pay-per-view fight, to service outages, to a UI change confusing half the user base. On those days, ASAPP anomaly detection is there to help.
Every conversation that comes into a content center and enters our infrastructure contains a problem statement somewhere within. Problem statements help direct agents towards the caller’s needs, and help contact center managers understand broader analytics for key traffic drivers. In messaging channels, the user is asked to input their problem directly. For voice channels, ASAPP speech-to-text transcription feeds an NLP extraction model that pulls the problem statement out.
We’ve seen a wide variety of problem statements over the years. Most of the time, our augmentation methods help agents swiftly resolve standard issues. But what happens when something totally new appears? When the unexpected causes an influx of inquiries about an unfamiliar issue? How do we quickly identify this new behavior and extract the conversations so our customers can more effectively address them? How do we know if our system is recognizing the right changes in language? And how do we measure the impact of these new behaviors?
The answer we found was to train a model to distinguish the problem statements coming in now from those that have appeared before, comparing the current stream of requests to the history of past requests. On an average day, the data stream coming in looks much like the historical data, full of similar inquiries about common issues. This makes it difficult to train a model to differentiate the current stream of data from the past.
But on an interesting day, the language in our data stream looks significantly different, containing words or phrases that don’t normally appear.
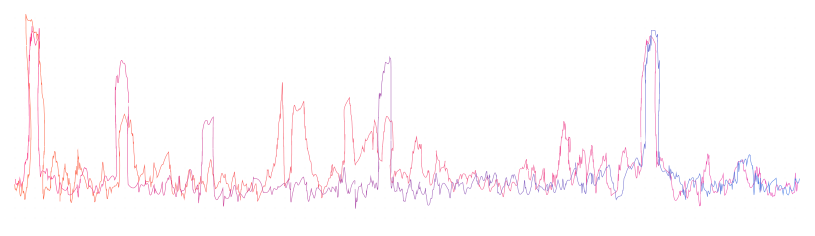
This is where our trained model becomes highly confident it can distinguish the current stream of data from our historical record. Moreover, on these days, the new problem statements typically reflect a single shared issue and include similar language on that topic. This helps explain exactly what issue caused users to hammer a customer’s contact center with traffic in a clearly defined set of words.

Our models provide actionable intelligence by directly surfacing customer complaints in real time, and even can measure the impact of a problem as it is occurring. All of this is to help contact center teams better recognize and react to new customer behaviors as they develop.
In the process of training a model to distinguish current behavior from past, we’ve also gotten:
- a list of high confidence problem statements representing the new behavior in the data
- a model for extracting whatever topic set off the alarm
That new dataset can be used to measure how much traffic is due to the novel problem we discovered. Plus, we can train a classifier to detect the problem the next time it occurs. The trained model can be used to extract historical volumes to see if this issue has happened in the past. It can also be used on incoming data to easily identify this new behavior in the future. Moreover, the models we trained are naturally interpretable, yielding key topic words that can be used in SQL queries for easy analysis.
The system ASAPP built around this new technology operates on a minute time scale. Our models provide actionable intelligence by directly surfacing customer complaints in real time, and even can measure the impact of a problem as it is occuring. All of this is to help contact center teams better recognize and react to new customer behaviors as they develop. Equipped with anomaly detection, CX organizations can more efficiently address unexpected events, then analyze these unique situations to understand and prepare for them.
The keys to CX success in 2022 (and beyond)
The past two years have fundamentally and permanently changed how we think about and approach customer service. Faced with an ongoing pandemic, the shift to remote work, supply chain disruptions, and the “Great Resignation,” companies have been forced to adapt and evolve at an incredible pace, re-examining the role of customer service and the critical link it provides between their customers and their brand.
As we move into the new year, brands will need to continue to advance every aspect of CX to stay competitive, as customer expectations (and frustrations) reach an all-time high. But how to best serve customers, grow revenue, and empower workers, all while keeping costs down? Here are four things forward-thinking CX organizations are doing right, right now.
Elevating the Frontline Agent Experience
Contact center agents represent the voice of your brand. It has always been one of the most difficult and important jobs in any company, and an underserved part of the customer + brand ecosystem. But in the wake of the Great Resignation, there is an even more critical need for companies to focus on agent and employee satisfaction.
It has become increasingly difficult to attract and retain talent in the current environment. With demand at an all-time high, agents are migrating to companies that value them and prioritize employee well-being, flexibility, and engagement. To reduce churn and maintain a motivated workforce, leading companies are actively working to make agents’ jobs better.
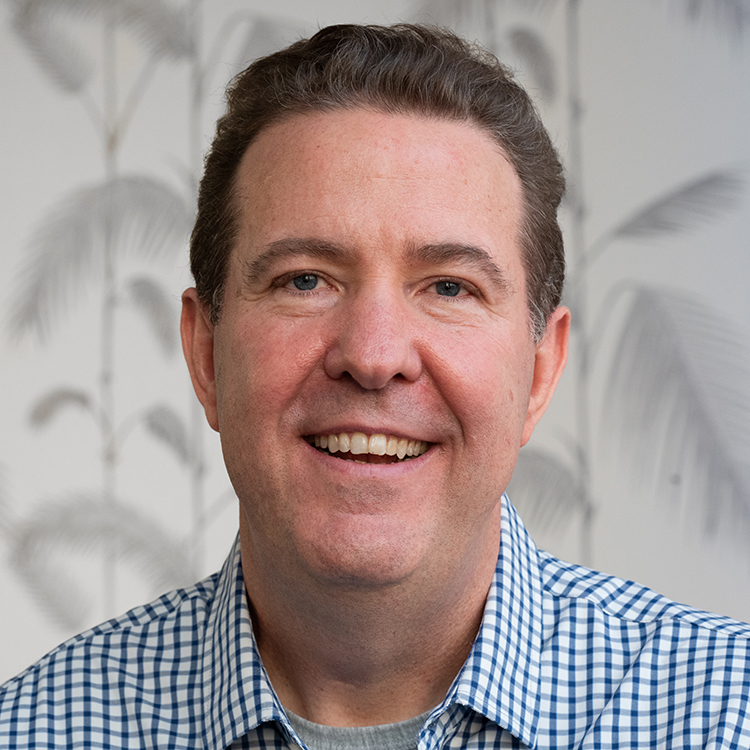
If you want to provide a great customer experience, start with the voice of your brand—your agents. When we look at the fundamental forces at play in evolving CX, it is essential to focus on the people at the center of the conversation.
It starts with focusing attention and resources on training that engages employees from day one and accelerates time to proficiency. Employing AI to improve onboarding and coaching is one way forward-thinking companies are flattening the learning curve. Advanced machine learning can now analyze every word and action taken by top agents in real time, compiling best practices to guide others on how to handle any customer request. With this streamlined approach, new agents get up to speed faster while building competence and confidence.
In addition to training, agents need technology that supports, not overwhelms. Many today are faced with a jumbled stack of applications and processes that can make completing tasks difficult. Companies are starting to be more mindful of the entire journey agents take to fulfill each customer request. With that knowledge, they can identify where to engage automation to reduce tedious tasks and streamline workflows, enabling agents to concentrate on what they actually want to do—help customers.
Making Customer Experience a Feature
There are more ways to engage with customers than ever before. Due to the global pandemic, digital channels more quickly became the norm, and companies are making themselves available on a growing number of platforms (e.g., Apple Messages for Business, Google Business Messaging, SMS, and more). Most consumers now expect unlimited access to their favorite brands through seamless multichannel service. The companies succeeding in CX are those that continue to move aggressively towards these digital and asynchronous experiences. Creating a cohesive cross-channel experience is key to both automated and human-driven modalities, allowing customers to engage wherever and whenever, while supplying agents with critical context to deliver personal and relevant experiences.
To maintain a competitive advantage, brands must provide memorable, elevated service that builds loyalty (and increased lifetime value). This means experiences that bring customers back again and again, making them fans of the company based not only on the product purchased, but how the company took care of them during a “moment of truth.” We are seeing more companies emphasize experience, now fully aware of its potential to differentiate their brand—and possibly turn their cost center into a profit center.
Using Data to Fuel CX Innovation
The growing move to digital, increase in smart products, and evolution of tech to capture customer behavior all mean more data for companies to digest. Faced with an almost overwhelming volume of customer and operational data, leading brands are examining how best to use this information to both improve processes and deliver more effective service. Those at the forefront have figured out how to make the data do the work for them, using AI-powered analytics to inform everything from marketing strategy to agent onboarding.
Customers today expect hyper-personalized interactions with brands, and are more likely to respond to communications that understand their specific interests and history with the company. CX teams can now employ AI to learn from every interaction with an individual, remembering and adapting to their personal preferences to tailor engagement efforts. To truly improve business outcomes, organizations have adopted platforms that make full use of AI to feed analytics and gain actionable insights—in real time. And thanks to the availability of data on past interactions along with advancements in natural language processing, companies can even engage AI services that simulate customer scenarios to train agents in more dynamic, real-world settings before they get on an actual call or chat.
Building a Continuous Cycle of Automation
As noted, one trend increasingly evident in CX is the growing confidence in and adoption of AI. The key here is finding the right balance between automation and humanity. Customers still want human interaction, perhaps more than ever after years of less than optimal chatbot conversations. While bots continue to serve a role, there is a push now to find smarter ways to engage automation for both customers and agents.
What is commonly referred to as “agent assist” has become a core capability. But this basic feature is only the beginning of how CX teams can use AI to achieve significant productivity gains and better customer experiences. The most advanced companies are exploring ways to integrate AI-driven capabilities throughout agent workflows. This unlocks the ability to not only dramatically streamline processes, but power more and more automation using the knowledge gained through machine learning. As the ML models study each interaction, they continually feed improvements to the automated experience, identifying opportunities to both further support agents and enhance self-service for customers.
Looking to the Future Now
Today’s leading companies understand that to compete they must focus on the entire ecosystem of the customer journey—and that starts with frontline agents. Ultimately, we know:
- Happy, engaged agents provide superior customer service
- Elevated experiences are the key to winning customer loyalty
- Experiential data fuels analytics to produce valuable insights
- These insights can help enhance automation, improving engagement
To succeed in 2022 and beyond, organizations not only need to understand this cycle, but foster each part of it. That is what ASAPP helps our customers do everyday.
Scaling for growth and expansion
Data like you've never seen before in this industry
GOLD: Improving Out-of-Scope Detection in Dialogues using Data Augmentation
Imagine you’re booking airline tickets through a conversational AI assistant, and after purchasing tickets, you ask for help in finding an in-home pet sitter during your trip. The conversational AI misinterprets what you mean, and instead shares details on how to board your flight with pets. This has an obvious reason: the AI has never encountered this particular task, and was unable to map it to a procedure. Thus, your request to find an in-home pet sitter was out of the distribution of what the assistant was trained to handle. Alternatively, suppose you had asked about upgrading your flight, but the system confuses your request as wanting to update your flight to a different date. In this case, the AI assistant is capable of managing flights but was unable to complete the request due to a dialogue breakdown. In both cases, we arrive at the same result: a failed conversation.
Both out of distribution requests and dialogue breakdowns described above are considered out-of-scope (OOS) situations since they represent cases that your assistant is unable to handle. To avoid customer frustration, detecting OOS scenarios becomes an essential skill of today’s conversational AI and dialogue systems. While the ideal conversational AI agent would be able to help find an in-home pet sitter as requested and manage all the complex nuances of natural language, this is simply not possible given that training data is finite and consumer queries are not. So knowing when the user is asking something in-scope vs out-of-scope can help refine conversational AI systems into better performing in their core tasks.
It can be hard to provide training data for, or even enumerate, the potentially limitless number of out-of-scope queries a dialogue system may face. However, new ASAPP research presented at the conference on Empirical Methods in Natural Language Processing (EMNLP) offers a novel way to address this limited-data problem.
Out-of-Scope Detection with Data Augmentation
We introduce GOLD (Generating Out-of-scope Labels with Data augmentation), as a new technique that augments existing data to train better out-of-scope detectors operating in low-data regimes. The key insight is that rather than training on in-scope data alone, our proposed method operates on out-of-scope data as well. Furthermore, we discover that common NLP techniques for augmenting in-scope data, such as paraphrasing, do not provide the same benefit when working with out-of-scope data.
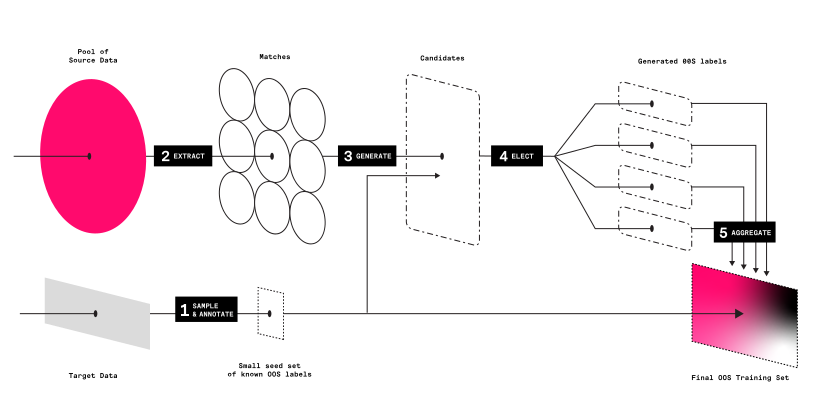
GOLD works by starting with a small seed set of known out-of-scope examples. This small amount (only 1% of the training data) is typically used by prior methods for tuning thresholds and other hyperparameters. Instead, GOLD uses this seed set of OOS examples to find semantically similar utterances from an auxiliary dataset, which yields a large set of matches. Next, we create candidate examples by replacing utterances in the known out-of-scope dialogues with the sentences found in extracted matches. Lastly, we filter down candidates to only those which are most likely to be out-of-scope. These pseudo-labeled examples created through data augmentation are then used to train the OOS detector.
The results? State-of-the-art performance across three task-oriented dialogue datasets on multiple metrics. These datasets were created by post-processing existing dialogue corpora spanning multiple domains with multi-turn interactions. Notably, the out-of-scope instances were designed as a natural progression of the conversation, rather than generated through synthetic noise or negative sampling.
Why this matters
Data augmentation is a popular method to improve model performance in low-resource settings, especially in real life settings where annotating more examples can quickly become cost-prohibitive. With just a small seed of out-of-scope examples, GOLD achieved a 10X improvement in training out-of-scope detectors compared to using the seed data alone. Previous methods relied on using tremendous amounts of labeled out-of-scope data that is unrealistic to obtain in real-world settings or relied on in-scope data alone which doesn’t provide sufficient signal for detecting OOS items.
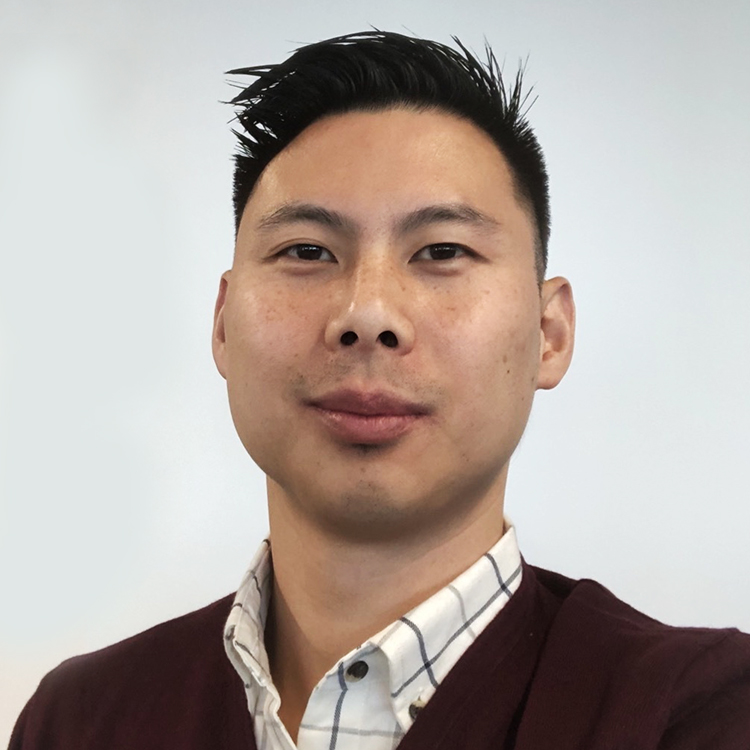
With just a small seed of out-of-scope examples, GOLD achieved a 10X improvement in training out-of-scope detectors compared to using the seed data alone.
Derek Chen
GOLD supports robustness and prevents overfitting by relying on other methods during the filtering process. As other out-of-scope detection methods improve over time, GOLD can take advantage of those gains and improve as well.
At ASAPP, we are exploring similar methods in our products to both reduce out-of-scope issues in our conversational systems, as well as improve overall systems when operating in limited data regimes. If you’re a researcher conducting work to detect more granular levels of errors, or more sophisticated methods of data efficiency, we’d love to chat! Give us a tweet at @ASAPP.
Learning to recommend what agents should do
At ASAPP, we build AI models to increase the efficiency and effectiveness of customer service agents by recommending the next action to perform during live chat. A natural starting point for creating such models is to learn to predict when the agent would perform the action based on the data we have collected in production. The data we collect are usually of the form: timestamp & event. For example:
14:28:03, AGENT ASSIGNED TO AN ISSUE
14:28:44, AGENT SENDS A MESSAGE
14:29:18, AGENT CLICKS AN AUTOMATED RESPONSE
14:31:52, AGENT ENABLES AUTOMATIC TIMEOUT TO TAKE OVER
For example, our AutoSuggest model learns the likelihood that an agent will send a certain message based on the context of the conversation and other features. Our AutoCompose service uses this model to surface the most likely next message as a suggestion to the agent, reducing their response time, lower cognitive load, and encouraging them to use preferred phrases. The actual next message sent by the agent has proven to be an effective target for AutoCompose, resulting in high usage, reduced average handle time, and positive feedback from agents.
However, sometimes what agents actually do, isn’t necessarily what they should do. And what agents actually do in production is the raw data that’s logged. If we are not careful, this is the data that will be used to train AI models, which will reinforce suboptimal agent behaviors through recommendations and automation.
This was the situation with our Automatic Timeout feature and our Flexible Concurrency feature. Automatic Timeout is an automation feature that agents can opt in to when the customer has likely left the chat. The feature will send timeout messages on behalf of the agent so that the agent can focus their effort elsewhere. The feature was a huge success in increasing agent efficiency and extremely popular with agents.
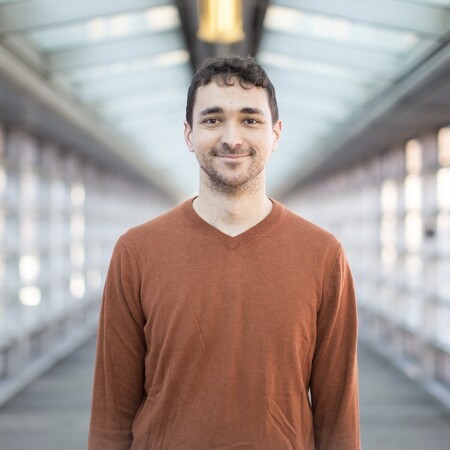
We discovered that agents would manually time out customers rather than use the feature to avoid receiving additional assignments.
Chris Fox
To improve usage of Automatic Timeout, ASAPP developed a recommendation model to recommend the Automatic Timeout feature to agents. The most natural starting point seemed to be predicting when agents were likely to use the feature, based on the usage data we had collected in production. But, there was a wrinkle.
Soon after Automatic Timeout went live, our AI-driven Flexible Concurrency feature was launched. This feature learns and predicts agent busyness. When it predicts the agent is likely to not be busy, the agent’s concurrency can be increased (flexed) without overwhelming the agent. One of the biggest predictors of an agent’s busyness is whether Automatic Timeout has been enabled. Agents began to notice that there was a correlation between using Automatic Timeout and increased concurrency. Because companies typically tie agent performance to agent handle time (rather than their throughput), agents are not incentivized to take on additional issues. As a result, usage of the Automatic Timeout feature decreased. Agents would manually time out customers rather than use the feature to avoid receiving additional assignments.
Because some agents were not using Automatic Timeout to avoid additional issue assignments, many timestamps where a recommendation would be relevant were incorrectly labeled as times not to recommend.
As an alternative to leveraging agents’ past usage of Automatic Timeout as the prediction target, we explored instead labeling each timestamp based on whether there would be no further customer actions after that point in the chat. This approach had the advantage of not being affected by some agents’ preference to manually time out the customer. It captured all cases where the customer became idle during the chat. Moreover, the model achieved high accuracy on this prediction task.
However, upon further testing, we discovered that this prediction target was in fact not as good a choice as it first appeared. The model was recommending Automatic Timeout very frequently during the end of normal chats, in which the customer issue had been resolved and the agent was closing out the conversation. The predictions were highly confident in these sections of the conversation.
Meanwhile, in cases where the customer had gone idle while the agent was waiting for them to respond, the model often predicted no recommendation or had low confidence. Looking further into the data, the reason was clear: normal chats are far more common than chats in which the customer leaves the agent waiting. As a result, the model focused on detecting normal chat endings, and worse, our evaluation metric was largely reflecting model performance in those irrelevant situations.
This is an example of a common issue in the application of AI models: the usefulness of a model depends on choosing the prediction target carefully. A poorly selected target can result both in a model that is ineffective for its intended application and an evaluation metric that obscures this fact from the model developer.
We considered further restricting the target to require, not only that the customer was inactive, but also that the conversation concludes by being timed out. However, it can be helpful for the agent to use Automatic Timeout to free them up temporarily to focus on other work, even when the customer comes back before the sequence completes in a few minutes.
In the end, we designed a more complex target that better identifies the times in the chat when it would be useful to enable Automatic Timeout, based on additional situational data and an auxiliary model. Specifically, the customer needs to have been idle for a certain period, and the next event in the chat is either an Automatic Timeout message, a timeout-like message sent manually by the agent, or the agent timing out the customer (which closes the chat and prioritizes the customer in the queue if they chat back in again). An auxiliary model is used to identify timeout-like messages. This work was primarily driven by our amazing intern Sara Price.
Data used to label Automatic Timeout recommendation model training data
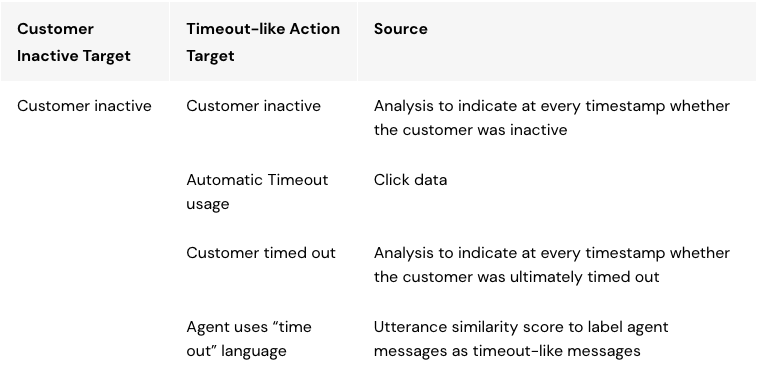
As you can see from the table above, labeling training data for the Automatic Timeout recommendation model based on what agents should do entails a lot more modeling effort than simply replying on what agents have been doing (using the feature or not). Fortunately, with the ASAPP AI Native® approach, the additional models needed to determine the type of language the agent is using are already available and can be easily consumed by the Automatic Timeout recommendation model.
With the final version of the prediction target, we achieved a better alignment between the training data, and hence the model’s behavior, and the usefulness of our recommendations. And the evaluation metric became a better indicator of how useful our model would be in practice. In some cases, simply predicting agent actions is sufficient to build helpful AI recommendations, but in other cases, as with Automation Timeout, we have found it can pay dividends to think carefully about how to engineer the training data to guide agents toward more optimal workflows.