Heather Reed
.jpg)
Heather Reed, PhD is a Product Manager at ASAPP where she applies her understanding of AI and statistical analysis to gain novel and in-depth insights into ASAPP products and users. Prior to joining ASAPP, Heather spent Spring of 2018 at NASA Langley Research Center as a Visiting Scientist and has taught model verification, validation, and uncertainty quantification; inverse problems; and the use of high performance computing. She's an educational advocate for AI and data-driven approaches in areas where non-AI solutions have been the status quo.
Preventing hallucinations in generative AI agent: Strategies to ensure responses are safely grounded
The term “hallucination” has become both a buzzword and a significant concern. Unlike traditional IT systems, Generative AI can produce a wide range of outputs based on its inputs, often leading to unexpected and sometimes incorrect responses. This unpredictability is what makes Generative AI both powerful and risky. In this blog post, we will explore what hallucinations are, why they occur, and how to ensure that AI responses are safely grounded to prevent these errors.
What is a Hallucination?
Definition and Types
In the context of Generative AI, a hallucination refers to an output that is not grounded in the input data or the knowledge base the AI is supposed to rely on. Hallucinations can be broadly categorized into two types:
- Harmless Hallucinations: These are errors that do not significantly impact the user experience or the integrity of the information provided. For example, an AI might generate a slightly incorrect but inconsequential detail in a story.
- Harmful Hallucinations: These are errors that can mislead users, compromise brand safety, or result in incorrect actions. For instance, an AI providing incorrect medical advice or financial information falls into this category.
Two Axes of Hallucinations
To better understand hallucinations, we can consider two axes. Justification - whether the AI had information indicating that its statement was true. And truthfulness - whether the statement was actually true.

Based on these axes, we can classify hallucinations into four categories:
- Justified and True: The ideal scenario where the AI’s response is both correct and based on available information.
- Justified but False: The AI’s response is based on outdated or incorrect information. This can be fixed by improving the information available. For example, if an API response is ambiguous, it can be updated so that it is clear what it is referring to.
- Unjustified but True: The AI’s response is correct but not based on the information it was given. For example, the AI telling the customer that they should arrive at an airport 2 hours before a flight departure. If this was not grounded in, say, a knowledge base article, then this information is technically a hallucination even if it is true.
- Unjustified and False: The worst-case scenario where the AI’s response is both incorrect and not based on any available information. These are harmful hallucinations that could require an organization to reach out to the customer to fix the mistake.
Why Do Hallucinations Occur?
Hallucinations in generative AI occur due to several reasons. Generative models are inherently stochastic, meaning they can produce different outputs for the same input. Additionally, the large output space of these models increases the likelihood of errors, as they are capable of generating a wide range of responses. AI systems that rely on incomplete or outdated data are also prone to making incorrect statements. Finally, the complexity of instructions can result in misinterpretation, which may cause the model to generate unjustified responses.
Hallucination prevention and management
We typically think about four pillars when it comes to preventing and managing hallucinations:
- Preventing hallucinations from occurring in the first place
- Catching hallucinations before they reach the customer
- Tracking hallucinations post-production
- Improving the system
1. Preventing Hallucinations: Ensuring Responses are Properly Grounded
One of the most effective ways to prevent hallucinations is to ensure that AI responses are grounded in reliable data. This can be achieved through:
- Explicit Instructions: Providing clear and unambiguous instructions can help the AI interpret and respond accurately.
- API Responses: Integrating real-time data from APIs ensures that the AI has access to the most current information.
- Knowledge Base (KB) Articles: Relying on a well-maintained knowledge base can provide a solid foundation for AI responses.
2. Catching hallucinations: Approaches to Catch Hallucinations in Production
To minimize the risk of hallucinations, several safety mechanisms can be implemented:
- Input Safety Mechanisms: Detecting and filtering out abusive or out-of-scope requests before they reach the AI system can prevent inappropriate responses.
- Output Safety Mechanisms: Assessing the proposed AI response for safety and accuracy before it is delivered to the user. This can involve:some text
- Deterministic Checks using rules-based systems to ensure that certain types of language or content are never sent to users, or
- Classification Models, which employ machine learning models to classify and filter out potentially harmful responses. For example, a model can classify whether the information contained in a proposed response has been grounded on information retrieved from the right data sources. If this model suspects that the information is not grounded, it can reprompt the AI system to try again with this feedback.
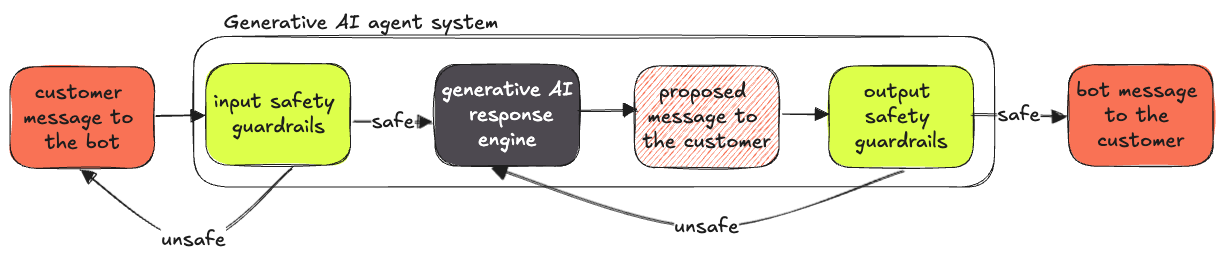
3. Tracking hallucinations: post-production
A separate post-production model can be used to classify AI responses as mistakes in more detail. While the “catching hallucination” model should balance effectiveness with latency, the post-production mistake monitoring model can be much larger, as latency is not a concern.
A well-defined hallucination taxonomy is crucial for systematically identifying, categorizing, and addressing errors in Generative AI systems. By having a well-defined error taxonomy system, users can aggregate reports that make it easy to identify, prioritize, and resolve issues quickly.
The following categories help identify the type of error, its source of misinformation, and the impact.
- Error Category -broad classification of types of the generative AI system errors.
- Error Type - specific nature or cause of an AI system error.
- Information Source - origin of data used by the AI system.
- System Source - component responsible for generating or processing AI output.
- Customer Impact Severity - level of negative effect on the customer.
4. Improving the system
Continuous improvement is crucial for managing and reducing hallucinations in AI systems. This involves several key practices. Regular updates ensure that the AI system is regularly updated with the latest data and information. Implementing feedback loops allows for the reporting and analysis of errors, which helps improve the system over time. Regular training and retraining of the AI model are essential to enable it to adapt to new data and scenarios. Finally, human oversight involving contact center supervisors to review and correct AI responses, especially in high-stakes situations, is critical.
Conclusion
By understanding the nature of hallucinations and implementing robust mechanisms to prevent, catch, and manage them, organizations can harness the power of Generative AI while minimizing risks. Just as human agents in contact centers are managed and coached to improve performance, Generative AI systems can also be continually refined to ensure they deliver accurate and reliable responses. By focusing on grounding responses in reliable data, employing safety mechanisms, and fostering continuous improvement, we can ensure that AI responses are safely grounded and free from harmful hallucinations.
Not all contact center automation is the same
At ASAPP we develop AI models to improve agent performance in the contact center. Many of these models directly assist contact center agents by automating parts of their workflow. For example, the automated responses generated by AutoCompose - part of our ASAPPMessaging platform - suggest to an agent what to say at a given point during a customer conversation. Agents often use our suggestions by clicking and sending them.
How we measure performance matters
While usage of the suggestions is a great indicator of whether the agents like the features, we’re even more interested in the impact the automation has on performance metrics like agent handle time, concurrency, and throughput. These metrics are ultimately how we measure agent performance when evaluating the impact of a product like the AutoCompose capabilities of ASAPPMessaging, but these metrics can be affected by things beyond AutoCompose usage, like changes in customer intents or poorly-planned workforce management.
To isolate the impact of AutoCompose usage on agent efficiency, we prefer to measure the specific performance gains from each individual usage of AutoCompose. We do this by measuring the impact of automated responses on agent response time, because response time is more invariant to intent shifts and organizational effects than handle time, concurrency and throughput.
By doing this, we can further analyze:
- The types of agent utterances that are most often automated
- The impact of the automated responses when different types of messages are used (in terms of time savings)
Altogether, this enables us to be data-driven about how we improve models and develop new features to have maximum impact.
Going beyond greeting and closing messages
When we train AI models to automate responses for agents, the models look for patterns in the data that can predict what to say next based on past conversation language. So the easiest things for models to learn well are the types of messages that occur often and without much variation across different types of conversations, e.g. greetings and closings. Agents typically greet and end a conversation with a customer the same way, perhaps with some specificity based on the customer’s intent.

Most AI-driven automated response products will correctly suggest greeting and closing messages at the correct time in the conversation. This typically accounts for the first 10-20% of automated response usage rates. But when we evaluate the impact of automating those types of messages, we see that it’s minimal.
To understand this, let’s look at how we measure impact. We compare agents’ response times when using automated responses against their response times when not using automated responses. The difference in time is the impact—it’s the time savings we can credit to the automation.

Without automation, agents are not manually typing greeting and closing messages for every conversation. Rather they’re copying and pasting from notepad or word documents containing their favorite messages. Agents are effective at this because they do it several times per conversation. They know exactly where their favorite messages are located, and they can quickly copy and paste them into their chat window. Each greeting or closing message might take an agent 2 seconds. When we automate those types of messages, all we are actually automating is the 2-second copy/paste. So when we see automation rates of 10-20%, we are likely only seeing a minimal impact on agent performance.
The impact lies in automating the middle of the conversation.
If automating the beginnings and endings of conversations is not that impactful, what is?

Automating the middle of the conversation is where response times are naturally slowest and where automation can yield the most agent performance impact.
- Heather Reed, Product Manager, ASAPP
The agent may not know exactly what to say next, requiring time to think or look up the right answers. It’s unlikely that the agent has a script readily available for copying or pasting. If they do, they are not nearly as efficient as they are with their frequently used greetings and closings.
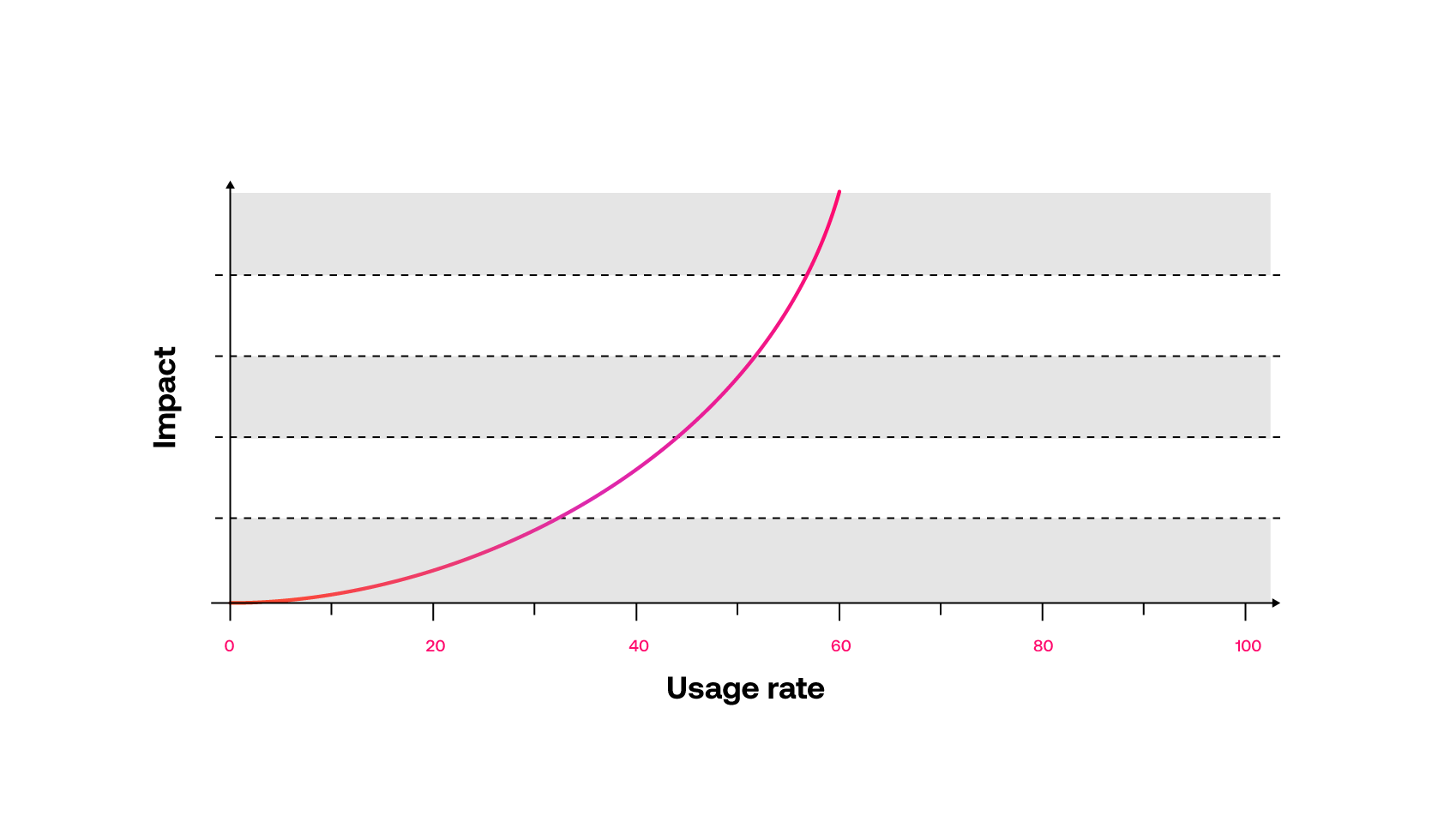
Where it was easy for AI models to learn the beginnings and endings of conversations, because they most often occur the same way, the exact opposite is true of the middle parts of conversations. Often, this is where the most diversity in dialog occurs. Agents handle a variety of customer problems, and they solve them in a variety of ways. This results in extremely varied language throughout the middle parts of conversations, making it hard for AI models to predict what to say at the right time.
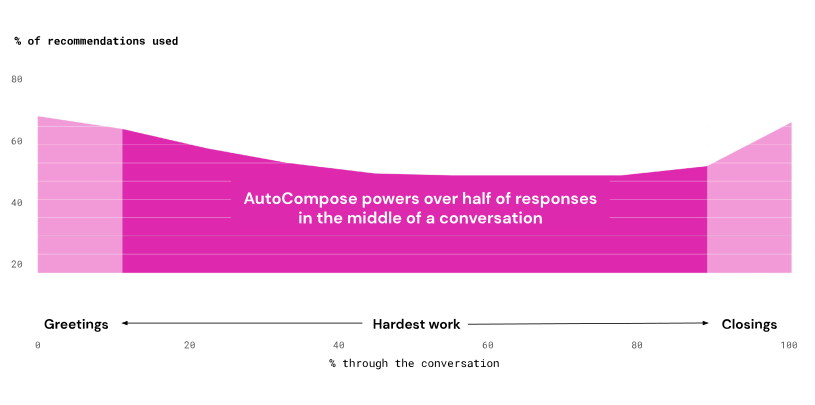
ASAPP’s research delivers the biggest improvements
Whole interaction models are exactly what the research team at ASAPP specializes in developing. And it’s the reason that AutoCompose is so effective. If we look at AutoCompose usage rates throughout a conversation, we see that while there is a higher usage at the beginnings and endings of conversations, AutoCompose still automates over half of agent responses in between.
The low response times in the middle of conversations are where we see the biggest improvements in agent response time. It’s also where the biggest opportunities for improvements are realized.
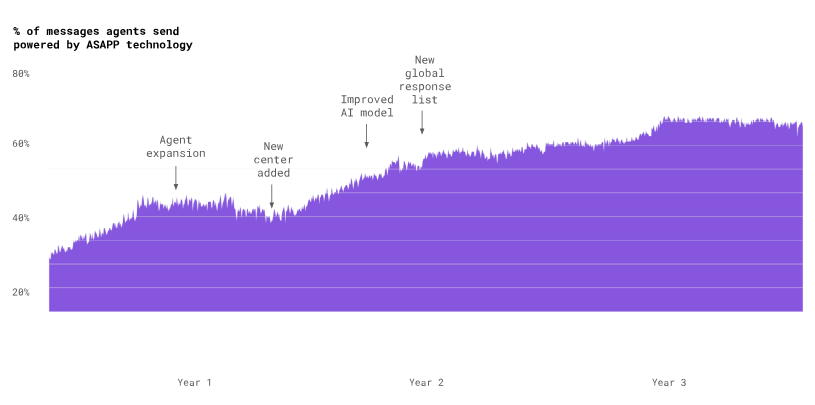
Whole interaction automation shows widespread improvements
ASAPP’s current automated response rate is about 80%. It has taken a lot of model improvements, new features, and user-tested designs to get there. But now agents effectively use our automated responses in the messaging platform to reduce handle times by 25%, enabling an additional 15% of agent concurrency, for a combined improvement in throughput of 53%. The AI model continues to get better with use, improving suggestions, and becoming more useful to agents and customers.
Why AHT isn’t the right measure in an asynchronous and multi-channel world
Operations teams have been using agent handle time (AHT) to measure agent efficiency, manage workforce, and plan operation budgets for decades. However, customers have been increasingly demonstrating they’d prefer to communicate asynchronously—meaning they can interact with agents when it is convenient for them, taking a pause in the conversation and seamlessly resuming minutes or hours later, as they do when multitasking, handling interruptions, and messaging with family and friends.
In this new asynchronous environment, AHT is an inappropriate measure of how long it takes agents to handle a customer’s issue: it overstates the amount of time an agent spends working with a customer. Rather, we consider agent throughput as a better measure of agent efficiency. Throughput is the number of issues an agent handles over some period of time (e.g. 10 issues per hour) and is a better metric for operations planning.
One common strategy for increasing throughput is to merely give agents more issues to handle at once, which we call concurrency. However, attempts to increase throughput by simply increasing an agent’s concurrency without giving them better tools to handle multiple issues at once are short-sighted. Issues that escalate to agents are complex and require significant cognitive load, as “easier” issues have typically already been automated.
Therefore, naively increasing agent concurrency without cognitive load consideration often results in adverse effects on agent throughput, frustrated customers who want faster response times, and agents who burn out quickly.
The ASAPP solution to this is to use an AI-powered flexible concurrency model. A machine learning model measures and forecasts the cognitive demand on agents and dynamically increases concurrency in an effective way. This model considers several factors including customer behaviors, the complexities of issues, and expected work required to resolve the issue to determine an agent’s concurrency capacity at a given point in time.
We’re able to increase throughput by reducing demands on the agent’s time and cognitive load, resulting in agents more efficiently handling conversations, while elevating the customer experience.
Measuring throughput
In equation form, throughput is the inverse of agent handle time (AHT) multiplied by the number of issues an agent can concurrently handle at once.

For example, if it on average takes an agent half an hour to handle an issue, and she handles two issues concurrently, then her throughput would be 4 issues per hour.

The equation shows two obvious ways to increase throughput:
- Reduce the time it takes to handle each individual issue (reduce the AHT); and
- Increase the number of issues an agent can concurrently handle.
At ASAPP, we think about these two approaches to increasing throughput, particularly as customers move to adopt more asynchronous communication.

AHT as a metric is only applicable when the agent handles one contact at a time—and it’s completed end-to-end in one session. It doesn’t take into account concurrent digital interactions, nor asynchronous interactions.
Heather Reed, PhD
Reducing AHT
The first piece of the throughput-maximization problem entails identifying, quantifying, and reducing the time and effort required for agents to perform the tasks to solve a customer issue.
We think of the total work performed by an agent as both a function of the cognitive load (CL) and the time required to perform a task. This definition of work is analogous to the definition of work in physics, where Work = (Load applied to an object) X (Distance to move the object).
The agents’ cognitive load during the conversations (visualized by the height of the black curve and the intensity of the green bar) are affected by:
- crafting messages to the customer;
- looking up external information for the customer;
- performing work on behalf of the customer;
- context switching among multiple customers; etc.
The total work performed is the area under the curve, which can be reduced by decreasing the effort (CL) and time to perform tasks. We can compute the average across the interaction—a flat line—and in a synchronous environment, that can be very accurate.
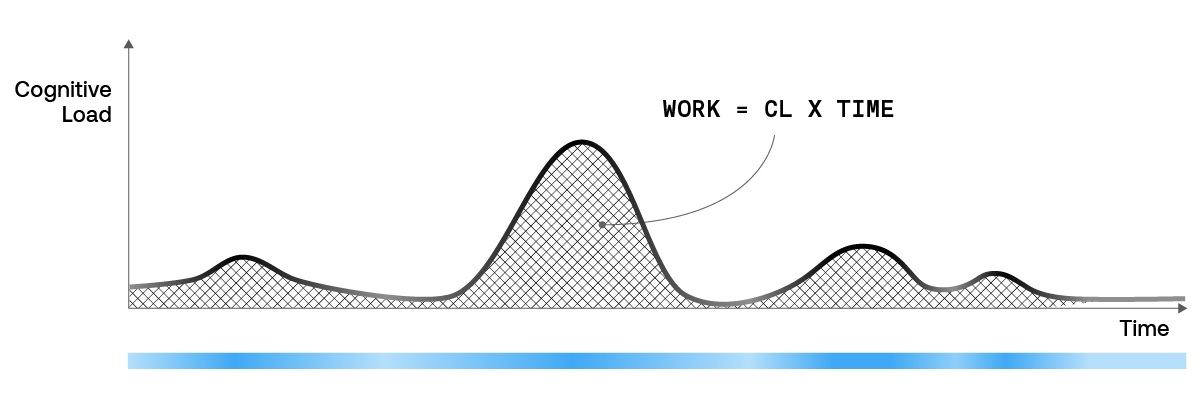
ASAPP automation and agent augmentation features are designed to both reduce handling time and reduce the agents’ cognitive load—the amount of energy it takes to solve a customers’ problem or upsell a prospect. For example Autosuggest provides message recommendations that contain relevant customer information, saving agents the time and effort they would need to spend looking up information about customers (e.g. their bill amount) as well as the time spent physically crafting the message.
For synchronous conversations, that means each call is less tiring. For asynchronous conversations, that means agents can handle an increasing number of issues without corresponding increases in stress.
In some cases, we can completely eliminate the cognitive load from a part of a conversation. Our auto-pilot feature enables automation of entire portions of the interaction—for example, collecting customer’s device information, freeing up agents’ attention.

The result of use of multiple augmentation features during an issue is the reduction of overall AHT as well as reduction of work.
When the customer is asynchronous, the majority of the agent’s time would be spent waiting for the customer to respond. This is not an effective use of the agent’s time, which brings us to the second piece of the throughput-maximization problem.
Increasing concurrency
We can improve agent throughput by increasing concurrency. Unfortunately, this is more complex than simply increasing the number of issues assigned to an agent at once. Issues that escalate to agents are complex and emotive, as customers typically get basic needs met through self-service or automation. If an agent’s concurrency is increased without forecasting workload, then increasing concurrency will actually have an adverse effect on the AHT of individual issues.
If increasing concurrency results in increased AHT, then the impact on overall throughput can be negative. What’s more customers can become frustrated at the lack of response from the agent and bounce to other support channels, or worse—consider switching providers; and agents may feel overwhelmed and risk burning out or churning out
Flexible concurrency
We can alleviate this problem with flexible concurrency: an AI-driven approach to this problem. A machine learning model keeps track of the work the agent is doing, and dynamically increases an agent’s concurrency to keep the cognitive load manageable.
Combined with ASAPP augmentation features, our flexible concurrency model can safely increase an agent’s concurrency, enabling higher throughput and increased agent efficiency.

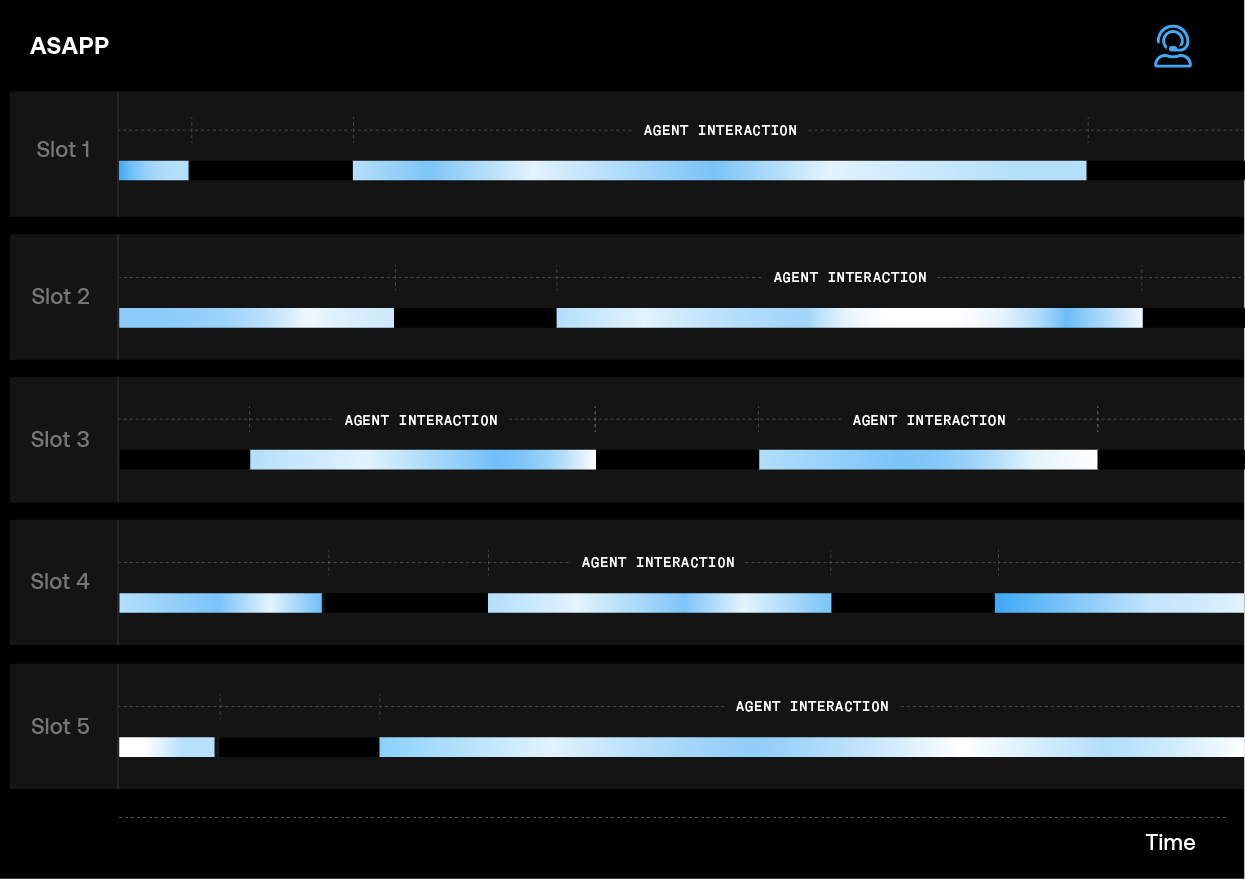
In summary
As customers increasingly prefer to interact asynchronously, AHT becomes less appropriate for operations planning. Throughput (the number of issues within a time period) is a better metric to measure agent efficiency and manage workforce and operations budgets. ASAPP AI-driven agent augmentation paired with a flexible concurrency model enables our customers to safely increase agent throughput while maintaining manageable agent workload—and still deliver an exceptional customer experience.
Does AI really improve agent efficiency?
At ASAPP we develop AI-driven platform features to improve agent efficiency. To be data-driven about feature development, we require a way to measure how well we’re performing. If we introduce something new (like a new feature), we want to know how that feature impacts agent efficiency so that we can learn from it to drive the next features. Because of the complex nature of confounding effects on both 1) the features we wish to analyze as well as 2) the response KPIs of interest, we require specialized techniques to disentangle these confounding effects to properly measure the impact of our features, which is described in this post.
We can think of agent efficiency as throughput of customer issues: the number of issues per agent per time. We can improve throughput both by decreasing agent handle time (AHT) for a single issue and by increasing concurrency (the number of issues an agents handles at the same time). This post specifically discusses how we measure AHT improvement gains enabled by agent augmentation and automation features in digital messaging application.

Calculating how much augmentation improves AHT
AHT improvement should not be measured by usage of a feature alone. Usage of a feature is a great signal about whether agents find our features useful to do their jobs (otherwise we would expect them not to use it). We can think of AHT improvement as the multiplication of both feature usage AND the feature’s impact on AHT when it is used.

Usage is easy to measure: we have data events every time an agent clicks on a suggestion. Those events are aggregated nicely into tables that we can consume. And then we can plot the average daily augmentation rates over time, as in the graph below

But measuring impact is more difficult because there are other factors that contribute to AHT. For example, some types of customer issues naturally take longer to resolve than others (such as troubleshooting, where much of the handle time is waiting for devices to restart). If those types of issues happen to have a lower augmentation rate than other types of issues, it would be unfair to say that augmentation causes longer AHT, when it is moreso the content of the issue that contributes to longer AHT.

As use of agent augmentation features increase handle time decreases—and agents can manage concurrent conversations. This results in higher throughput.
Heather Reed, PhD
Measuring impact
While randomized experiments are the gold standard for measuring the causal effect of a treatment (e.g. a feature) on KPIs of interest (e.g. AHT), observational studies also enable measurement of feature impact without requiring experimentation. This becomes tricky because there are confounding factors that may impact both:
- Agents’ likelihood of using augmentation (e.g. some customer issues are more augmentable than others); and
- AHT (e.g. seasoned agents may be “naturally” faster at solving customer problems).
We can build a causal, statistical model to account for these confounding factors, constructed in such a way that we are able to isolate the impact of the variable that we are interested in (i.e. augmentation). We solve this problem by using random effects regression analysis.
In regression analysis, the goal is to fit regression parameters that describe the relationship between features and responses of interest. In random effects modeling, the regression parameters vary by group (e.g. by individual agent, types of issues, etc.), and the model accounts for both the average group effect (i.e. for all agents) as well as the offset between each agent’s effect and the group average. Random effects models are beneficial when the data exhibits a clustered structure and thus the data are not independent observations. The benefit of the random effects model is that in the absence of a lot of data for a value in the group (e.g. a new agent), the model shrinks the estimate for that agent toward the group average.
The schematic below demonstrates the concept of a random effects model where there is a group-varying intercept and slope. The black line represents the average effect for the group (i.e. all agents), and the pink and blue lines are the offsets from the group average that correspond to specific agents.

When constructing the statistical model, we consider the clustered and/or hierarchical nature of the data. Then, we fit this regression model with production data to learn what these model coefficients are (or more accurately, we learn the posterior distributions of these coefficients).
We then use this trained model to infer insights about augmentation. We do this by using the model to answer the counterfactual question, “What would the AHT have been, if there had been no agent augmentation?”. The difference between this potential AHT outcome and the real AHT provides an estimate of the AHT savings. We can take statistics across all issues (and subsets of issues) to learn insights or assess agent efficiency (proxied as AHT savings) over time, like the blue curve below.

Using these approaches, we’re able to measure how much handle time our augmentation features save. The blue curve in the plot above shows a 100% increase in AHT savings (15% to 30%) over a period of 8 months. This is the result of both measuring the impact of new features as well as increasing usage of features that are driving increased impact (the green curve above). In this way we can quantify the value we deliver for our customers with current features and our Product team can use this insight to develop new features.