Theresa Liao
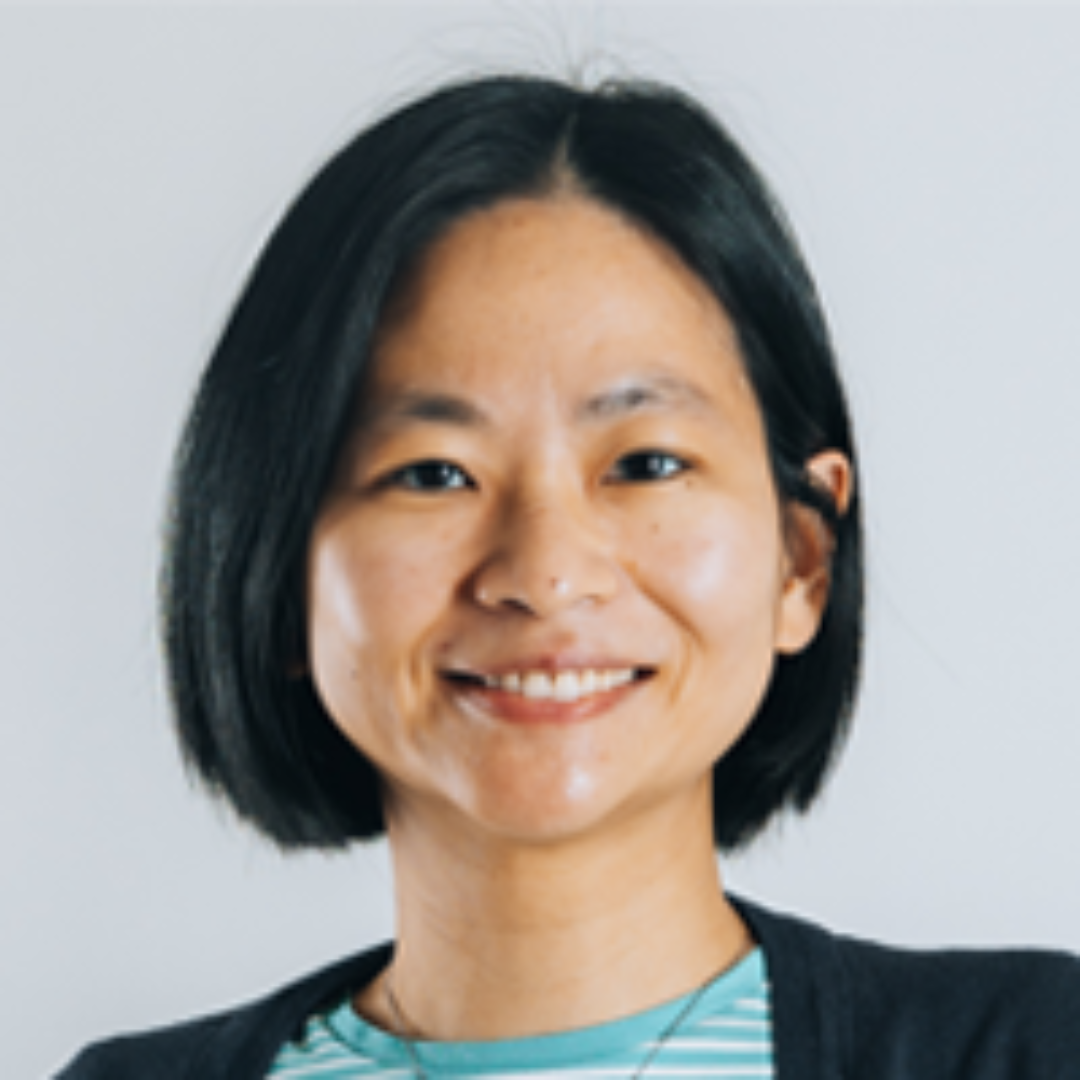
Theresa Liao leads initiatives to shape content and design at ASAPP. With over 15 years of experience managing digital marketing and design projects, she works closely with cross-functional teams to create content that helps enterprise clients transform their customer experience using generative AI. Theresa is committed to bridging the gap between complex knowledge and accessible digital information, drawing on her experience collaborating with researchers to make technical concepts clear and actionable.
Why wait? JetBlue’s blueprint for leading AI-driven CX transformation
What if the biggest obstacle to improving customer service isn’t technology, but the fear of jumping in before you're fully ready? In this final installment of our three-part series on JetBlue’s approach to generative AI in its contact center, Shelly Griessel, VP of Customer Support, shares her team's forward-thinking strategy for customer support and explores the realities of deploying ASAPP’s GenerativeAgent (JetBlue’s Amelia 2.0). Her message is clear: don’t wait for the perfect conditions to start — the time to act is now, or risk falling behind, especially from a cost perspective.
You can also watch the full discussion. [link to full Wistia video].
Read Part 1, JetBlue’s CX journey: tackling challenges in an evolving industry.
Read Part 2, How JetBlue aligns costs, culture, and AI for CX success.
* Minor edits have been made to the transcript for clarity and readability.
Embracing generative AI to boost resolution and satisfaction
Dan: The way ASAPP thinks about it is that we're trying to build is something that helps improve the performance of agents, but also candidly to reduce the number of agents or labor hours or tier one interactions, whatever term you're using.
When you and I were speaking, you put it into a similar construct. And when you're thinking about AI, you're thinking about tech. You're looking at how I can improve and accelerate the performance of my crew members (JetBlue’s contact center agents), and how to reduce the pizza pie, so to speak, of the number of agents.
So take us through that. Because you are partnered with ASAPP, you’re using us for digital, for chat essentially, and live agent interactions all through digital. And then you've just recently deployed GenerativeAgent, or Amelia.
Take us through that journey of how you're improving the performance of an agent or accelerating the performance. And then you've introduced GenerativeAgent, or Amelia 2.0 recently.
Shelly: So the plan has been all along that we have to make the pizza pie smaller because that's how you bring costs down. We have to bring volume down. You have one shot at getting it right because if you don't get it right, then the customer will call back again and again. I mean, I don't know about your industries, but when a customer is not happy in an airline situation, they will call you back six, seven, eight, nine, ten times.
And that wastes money. So, the idea has always been that first contact resolution is a big deal for us, followed by CSAT.
I will never say we don't care about handle time, but we manage handle time as a separate entity altogether. If we are able to just shrink the pie by making the crew members more effective, we can push more of the really simple stuff to Amelia, and she will deal with it. I think now that we've got generative AI going, we really want to accelerate what she's able to do, and to have more of the bigger conversations with customers.
Understanding customer intents to optimize support
Shelly: I don't believe that Amelia should have the personality of being super empathetic because everybody knows she's a bot. So you have to be very careful that it still remains authentic, and she's not gonna ever be super authentic.
I think that the customer wants to get the job done as fast as possible, and get the right resolution that they're looking for. So we have to just keep on looking at understanding why customers are contacting us, and ASAPP has done an amazing job for us to explain the intent of our customers.
Once you understand that better, you can actually start looking at your product and say we need to make changes in the product. Why do they keep on calling about baggage? They don't like the baggage policy? Or checking in? They don't like that policy?
ASAPP has helped us a lot to understand the intents of why customers are contacting us. But that's all technology that is helping us shrink the pie.
Nobody, no company, wants to pay tens of or hundreds of millions for customer support. They don't. They want to invest the money in brand-new aircraft, and so they should.
We have an obligation to get a whole lot smarter about it. So our strategy is very much constantly evaluating our tech stack. Is it what it's what it's still needed? Do we provide them with enough information to be able to do the job? Like guided call flows. And making sure that crew members understand this is how it's going to help you versus anything else.
From proof of concept to progress: Teaching GenerativeAgent
Dan: I was thinking about this as you were speaking. I saw some great research. Shout out to Brian and Brooke from CMP on the research. I saw in a session yesterday around chatbots and voice bots just some dissatisfaction with customers and etcetera.
Everybody's familiar with that. When you dipped your toe into GenerativeAgent, or Amelia 2.0, what were concerns that you had going in? Because chatbots and voice bots promised a lot of the same things that you're hearing from a generative AI agent. And so what we hear a lot of is skepticism because we promised a lot, and it didn't necessarily happen.
So when you approached generative AI, how did you approach that to go, I'm going to see if GenerativeAgent, or Amelia 2.0, can actually work? And then tell us about the journey, trepidation, results, anything that you would wanna share about that.
Shelly: So we started in May when we said, okay, let's do a POC (proof of concept), and let's see how it goes.
And we had a team watching it and course correcting. I think you're familiar with the term hallucination. So she comes up with things that you go, why did you say that, Amelia? That's not true.
And then it's a matter of, okay, let's pull her back. Let's teach her how to do this differently. And I think that we've got enough – so this started in May. At that time, our containment with her was at about 9%. And then by August, she went up to as high as 21%.
And that's amazing in a very, very short period of time, and it's just a proof of concept. So it's very little volume that we're giving her, but I think that we now need to double down on this. I want to fast-track teaching her. I think that this has to come from taking some of our best crew members in the company and watching her and saying, “No, take that option away.” So there are certain things, for instance, that we learned that we don't want her to do.
There’s so much pressure on airlines at the moment to get your refund policies right. So the DOT is all over us. We can't let her make decisions on refunds. So we say, okay. Put that out of scope. What else is a hot topic? Like ADA, hot topic. Wheelchairs, hot topic. You have to keep that stuff out.
And I think that it's just going to take a little bit of time blending humans with teaching her on the areas that she can absolutely start knocking out of the park, and we'll get there. I think it just has to be this relationship made between humans and Amelia to learn.
I think that some of the companies that are getting some good success with it are taking a bot, whatever bot they have, and let the bot learn from a human. So I think that matching what great crewmembers can do with the bot is for us looking like that's going to be the future.
Start now even if you are not ready – or risk being left behind
Dan: A lot of the questions that we hear at ASAPP are, “I'm not ready for a GenerativeAgent experience because I've got knowledge base issues or technical debt” or any of those things.
If you were to give any advice to this audience about a place to start this journey – for people who are wanting to start on this AI journey but aren't ready to, like, deploy some sort of GenerativeAgent, where could they start? How do you evaluate?
Shelly: Your environment is never going to be right and ready. It's never. I mean, come on. For all of us that have been in customer support areas forever, every year we plan all the things that I'm going to do. And before you know, it's the end of the year. And I didn't do 50% of it because why? Because we come in and there's a new drama.
I think that the time is never right. I think that for this, in my mind, you have to jump in because I think if you don't, you're going to be left so far behind, especially from a cost perspective.
I don't think it's just airlines that are under the pressure for forecasts at the moment. We're going through budgets right now, and it's tough. Everybody wants customer support to cost nothing, and please make sure the customer is really happy still.
Don't spend any money, but don't you dare let that NPS go down.
I think we all know that, and that's why I say you've got to jump in and say, what have you got to lose? If you put the boundaries around it, what have you got to lose? You really don't.
So I don't think that you have the luxury of waiting for everything to be ready and perfect. You have to go, okay, I'm ready now. Now I can do it.
I think you're gonna be left behind if you do.
Read Part 1, JetBlue’s CX journey: tackling challenges in an evolving industry.
Read Part 2, How JetBlue aligns costs, culture, and AI for CX success.
How JetBlue aligns costs, culture, and AI for CX success
In part two of our blog series on JetBlue’s approach to customer service, we dive into the financial and labor dynamics that shape the airline’s contact center operations. Shelly Griessel, VP of Customer Support at JetBlue, offers a candid look at the factors driving up costs, from first-contact resolution challenges to the role of AI in improving efficiency. But it’s not just about numbers—JetBlue is deeply committed to supporting their workforce, investing in training, and fostering a culture where employees feel valued and empowered. In this post, we’ll explore how GenerativeAgent, what JetBlue calls Amelia 2.0, is not just a tool for improving customer interactions, but a vital partner in alleviating burnout and keeping agents engaged.
Read Part 1, JetBlue’s CX journey: tackling challenges in an evolving industry.
Read Part 3, Why wait? JetBlue’s blueprint for leading AI-driven CX transformation.
* Minor edits have been made to the transcript for clarity and readability.
Understanding Cost Drivers in Customer Service
Dan: On cost reduction in the contact center, some will say, “okay, we're trying to reduce or maintain costs.” When you think of the financial aspect that either you or your peers are seeing, what is driving up that cost? Any insight that you have on what's affecting that?
Shelly: What's driving up the cost is that if you don't keep FCR at the highest possible level, customers will keep on calling back. And more volume means the cost of calls goes up.
So you have to try and find a way of doing two things in the customer contact center environment going forward. For us, it is bringing down the cost per call, and a big part of that is containment through conventional chat or now with more progressive, what we call Amelia 2.0 (powered by ASAPP’s GenerativeAgent).
We call our bot Amelia (after Amelia Earhart) because we had to give her a name.
We really like her. She shows up every day. She's got no absenteeism problems, never wants PTO, and she just shows up every day, and she's always friendly. She's, like, always friendly.
The Role of AI in Enhancing Customer Interactions
Shelly: So now we've got Amelia 2.0, which is gen AI, and she's a little bit more conversational, sometimes too much, but we're getting her there.
And I think that that is the next evolution.
We have to free crew members (JetBlue’s contact center agents) from having to deal with very basic stuff, and frankly, they get bored with it.
Our tenure is extremely long at JetBlue. The average tenure is about ten and a half years; they don't leave. But also the majority of our crew members are part-time.
So they work anything between fifteen and thirty hours a week. So that also helps with the lack of burnout. They don't burn out, and that's why.
But gen AI has got a massive role to play in this. It has a massive role to play in it.
Dan: When you're approaching the big topic of AI – something that’s so ubiquitous now that it's become very generic and losing a lot of its meaning and power – how are you and the team approaching those waters in the contact center space? What are the concerns and the outcomes that you're trying to get?
Shelly: So when we started really ramping up AI, there was obviously a massive fear by our crew members about “it's gonna take my job away.” And that was a very real fear for them.
And then they started realizing that she (Amelia 2.0) actually covers the shifts that they don't want to do. So Amelia became very handy. She would work weekends, and she would work through the night. So from that perspective, it became less of a threat for them because they knew that she was complementing them.
And our containment is extremely high. We started two years ago in the low 30s – 35, 36% containment. And now we're sitting at between 68% and 70% containment.
It just never gets past Amelia. Amelia keeps it. But when a call actually eventually comes over to the crew member, all the hard work has kind of been done already, and they can step in and just start making decisions that the customer is looking to be made. So for us, we've embraced AI as a company. Crew members are still more afraid of “will a BPO take over my job?” They're all more afraid of that than they are of AI now.
Addressing Labor Concerns in the Age of AI
Dan: When we talk about how agents are gonna lose jobs because of AI, I'm wondering why we aren't talking more about how agents are removing themselves from the job themselves and in record numbers. I think we are at 52-62% average turnover, major absenteeism, and the majority of contact center leaders are saying they are having a hard time recruiting.
I'm really interested in your thoughts on AI when it comes to this big issue of labor in the contact center and the high cost and absenteeism, and how contact centers are just dealing with that.
Shelly: We are proud of the fact that we don't lose people. But I think it's got a lot to do with the part-time model that we run. In fairness, there's a massive burnout level.
If you take call after call after call - and customers, let's be honest, they don't call in to say, ”way to go,” “I had a great flight,” “my flight was on time.” That's a given.
They don't. I mean, so every single call, call after call after call.
So we obviously manage it through the fact that they don't have these 40-hour week shifts, and they work part-time, so it makes for life to be a whole lot easier. But we also have a responsibility – and we spend a lot of time on culture. So we double down on culture. We watch our ratios of crew members to supervisors.
Maintaining Relevance in a Changing Workforce
Shelly: I was sharing with you earlier how much time we spend with crew members – what we call the PDRs, which is protecting the direct relationship that we have with them. It's a big deal for us. And we explain to them the why behind BPOs, the why behind Amelia. And the more they understand it, the better.
But the other obligation that we have in this industry is to make crew members or agents, wherever they are, relevant in five years from now because they won't be relevant if we don't make the effort. It's kind of our responsibility to do it.
And we have to teach them different scenarios of how to deal with de-escalation or really complicated problems. We have to, and that is where technology comes into it. Technology becomes their friend.
So I think we have a massive obligation and a responsibility to keep them relevant in the new world, and the communication has to be so big and so wide open to bring them along.
The worst thing that we can do is to make them feel disenchanted and they stay. Because if they stay, your customers will feel the impact of their unhappiness.
You've got an obligation. Employee engagement scores are huge in JetBlue. It's very, very big.
So we spend a lot of time on that, but I think that embracing technology as a part of it, we've never shied away from the fact that Amelia is there and this is what she does. These were her stats. We give them her stats on a daily basis because she's part of the team.
It's weird. She's not really a person, you know that.
Dan: Amelia is ASAPP's GenerativeAgent, but when she talks about it as Amelia, I'm like, yeah, she's part of the team. This nice woman in the front row said, “I wanna be Amelia's friend now.”
Fostering a Valued Workforce Culture
Dan: So, to put a finer point on it, because we are gonna talk about the tech part of this and the partnership with ASAPP later. Can you touch on what you are doing in the culture that you think, “this is going to have a really appreciable effect on keeping the crew members in the job?”
Shelly: People really want to feel they’re valued in the world. The feedback we constantly get from our crew members is that this is the first place I have worked at that I don't feel like a number. I'm not a number.
They've got access, and when we say they've got access to me as the VP, that is not just empty talk. It's real.
They really do have access. Every leader in my team has to dedicate two hours a week in their calendar for any crew member to talk to them about anything. Anything. And they blocked up those views.
We have monthly what we call "directly to you” meetings, in which it's open kimono. We tell them all the good and all the bad. The company is not doing well. The company is doing well. This is where we're going. Thhis is the good and the bad.
We've got a CEO that absolutely believes in 100% transparency. There's absolutely no point in sugarcoating anything. You have to be very honest with people.
And that's how we bring 24,000 people along with us. That is the total number of crew members that we've got in the company, but that's how we bring them along and how we protect the culture. So for the customer contact center area, we are not unionized, and there's no talk of being unionized because people know that things get done faster when they come to Shelley directly or their director or their manager to say, “I'm really not happy about this.” “What are we gonna do about it?” “Oh, well, this is why I can't do anything about it.”
So we absolutely believe in a very transparent relationship with them. We tell them the good and the bad all the time.
Read Part 1, JetBlue’s CX journey: tackling challenges in an evolving industry.
Read Part 3, Why wait? JetBlue’s blueprint for leading AI-driven CX transformation.
JetBlue’s CX journey: tackling challenges in an evolving industry
Customer support is not just about answering customer questions—it's also about resolving customer issues quickly, and adapting to rapidly changing expectations. JetBlue has been on the forefront of this shift, with a model that’s built on 25 years of remote work and a deep commitment to empowering their agents. In this post, part one of a three-part blog series based on a discussion between Dan Rood, SVP Marketing at ASAPP, and Shelley Griessel, VP of Customer Support at JetBlue, we explore how the airline’s approach has adapted to the challenges of a rapidly evolving industry, where the unpredictable nature of customer needs and agents’ lives can make every day a unique experience.
Read Part 2, How JetBlue aligns costs, culture, and AI for CX success.
Read Part 3, Why wait? JetBlue’s blueprint for leading AI-driven CX transformation.
* Minor edits have been made to the transcript for clarity and readability.
The unpredictable joys of contact center work
Dan: I'd love to hear from you - what is a moment or moments that occur to you in this industry in your role that you go, “I love this job. I love this.”
Shelly: I think without a doubt, it is the unpredictability.
We all think we've got our week planned out, we've got our day planned out, but it really doesn't work like that. I think that I love, love, love the fact that I've got absolutely no idea what's going to come at me today… And the unpredictability of what's happening in your crew members' lives (that's what we call our agents).
And it makes for just constant entertaining. Sometimes it's heart-wrenching stuff that you have to deal with. So we go permanently from a state of being absolutely ecstatic about life and, like, “we're winning every day” – and then they are just heartbreaking times.
And I think that's what I love most about the job.
I don't think… this is not what I planned to do in life, but just being in contact centers for so long, I think I've been blessed to have experienced so much over the thirty-five years that I've been in the business.
Why contact center challenges persist
Dan: If we were to go back five years or ten years, the conversation in any surveys that we have in a room like this, is that three or four things happen in a contact center – controlling, or even reducing costs, and improving CSAT, are the themes over and over again. And yet, in 2024, there's a lot of evidence to say it's not necessarily getting better. If you were to just step back, why does it feel like it is not getting better?
Shelly: I think to start off with, the last four to five years have made it exponentially harder. There's something that has just clicked in the mind of consumers that they're not tolerating as much.
It feels like it is a lot harder to please. And I think that people are also more than ever before stretched for time. There's just so little tolerance for long hold times, and then they get through to somebody who is not going to wrap it up quick enough.
I think the most precious commodity in today's world is absolutely time.
I am of the belief that they want to get an answer quickly. They want to get a consistent answer. If they don't like it, then they'll phone back three or four or five or six or seven times, until they get the answer that they're looking for. That drives up costs, that drives down FCR.
It's just a lot more complicated, and I think COVID has a lot to do with it. It has also got a lot to do with how much more challenging crew members' lives are. It is harder, and the value of the job hasn't gone up. So people are not necessarily getting paid more, and they're holding down two or three jobs, which makes their lives complicated.
Remote work and customer expectations
Shelly: In the JetBlue model, our contact center crew members work one hundred percent from home, and have since the beginning for 25 years.
In addition to that, as they work from home, they were challenged with, during COVID, being alone and having no contact with anybody else. So it's been a lot worse for them.
But I do think that for customers, it is a lot more about getting through to somebody that can give me an answer really quickly, and let me be on my way. And I want to do it when I want to do it, any time of the day or night. It's just a different experience that we have to offer customers today versus what it was.
Empowering contact center roles for speed and resolution
Dan: At ASAPP, we call it abbreviating customer pain. From a performance standpoint, the main threshold that you're trying to get to is speed and obviously real resolution. Is that the strategy when it comes to keeping brand differentiation with JetBlue versus your competitors?
Shelly: Absolutely. So we do want to speed it up, and we want to make sure that we use the human intellect in a different way than what we probably did three or five years ago. Which means that we are personally investing a lot more in the training and development of people to deal with the really tough issues. It doesn't help to say to a person, “You're empowered, and you can do what you need to do.” You actually have to teach them what empowerment looks like. So there's a lot more time and resources that we're spending on evolving the role of a customer support person.
And I do believe that the footprint of humans in a contact center is going to get smaller, but I think it's gonna finally become a higher paid role, because they're going to deal with a lot more complicated matters – a lot more complicated – and we should pay them more.
So I think the footprint will get smaller, but we should be able to pay them more.
Read Part 2, How JetBlue aligns costs, culture, and AI for CX success.
Read Part 3, Why wait? JetBlue’s blueprint for leading AI-driven CX transformation.
5 questions to ask before building your own AI solution for CX
The build vs. buy debate in software is nothing new. With the rise of large language models (LLMs), spinning up prototypes that can complete tasks has become easier than ever. However, developing an AI application for CX that’s scalable and production-worthy demands extra consideration. In this post, we’ll explore key questions to ask when deciding whether to build or buy generative AI solutions for CX.
While the right answer depends on industry, use case, and business goals, it’s important to carefully weigh your options.
Why building an AI application for CX requires extra consideration
The reason for this has a lot to do with the nature of generative AI.
Unlike most software development where the goal is to create a deterministic system with the same, expected outcome every single time, generative AI generates new information and content based on patterns, often producing varied responses that can be difficult to predict.
This variability is what makes gen AI applications so powerful, but also adds complexities in maintaining consistency and control.
Differing from standard applications where the workflow is predefined, generative AI models require continuous monitoring, training, and refinement. There is a misconception that generative AI just needs instructions. But in fact, they need much more, especially if you are training on your own data.
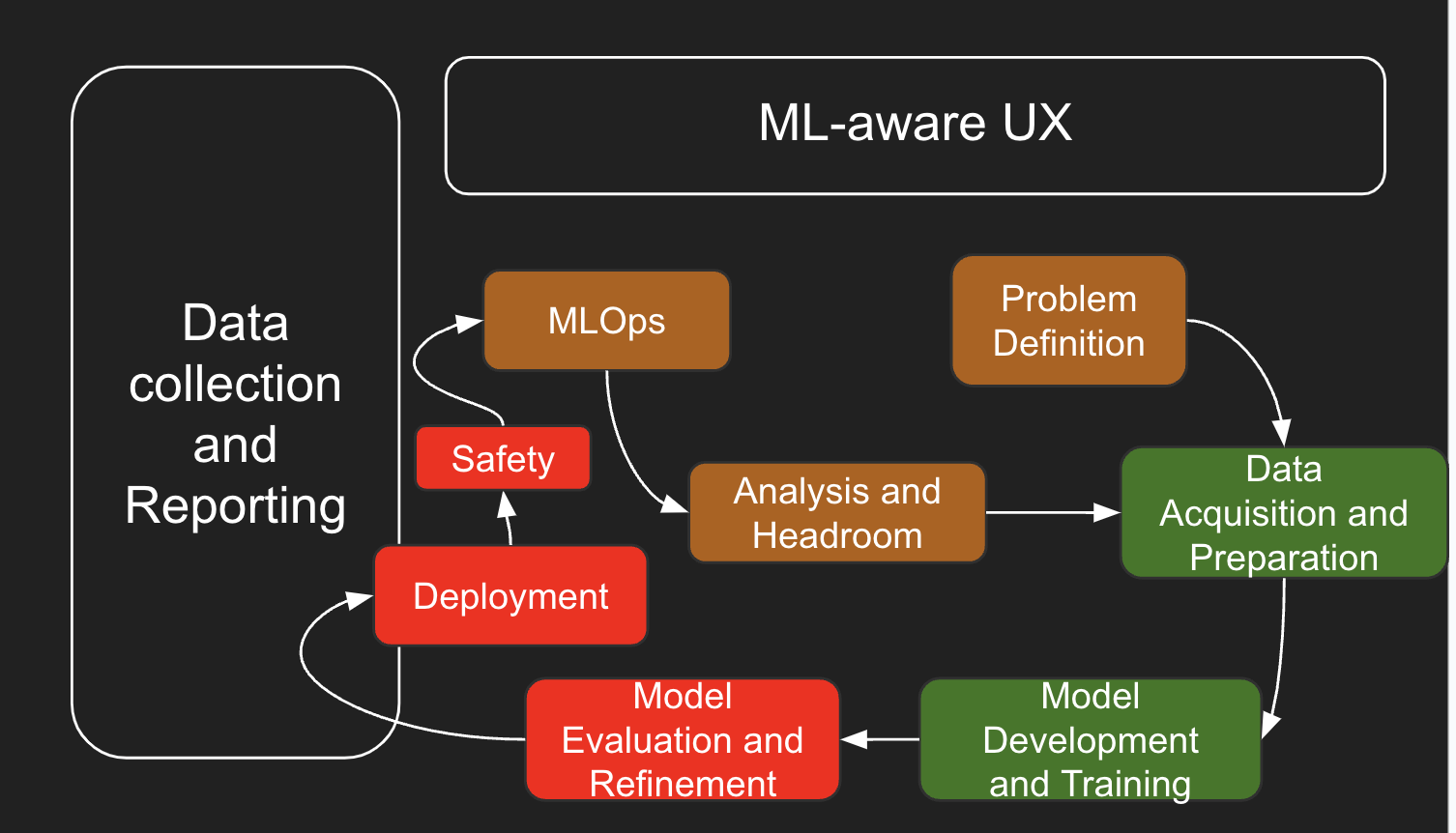
The flexibility makes them ideal for tasks that benefit from creative problem-solving or personalized engagement, but it also means that development doesn’t stop at launch. This, compounded by the fact that CX applications can ultimately impact a brand’s relationship with its customers, brings additional layers of considerations when deciding whether to build the application yourself or to partner with a vendor.
While the following is not an exhaustive list, here are some top questions you should consider when making a decision.
Is building AI applications part of your business objectives?
If building AI applications aligns with your business objectives, you’re likely prepared for both short-term and long-term investment in development and maintenance that will be absolutely critical for AI applications, ensuring the solution doesn’t become a burden on your resources.
In contrast, if developing your own AI applications isn’t a core objective, partnering with a vendor may be the more sensible approach. Vendors are equipped to provide solutions that fit your needs without the complexities of internal development, allowing you to focus on your primary business goals.
Based on McKinsey & Company’s recent estimates, building and maintaining your own foundational model could cost up to 200M with an annual recurring cost of 1-5M. Using off-the-shelf solutions or fine-tuning existing models with sector-specific knowledge can dramatically reduce this cost.
What’s your timeline for deployment?
If your deployment timeline is urgent—perhaps due to a competitor already leveraging AI solutions—working with an AI solution provider can help you hit the ground running and accelerate implementation. A vendor with solid experience in enterprise AI development will help you avoid unnecessary trial and error, minimizing risks associated with AI safety - such as AI hallucinations.
Instead, leaders should strongly consider partnering with gen AI solution providers and enterprise software vendors for solutions that aren’t very complex or [industry] specific. This is particularly critical in instances where any delays in implementation will put them at a disadvantage against competitors already leveraging these services.
- McKinsey & Company, in "How generative AI could revitalize profitability for telcos"
Do you have the internal expertise, resources, and infrastructure to build, scale, monitor, and maintain an AI solution?
While widely available large language models (LLMs) have significantly accelerated the process of building a working prototype on a laptop, this does not equate to having a production-ready, scalable solution that can effectively address your business needs [link to last mile]. Additionally, the demand for ongoing maintenance means that development does not stop after the application is launched.
Developing AI applications requires a skilled team knowledgeable in machine learning, data management, and software engineering, along with the necessary technological resources and datasets. Even choosing the right LLM to use for the best fitted use cases would require a good understanding of how various LLMs differ. Experience working with AI solutions is also crucial for successful deployment in an enterprise context.
In the last two months, people have started to understand that LLMs, open source or not, could have different characteristics, that you can even have smaller ones that work better for specific scenarios
- Docugami CEO Jean Paoli, in CIO “Should you build or buy generative AI”
If your organization lacks this expertise or the infrastructure to support such a project - not just at the prototype stage, but also to scale, monitor, and maintain the solution in the future - it may be more prudent to consider vendor solutions that can provide the capabilities you need without overextending your internal teams.
Also consider, if problems arise, do you have the manpower and expertise to investigate the root cause and implement long-term fixes, or will you be crossing your fingers and hoping the issues don’t recur? A strong internal team capable of addressing challenges as they emerge is necessary for ensuring the reliability and effectiveness of your AI application for CX. Without this capability, you risk operational disruptions and even diminishing trust in your brand.
What ROI do you expect from your AI application? And how comfortable are you with the associated risks?

When considering your expected return from an AI investment, it’s essential to balance potential returns with the associated risks.
Agent-facing projects generally carry lower risks, as the AI solution won’t directly interact with customers. This allows for more trial and error, with agents able to provide feedback on the AI application’s performance. That said, such solutions might yield only incremental gains in agent productivity with less or no impact on the customer experience, and do not take advantage of the full capabilities of gen AI.
In contrast, customer-facing gen AI applications can offer a much better return because they can directly improve customers’ ability to self-service and, in some cases, resolve their issues directly. Here are the kinds of results a customer got when deploying generative AI agents with the capability to resolve Tier-one tasks.
Allowing AI to handle a broader range of tasks introduces added complexity. While there are risks—such as AI hallucination, where the system may generate incorrect or irrelevant responses—these challenges can be managed with the right approach. Having a strong internal team that can tackle this, or choosing an experienced vendor with a well-informed strategy for handling AI behavior, will ensure guardrails are placed around customer-facing interactions — so you can get the most out of your AI applications with confidence.
Making a thoughtful decision
Ultimately, the choice between building or buying an AI solution should align with your organization’s long-term vision. Each option carries its own set of challenges and opportunities, and taking the time to assess your specific needs can set the stage for success.
Considering the evolving landscape of AI, it's not just about deploying technology, but also ensuring that it fits well into your operational framework and readies you for the future. With careful evaluation, you can make a choice that enhances your customer experience. Whether you decide to build or partner, the key is to stay focused on your goals and embrace a strategic approach to generative AI applications.