Stefani Barbero
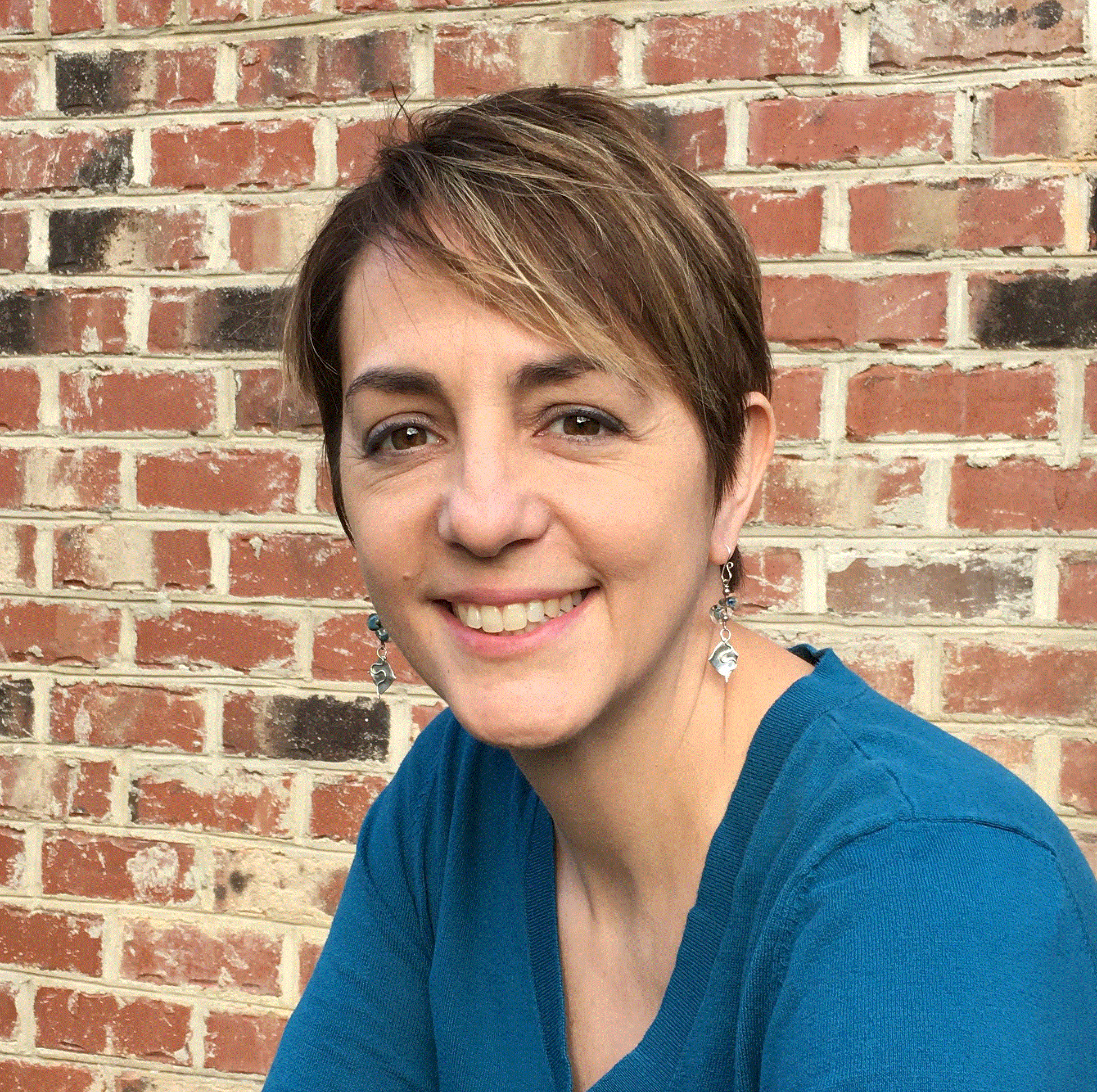
Stefani Barbero is a marketing content writer at ASAPP. She has spent years writing about technical topics, often for a non-technical audience. Prior to joining ASAPP, she brought her content creation skills to a wide range of roles, from marketing to training and user documentation.
A New Era of Unprecedented Capacity in the Contact Center
Ever heard the phrase, "Customer service is broken?"
It's melodramatic, right? —something a Southern lawyer might declaim with a raised finger. Regardless, there’s some truth to it, and the reason is a deadly combination of interaction volume and staffing issues. Too many inbound interactions, too few people to handle them. The demands of scale do, in fact, break customer service.
This challenge of scaling up is a natural phenomenon. You find it everywhere, from customer service to pizza parlors.
Too much appetite, too little dough
If you want to scale a pizza, you have to stretch the dough, but you can't stretch it infinitely. There’s a limit. Stretch it too far, and it breaks.
Customer service isn't exactly physical, but physical beings deliver it— the kind who have bad days, sickness, and fatigue. When you stretch physical things too far (like balloons, hamstrings, or contact center agents), they break. In contact centers, broken agents lead to broken customer service.
Contact centers are currently stretched pretty thin. Sixty-three percent of them face staffing shortages. Why are they struggling? Some cite rising churn rates year after year. Others note shrinking agent workforces in North America and Europe. While workers flee agent jobs for coding classes, pastry school, and duck farming, customer request volumes are up. In 2022, McKinsey reported that 61% of customer care leaders claimed a growth in total calls.
To put it in pizza terms (because why not?), your agent dough ball is shrinking even as your customers' insatiable pizza appetite expands.
What’s a contact center to do? There are two predominant strategies right now:
- reduce request volumes (shrink the appetite)
- stretch your contact center’s service capacity (expand the dough)
Contact centers seem intent on squeezing more out of their digital self-service capabilities in an attempt to contain interactions and shrink agent queues. At the same time, they’re feverishly investing in technology to expand capacity with performance management, process automation and real-time agent support.
But even with both of these strategies working at full force, contact centers are struggling to keep up. Interaction volume continues to increase, while agent turnover carries on unabated. Too much appetite. Not enough dough to go around.
How do we make more dough?
Here’s the harsh reality – interaction volume isn’t going to slow down. Customers will always need support and service, and traditional self-service options can’t handle the scope and complexity of their needs. We’ll never reduce the appetite for customer service enough to solve the problem.
We need more dough. And that means we need to understand the recipe for customer service and find a way to scale it. The recipe is no secret. It’s what your best agents do every day:
- Listen to the customer
- Understand their needs
- Propose helpful solutions
- Take action to resolve the issue
The real question is, how do we scale the recipe up when staffing is already a serious challenge?
Scaling up the recipe for customer service
We need to scale up capacity in the contact center without scaling up the workforce. Until recently, that idea was little more than a pipe dream. But the emergence of generative AI agents has created new opportunities to solve the long-running problem of agent attrition and its impact on CX.
Generative AI agents are a perfect match for the task. Like your best human agents, they can and should listen, understand, propose solutions, and take action to resolve customers’ issues. When you combine these foundational capabilities into a generative AI agent to automate customer interactions, you expand your contact center’s capacity – without having to hire additional agents.
Here’s how generative AI tools can and should help you scale up the recipe for customer service:
- Generative AI should listen to the customer
Great customer service starts with listening. Your best agents engage in active listening to ensure that they take in every word the customer is saying. Transcription solutions powered by generative AI should do the same. The most advanced solutions combine speed and exceptional accuracy to capture conversations in the moment, even in challenging acoustic environments.
- Generative AI should understand the customer’s needs
Your best agents figure out what the customer wants by listening and interpreting what the customer says. An insights and summarization solution powered by generative AI can also determine customer intent, needs, and sentiment. The best ones don’t wait until after the conversation to generate the summary and related data. They do it in real time.
- Generative AI should propose helpful solutions
With effective listening and understanding capabilities in place, generative AI can provide real-time contextual guidance for agents. Throughout a customer interaction, agents perform a wide range of tasks – listening to the customer, interpreting their needs, accessing relevant information, problem-solving, and crafting responses that move the interaction toward resolution. It’s a lot to juggle. Generative AI that proposes helpful solutions at the right time can ease both the cognitive load and manual typing burden on agents, allowing them to focus more effectively on the customer.
- Generative AI should take action to resolve customers’ issues
This is where generative AI combines all of its capabilities to improve customer service. It can integrate the ingredients of customer care—listening, understanding, and proposing—to safely and autonomously act on complex customer interactions. More than a conversational bot, it can resolve customers’ issues by proposing and executing the right actions, and autonomously determining which backend systems to use to retrieve information and securely perform issue-resolving tasks.
Service with a stretch: Expanding your ball of dough
Many contact centers are already using generative AI to listen, understand, and propose. But it’s generative AI’s ability to take action based on those other qualities that dramatically stretches contact center capacity (without breaking your agents).
A growing number of brands have already rolled out fully capable generative AI agents that handle Tier 1 customer interactions autonomously from start to finish. That does more than augment your agents’ capabilities or increase efficiency in your workflows. It expands your frontline team without the endless drain of recruiting, onboarding, and training caused by high agent turnover.
A single generative AI agent can handle multiple interactions at the same time. And when paired with a human agent who provides direct oversight, a generative AI agent can achieve one-to-many concurrency even with voice interactions. So when inbound volume spikes, your generative AI agent scales up to handle it.
More dough. More capacity. All without stretching your employees to the breaking point. For contact center leaders, that really might be as good as pizza for life.
Want more? Read our eBook on the impact of agent churn
Agent churn rates are historically high, and the problem persists no matter what we throw at it — greater schedule flexibility, gamified performance dashboards, and even higher pay.
Instead of incremental changes to timeworn tools, what if we could bypass the problem altogether?
Download the Agent Churn: Go Through It or Around It? eBook to learn why traditional strategies for agent retention aren't working, and how generative AI enables a radical new paradigm.