How anomaly detection helps you handle the unexpected
Every day, ASAPP customers handle tens of thousands of inquiries to their contact centers. We have a pretty solid understanding of what the majority of these requests are about. Most are routine issues that agents have experience dealing with. However, on any given day, there is the risk that a customer may be slammed with hundreds or thousands of calls about something out of the ordinary. These events might range from a popular pay-per-view fight, to service outages, to a UI change confusing half the user base. On those days, ASAPP anomaly detection is there to help.
Every conversation that comes into a content center and enters our infrastructure contains a problem statement somewhere within. Problem statements help direct agents towards the caller’s needs, and help contact center managers understand broader analytics for key traffic drivers. In messaging channels, the user is asked to input their problem directly. For voice channels, ASAPP speech-to-text transcription feeds an NLP extraction model that pulls the problem statement out.
We’ve seen a wide variety of problem statements over the years. Most of the time, our augmentation methods help agents swiftly resolve standard issues. But what happens when something totally new appears? When the unexpected causes an influx of inquiries about an unfamiliar issue? How do we quickly identify this new behavior and extract the conversations so our customers can more effectively address them? How do we know if our system is recognizing the right changes in language? And how do we measure the impact of these new behaviors?
The answer we found was to train a model to distinguish the problem statements coming in now from those that have appeared before, comparing the current stream of requests to the history of past requests. On an average day, the data stream coming in looks much like the historical data, full of similar inquiries about common issues. This makes it difficult to train a model to differentiate the current stream of data from the past.
But on an interesting day, the language in our data stream looks significantly different, containing words or phrases that don’t normally appear.
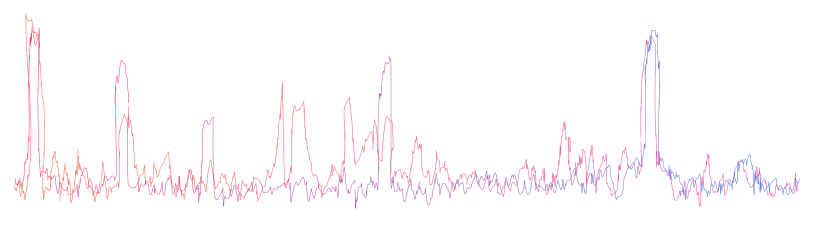
This is where our trained model becomes highly confident it can distinguish the current stream of data from our historical record. Moreover, on these days, the new problem statements typically reflect a single shared issue and include similar language on that topic. This helps explain exactly what issue caused users to hammer a customer’s contact center with traffic in a clearly defined set of words.

Our models provide actionable intelligence by directly surfacing customer complaints in real time, and even can measure the impact of a problem as it is occurring. All of this is to help contact center teams better recognize and react to new customer behaviors as they develop.
In the process of training a model to distinguish current behavior from past, we’ve also gotten:
- a list of high confidence problem statements representing the new behavior in the data
- a model for extracting whatever topic set off the alarm
That new dataset can be used to measure how much traffic is due to the novel problem we discovered. Plus, we can train a classifier to detect the problem the next time it occurs. The trained model can be used to extract historical volumes to see if this issue has happened in the past. It can also be used on incoming data to easily identify this new behavior in the future. Moreover, the models we trained are naturally interpretable, yielding key topic words that can be used in SQL queries for easy analysis.
The system ASAPP built around this new technology operates on a minute time scale. Our models provide actionable intelligence by directly surfacing customer complaints in real time, and even can measure the impact of a problem as it is occuring. All of this is to help contact center teams better recognize and react to new customer behaviors as they develop. Equipped with anomaly detection, CX organizations can more efficiently address unexpected events, then analyze these unique situations to understand and prepare for them.