Adrian Botta
.jpeg)
Adrian Botta is a Data Scientist at ASAPP, where he builds products to help our customers understand how agents can more effectively use their tools and identify opportunities for automation. Prior to ASAPP, Adrian studied Economics and Statistics at Carnegie Mellon University and worked at PwC’s AI Accelerator, focused on building natural language processing and computer vision models for customers in construction management, healthcare, and reinsurance.
Mapping the Agent Journey is more than just a time saver for agents
How to start assessing and improving the way your agents use their tools
Customer care leaders tasked with improving customer satisfaction while also reducing cost often find it challenging to know where to begin. It’s hard to know what types of problems are ideal for self-serve, where the biggest bottlenecks exist, what workflows could be streamlined, and how to provide both the right training and targeted feedback to a large population of agents.
We talk with stakeholders from many companies who are charged with revamping the tools agents have at their disposal to improve agent effectiveness and decrease negative outcomes like call-backs and handle time.
Some of the first questions they ask are:
- Where are my biggest problem areas?
- What can I automate for self-service?
- What needs to be streamlined?
- Where are there gaps in my agents’ resources?
- Are they using the systems and the tools we’re investing in for the problems they were designed to help solve?
- What are my best agents doing?
- Which agents need to be trained to use their tools more effectively? And on which tools?
These questions require an understanding of both the tools that agents use and the entire landscape of customer problems. This is the first blog in a series that details how an ASAPP AI Service, JourneyInsight, ties together what agents are saying and doing with key outcomes to answer these questions.
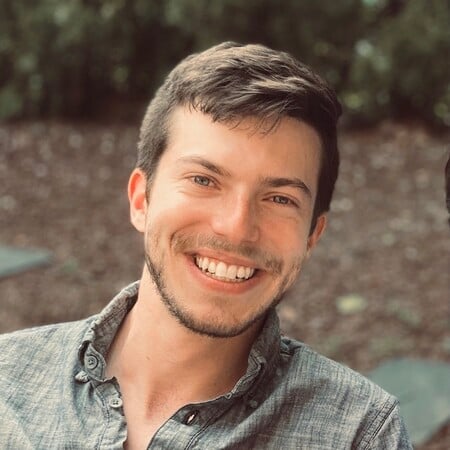
Using JourneyInsight, customer care leaders can make data-driven, impactful improvements to agent processes and agent training.
Most customers start with our diagnostic reports to identify problem areas and help them prioritize improvements. They then use our more detailed reports that tie agent behavior with outcomes and compare performance across agent groups to drive impactful changes.
These Diagnostic reports provide visibility and context behind KPIs that leaders have not had before.
Our Time-on-Tools report captures how much time agents spend on each of their tools for each problem type or intent. This enables a user to:
- Compare tool usage for different intents,
- Understand the distribution of time spent on each tool,
- Compare times across different agent groups.
With this report, it’s easy to see the problem types where agents are still relying on legacy tools or how the 20% least tenured agents spend 30% more time on the payments page than their colleagues do for a billing related question.
Our Agent Effort report captures the intensity of the average issue for each problem type or intent, This enables a user to:
- Compare how many systems are used,
- See how frequently agents switch between different tools,
- Understand how they have to interact with those tools to solve the customer’s problem.
With this report, it’s easy to identify which problem types require the most effort to resolve, how the best agents interact with their tools, and how each agent stacks up against the best agents.
These examples illustrate some of the ways our customers have used these reports to answer key questions.
What can I automate for self-service?
When looking for intents to address with self-serve capabilities, it is critical to know how much volume could be reduced and the cost of implementation. The cost can be informed by how complex the problem-solving workflows are and which systems will need to be integrated with.
Our diagnostic reports for one customer for a particular intent showed that:
- Agents use one tool for 86% of the issue and agents switch tabs infrequently during the issue
- Agents copy and paste information from that primary tool to the chat on average 3.2 times over the course of the issue
- The customer usually has all of the information that an agent needs to fill out the relevant forms
- Agents consult the knowledge base in only 2.8% of the issues within that intent
- There is very little variation in the way the agents solve that problem
All of these data points indicate that this intent is simple, consistent, and requires relaying information from one existing system to a customer. This makes it a good candidate to build into a self-serve virtual agent flow.
Our more detailed process discovery reports can identify multiple workflows per intent and outline each workflow. They also provide additional details and statistics needed to determine whether the workflow is ideal for automation.
Where are there gaps in my agents’ resources?
Correct resource usage is generally determined by the context of the problem and the type or level of agent using the resource.
Our diagnostic reports for one customer for a particular intent showed that:
- A knowledge base article that was written to address the majority of problems for a certain intent is being accessed during only 4% of the issues within that intent
- Agents are spending 8% of their time during that intent reaching out to their colleagues through an internal web-based messaging tool (e.g. Google Chat)
- Agents access an external search tool (e.g. Google search) 19% of the time to address this intent.
- There are very inconsistent outcomes, such as AHT ranging from 2 minutes to 38 minutes and varying callback rates by agent group
These data points suggest that this intent is harder to solve than expected and that resources need to be updated to provide agents with the answers they need. It is also possible that agents were not aware of the resources that have been designed to help solve that problem.
We followed up to take a deeper look with our more detailed outcome drivers report. It showed that when agents use the knowledge base article written for that intent, callback rates are lower. This indicates that the article likely does, in fact, help agents resolve the issue.
In subsequent posts, we’ll describe how we drive more value using predictive models and sequence mining to help identify root causes of negative outcomes and where newer agents are deviating from more tenured agents.