Blog
Using voice of the customer to drive conversation-powered operations
The key to happy customers? Learn from your best agents.
Using automation to increase revenue during customer conversations
When should a customer experience agent upgrade or upsell a customer? Even for a talented sales agent, it can be difficult to answer this question—choosing the right timing and the right offer in the flow of the conversation whether over messaging or a phone call. Add a steady stream of interactions, this year’s unprecedented demand for support, and the imperative to minimize handle time and it becomes next to impossible.
Since inception we’ve been helping businesses solve crucial customer experience challenges like this one by developing cutting edge AI. Most recently, we were granted a patent for “Automated Upsells in Customer Conversations.” The promise of our technology hinges on those challenges that every CX agent is facing along with the massive scale at which our customers operate.
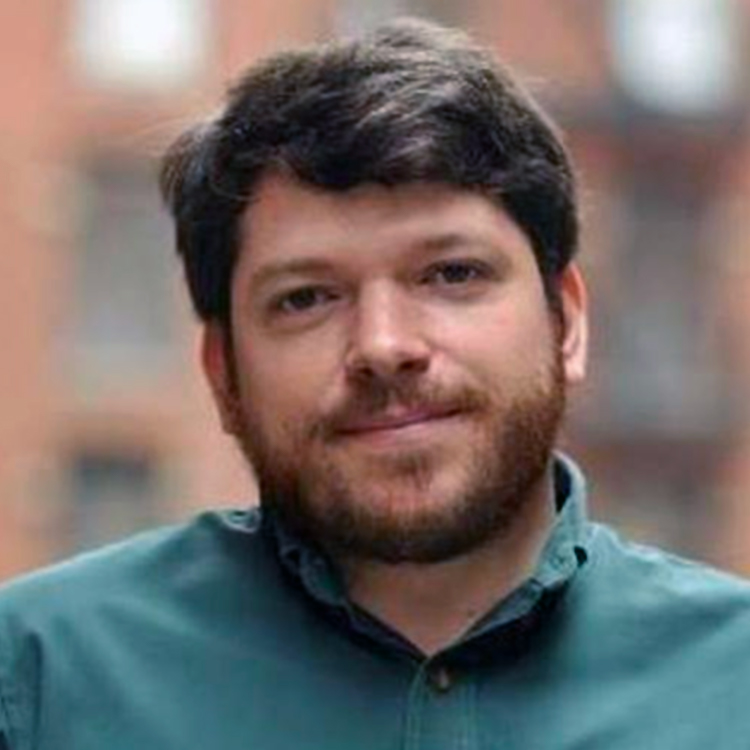
Using AI to predict when to upsell and what to offer can help consumer companies increase sales and grow revenue.
Shawn Henry, PhD
When agents are rushing from issue to issue, there’s often not enough time to access and contemplate the history and preferences of an individual customer. Yet we know that everything from a customer’s next billing date to their list of purchased products could be valuable predictors of their interest in a new product offering or upgrade. In fact, because our models can learn from millions or billions of related customer data points, we can both extract novel correlations and effectively leverage those insights in real time.
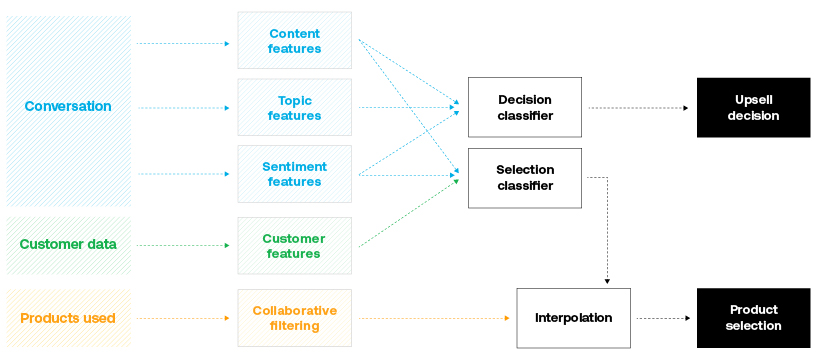
While many solutions in Customer Experience involve generic one-size-fits-all automations, our machine learning models consume a variety of customer specific data points to augment an expert human agent. Our model begins by breaking down the conversation (whether in text or voice) into the content so far, the topic(s) of conversation, and sentiment of the customer. This context is complemented by customer data, including metadata on their account, preferences, products etc. When deployed at contact centers that employ thousands, or tens of thousands of people, speech recognition, natural language processing and machine learning make it practical to determine what conversations are going to deliver higher likelihood of upsell success, even when customers call in for many different reasons and in different emotional states. Sometimes, for example, a customer may be very agitated, and an upsell attempt could not only degrade their experience but harm their perception of the brand, so our model may actually discourage an upsell in favor of maintaining and strengthening that relationship.
In addition to a customer’s own data, the product selection can be determined by interpolating that data with the product preferences of similar customers, as is done with collaborative filtering. All of these factors inform better predictions. Being able to help customers knowledgeably with new products, upgrades or offerings, regardless of department, can be done without friction as the ASAPP platform can automatically surface the right upsell opportunities at the right time to agents across a CX workforce to help grow revenues.
Regardless of industry and agnostic to the channels customers use to communicate, businesses can benefit by surfacing upsell opportunities automatically to agents. ASAPP’s patented technology can dramatically transform this process and accelerate revenue growth as well as a company’s journey to providing unparalleled customer experience.
Real results in weeks—not months (or years!)
Rethink your approach to AI to realize monumental value.
How model calibration leads to better automation
Machine learning models offer a powerful way to predict properties of incoming messages such as sentiment, language, and intent based on previous examples. We can evaluate a model’s performance in multiple ways:
Classification error measures how often its predictions are correct.
Calibration error measures how closely the model’s confidence scores match the percentage of time the model is correct.
For example, if a model is correct 95% of the time, we’d say its classification error is 5%. If the same model always reports it is 99% sure its answers are correct, then its calibration error would be 4%. Together, these metrics help determine whether a model is accurate, inaccurate, overconfident, or underconfident.
Reducing both classification error and calibration error over time is crucial for integration into human workflows. It enables us to maximize customer impact in an iterative manner. For example, well-calibrated models can trigger mature platform features to use more automation only when they have a high chance of succeeding. Furthermore, proper calibration creates an intuitive scale on which to compare multiple models, so that the overall system always utilizes the most confident prediction and that this confidence matches how well the system will actually perform.
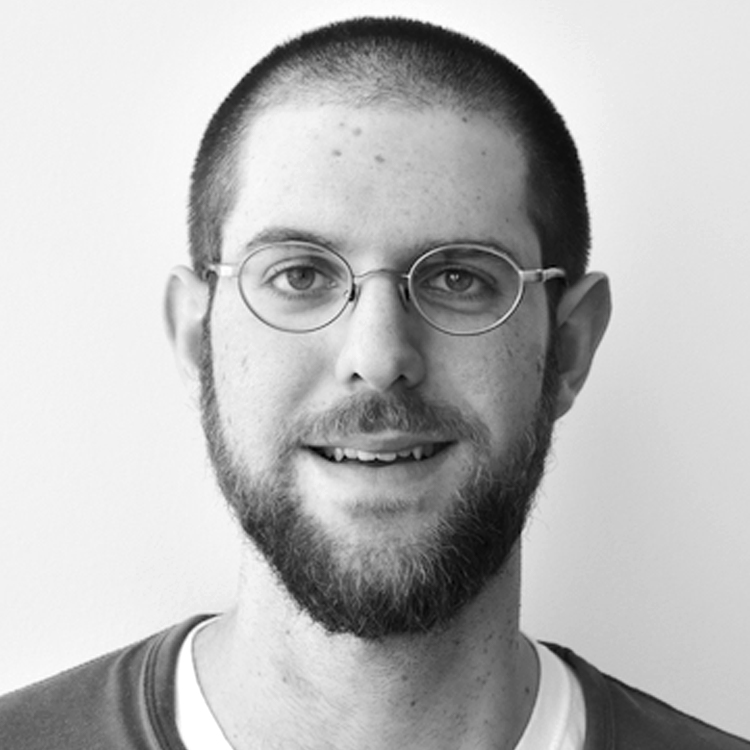
Automating workflows requires a high degree of confidence in the ML model predicting that this is what is needed. Proper calibration increases that confidence.
Ethan Elenberg, PhD
The models developed by ASAPP provide value to our customers not from raw predictions alone, but rather from how those predictions are incorporated into platform features for our users. Therefore, we take several steps throughout model development to understand, measure, and improve the accuracy of confidence measures.
For example, consider the difference between predicting 95% chance of rain versus 55% chance of rain. A meteorologist would recommend that viewers take an umbrella with them in the former case but might not in the latter case. This weather prediction analogy fits many of the ASAPP models used in intent classification and knowledge-base retrieval. If a model predicts “PAYBILL” with score 0.95, we can send the customer to the “Pay my bill” automated workflow with a high degree of confidence that this will serve their need. If the score is 0.55, we might want to disambiguate with the customer whether they wanted to “pay their bill” or do something else.
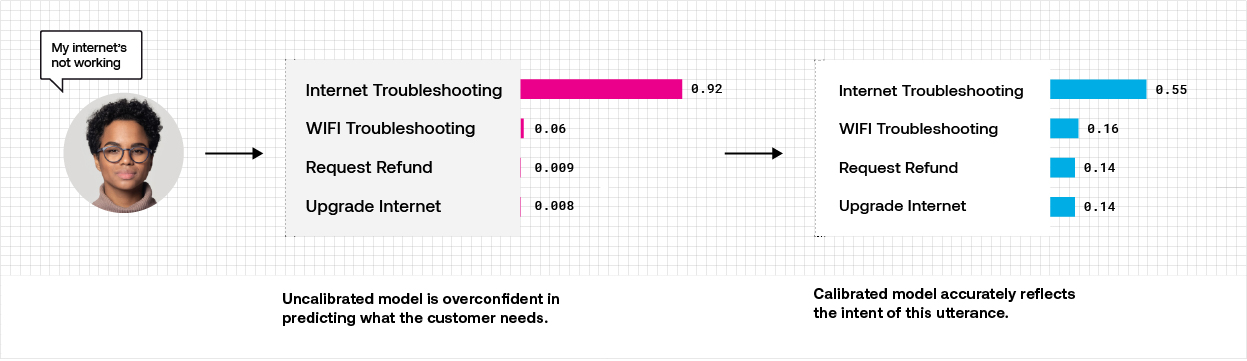
Following our intuition, we would like a model to return 0.95 when it is 95% accurate and 0.55 when it is only 55% accurate. Calibration enables us to achieve this alignment. Throughout model development, we track the mismatch between a model’s score and its empirical accuracy with a metric called expected calibration error (ECE). ASAPP models are designed with a method called temperature scaling, which adjusts their raw scores. This changes the average confidence level in a way that reduces calibration error while maintaining prediction accuracy. The results can be significant: For example, one of our temperature scaled models was shown to have 85% lower ECE than the original model.
When ASAPP incorporates AI technology into its products, we use model calibration as one of our main design criteria. This ensures that multiple machine learning models work together to create the best automated experience for our customers.
In Summary
Machine learning models can be either overconfident or underconfident in their predictions. The intent classification models developed by ASAPP are calibrated so that prediction scores match their expected accuracy—and deliver a high level of value to ASAPP customers.
CX: A value center, not just a cost center
How a little micro-automation delivers big productivity gains
Training natural language models to understand new customer events in hours
Named entity recognition (NER) aims to identify and categorize spans of text into entity classes, such as people, organizations, dates, product names, and locations. As a core language understanding task, NER powers numerous high-impact applications including search, question answering, and recommendation.
At ASAPP, we employ NER to understand customer needs, such as upgrading a plan to a specific package, making an appointment on a specific date, or troubleshooting a particular device. We then highlight these business-related entities for agents, making it easier for them to recognize and interact with key information.
Typically, training a state-of-the-art NER model requires a large amount of annotated data, which can be expensive and take weeks to obtain. However, a much more rapid response is demanded by many business use cases. For example, you can’t afford a weeks-long wait when dealing with emerging entities like those related to an unexpected natural disaster or a fast-changing pandemic such as with COVID-19.
My colleague PostDoc researcher Arzoo Katiyar and I solve this challenge using few-shot learning techniques. In few-shot learning, only a few labeled examples (typically one to five examples) are needed for the ML algorithm to train. We applied this technique to NER by transferring the knowledge from existing NER annotations from public NER datasets which only focus on entities in the general domain to each new target entity class. This is achieved by learning a similarity function between a target word and an entity class.

When we apply structured nearest neighbor learning techniques to named entity recognition (NER) we’re able to double performance, as shared in our paper published at EMNLP 2020. This translates to higher accuracy—and better customer experiences.
Yi Yang, PhD
Few-shot learning techniques have been applied to language understanding tasks in the past. However, compared to the reasonable results obtained by the state-of-the-art few-shot text classification systems (e.g., classify a web page into a topic such as Troubleshooting, Billing, or Accounts), prior state-of-the-art NER performance was far from satisfactory.
Moreover, existing few-shot learning techniques typically require the training and deployment of a fresh model, in addition to the existing NER system, which can be costly and tedious.
We identified two issues with adopting the existing few-shot learning techniques for NER. First, the existing techniques assume that each class has a single semantic meaning. NER adds a special class called ‘Outside’, which means it is not one of the entities that this specific model is looking for. If a word is labeled as ‘Outside’, it means that it doesn’t fit in the current set of entities, however, it can still be a valid entity in another dataset. This suggests that the ‘Outside’ class corresponds to multiple semantic meanings.
Second, they fail to model label-label dependencies, e.g., given the phrase “ASAPP Inc”, knowing that “ASAPP” is labeled as ‘Organization’ would suggest that “Inc” is also likely to be labeled as ‘Organization’.
Our approach is to address the issues with nearest-neighbor learning and structured decoding techniques, as detailed in our paper published at EMNLP 2020.
By combining the techniques, we are able to create a novel few-shot NER system that outperforms the previous state-of-the-art system by doubling the F1 score (standard evaluation metric for NER, similar to accuracy) from 25% to 50%. Thanks to the flexibility of the nearest neighbor technique, the system can work on top of the conventionally deployed NER model, which eliminates the expensive process of model retraining and redeployment.

The work reflects the innovation principle of our world-class research team at ASAPP—identify the most challenging problems in customer experience, and push hard to overcome the challenge without worrying about the confines of current limits.