Blog
Generating New Customer Intelligence
Contact centers are goldmines of market information – from addressing customer issues and gauging their wants and needs, to seeing how they rate you in comparison to your competitors, and more. Customer interactions contain valuable information to improve current products and can provide early warning signals for any potential issues or emerging competition.
Despite the valuable information available, it has been incredibly difficult to access: deciphering phone audio or messaging transcripts is an arduous task. Text analysis has provided some assistance, but more often than not the optimal solution has been to ask the agent or customer on the call to fill out a survey with the data we care about.
Agents can be extremely effective at filling out these surveys, yet at a cost: adding questions is very expensive, and you are only able to acquire future data. Analyzing historical trends is still an onerous task. Requesting feedback from customers proves more difficult as well; sampling bias becomes an issue while other obstacles may occur. Leveraging quality management data for insights quickly runs into sparsity issues, making proactive responses too slow.
At ASAPP, we’ve incorporated Large Language Models (LLMs) to solve this problem for years as part of our Structured AutoSummary product. LLMs are great at understanding the meaning of the text. We can use them to regularize the recording of the interaction. We can represent conversations as a free text summary, and we can pull structured data out of conversations.
Newer LLMs can also perform a facsimile of reasoning. GPT4 and other models can be great at answering questions that require combining pieces of information in a call transcript. That extends the number of questions we can answer with high confidence – and the amount of structured data we can extract from conversations.
Structured data remains essential. Although LLMs can be very good at analyzing a single conversation, it takes a different approach to analyze hundreds or millions of customer interactions. Traditional analytics approaches – e.g. BI tools, Excel, ML models, etc – are the best way to analyze, identify patterns, and understand trends across a large amount of data. Now we can expose customer interaction data in a way those analytical tools understand.
Certainly, there are some complications in relying on AI to convert unstructured conversations to a usable structured format. At ASAPP, we’ve devoted substantial effort to managing hallucinations and reliable data collection by building in dedicated feedback loops and having multiple models working together that tackle different aspects of hallucinations.
Not surprisingly, the quality of data matters too. We’ve benchmarked the quality of our AutoSummary outputs against ASR accuracy (1-WER), and we see that highly accurate transcripts (where our own generative end-to-end ASR system AutoTranscribe sits in the mix) produce materially higher quality data on downstream tasks like extracting structured data out of conversations.
Turning unstructured audio and text into structured data unlocks a wealth of data stored in contact center records. Utilizing existing analysis tools and approaches can make contact center data available to other departments, like Research and Development, Marketing, and Finance, in real-time without purchasing additional IT capabilities for analysis and visualization.
For agents, this provides a massive boost in efficiency, getting quick answers to business questions and freeing up more time to help customers. For customers, it’s even more dramatic. They get answers faster and have a much smoother experience overall – all without survey bias.
LLMs are fantastic tools for language: writing poetry, essays, and code. They are also great at turning natural language into structured data, blurring or eliminating the boundary between “structured data” and “unstructured data.” Leveraging that data, and making it available to all the existing business processes, is where we’re heading with Structured AutoSummary.
Generative AI for Agent Augmentation: Agents Models not Language Models
Generative AI and Large Language Models (LLMs) have made massive waves in the consumer and enterprise technology news due to the remarkable capabilities of tools like ChatGPT and GPT4. The models provide fluent text that integrate reasoning into their responses based on the knowledge they have absorbed from the huge volume of general documents they are trained on. Not surprisingly, their use in chatbots has been one of the most active development areas for enterprise businesses.
While increasing containment of calls can save call centers dollars, the primary cost is still front line agents who must handle the most challenging calls and customers (otherwise a bot would have handled it). At ASAPP, we already have many tools that assist the agent when handling a live call. AutoCompose helps agents craft messages and significantly increases throughput in the call center while increasing CSAT in tandem. AutoSummary helps automate dispositioning steps for agents. Both use Generative AI models, in some cases for approximately five years.
However, agents spend their time doing much more than just writing messages to customers. They must execute actions on the customer’s behalf (e.g., change a seat on a flight or schedule a technician visit) as well as follow flows and instructions in knowledge base articles to be compliant when handling issues with safety or business regulations. To do this agents use a large number of tools. These tools are rarely homogeneous but are a frankenstack of vendors and user interfaces. On top of that, agents handling digital calls are usually managing more than one issue at a time, which leads to a huge number of applications open at once. Any model that focuses only on the text of a conversation and not all the actions the agent is executing is leaving a huge amount of headroom on the floor. For many of our customers agents can spend upwards of 60% of their time on tools outside of the conversation!!
Thus, to truly augment the agent a model must not just be a Language Model – it must be an Agent Model. That is, it needs to be a multimodal model that operates not just on the text of the conversation, but also on all the information the agent is currently interacting with as well as information hidden in business documents and logic that are salient for the issues at hand. At ASAPP, we have already invested in understanding the data stream of all agent actions and have used that data stream to build multimodal models that can improve augmentation for an agent. There is an amazing synergy when using this data. First, conditioning on the agent action data stream allows us to better improve our predictions of what the agent should say and do next. Conversely, information from the conversation feeds into what actions the agent should do, i.e., ‘I need to book a flight from New York to San Fran tomorrow in the morning’ allows the model to predict a flight search action, populate the origin city with ‘New York’, the destination to ‘San Francisco’ and the date as a day from today and execute that command.
Varying levels of experience with internal tools will impact how consistently advisors are solving customer problems. We commonly see less tenured representatives reaching out to their colleagues more often after getting stuck using an internal tool, spending more time searching for knowledge base articles, and switching back and forth more often between screens when handling workflows. Agent models can help newer agents become more comfortable and guide them to more effectively use their tools.
A core aspect of ASAPP’s mission is to ‘multiply agent productivity’. This can only be achieved in its fullest with Agent Models and not just Language Models.
Generative AI: When to Go Wide and When to Go Deep
Generative AI is everywhere, and you might be feeling the pressure from your colleagues or managers to explore how to incorporate Generative AI into your role or business. I’ve been seeing a lot of speculation about ChatGPT’s capabilities and what it can and cannot do. As a research scientist with years of experience in academic and industrial research with large language models, I wanted to dig into some of these notions:
ChatGPT isn't a product
First, ChatGPT is not a product, it’s an engine – and a really good one. However, a valuable solution still needs more in order to make a difference and drive business value in almost every case. This includes the UX (UI, latency, runtime constraints) and critical ML capabilities like data collection, data processing and selection, continuous training frameworks, optimizing models for outcomes (beyond next word prediction) and deployment (measurement, A/B tests, telemetry).
Solving specific business problems
Second, while GPT does amazing things like write poetry, pass medical exams or write code (just to name a few), in CX we need solutions that solve specific problems like improving automated dispositioning or real-time agent augmentation. GPT models can be impressive, but when it comes to user experience and business outcomes, Vertical generative AI models that are trained on human data in a dynamic environment specifically for the task at hand typically outperform larger generic algorithms. In ASAPP’s case, this means solving customer experience pain points and building technology to make agents more productive.
Grounding with data
Lastly, while we don’t use ChatGPT at ASAPP, we do train large language models and have deployed them for years. We don’t pre-train them on the web, but we do pre-train them on our customer data, which is quite sizable. From there, we then train them to solve specific tasks optimizing the model for specific KPIs and business outcomes we care about and need to solve for our customers — not just general AI. This includes purpose-built vertical AI technology for contact centers and CX. Vertical AI allows enterprises to transform by automating workflows, multiplying agent productivity and generating customer intelligence to provide optimal CX.
Interested in learning more about ChatGPT or how large language models might benefit your business? Drop us a line.
Automation should help resolve, not deflect
The mission of Solution Design at ASAPP is to help companies identify areas of massive opportunity when it comes to optimizing their contact centers and digital customer experiences. At the highest level, we aim to help our customers provide an extremely personalized and proactive experience at a very low cost by leveraging machine learning and AI. While we’ve seen many different cost saving and personalization strategies when it comes to the contact center, the most common by far is as follows:
- Step 1:
Leverage self service channels (website, mobile apps, etc) built by digital teams and hope customers resolve issues themselves or buy products directly. - Step 2:
If customers aren’t able to solve issues on their own, offer “assistance” using an IVR or chatbot, with the goal of preventing customers from talking to an agent. - Step 3:
When these fail, because the question is too complex or there isn’t an easy way to self serve, have an agent handle it as quickly as possible, often starting from scratch.
It’s a strategy that many Fortune 500 companies were convinced would revolutionize the customer experience and bring about significant cost savings. Excited by the promises of chatbot and IVR companies who said they could automate 80% of interactions within a year—which they assumed would reduce the need for agents to handle routine tasks– companies spent millions of dollars on these technologies.
Automation as you know it isn’t working
While some are seeing high containment numbers put forth in business cases, the expected savings haven’t materialized—as evidenced by how much these companies continue to spend on customer service year after year. Furthermore, customers are frustrated by this strategy—with most people (myself included) asking repeatedly for an agent once they interact with an IVR or bot. The fact is, people are calling in about more complex topics, which require knowledgeable and empathetic people on the other end of the line.

We live in a new era where the companies who can provide extremely efficient and personalized interactions at a lower cost than their competitors are winning out.
Austin Meyer
It’s not surprising that in 2019, executive mentions of chatbots in earnings calls dropped dramatically and chatbot companies struggled to get past seed rounds of investment (cite). These programs cost millions of dollars in software and tooling, and double or triple that for the labor involved with building, maintaining, measuring, and updating logic flows. Beyond NOT increasing contact center efficiency, chatbot technology has reduced customer satisfaction, impeded sales conversion, and has caused the market to missassociate AI with automate everything or nothing.
A better automation strategy
We live in a new era where the companies who can provide extremely efficient and personalized interactions at a lower cost than their competitors are winning out.
There has been a retreat from using bot automation to avoid customer contact. Instead, leading companies are using ML and AI to improve digital customer experiences while simultaneously helping agents become more efficient. Furthermore, by connecting the cross channel experiences and using machine learning across them, conversational data is much more complete and more valuable to the business.
Compared to the earlier strategy, where there were distinct walls between self service, automation and agents, this new strategy looks far more blended. Notice that automation doesn’t stand alone—instead, it’s integrated with the customer experience AND agent workflows. Machine learning provides efficiency gains by enabling automation whenever appropriate, leading to faster resolution regardless of channel.

At ASAPP, we use AI-driven agent augmentation and automation to improve customer experience and increase contact center efficiency. The results have been transformative—saving our customers millions of dollars in opex, generating millions in additional revenue while dramatically improving CSAT/NPS and digital engagement. If you want to learn more about our research, results, or strategy reach out to me at solutions@asapp.com.
Are you tapping into the power of personalization?
For decades, one of the biggest consumer complaints has been that companies don’t really know them. Businesses may use segmentation for marketing, yet for inbound customer service, even this level of personalization is nearly non-existent. Now the race is on—because personalized service experiences are quickly becoming a brand differentiator.
When customers reach out to solve a problem, they want to feel reassured and valued. But too often, they’re treated like a number and end up more frustrated. Even if they get good service on one call, the next time they contact customer service it’s basically starting at ground zero because the next agent doesn’t know them.
As more digital customer service channels have emerged, consumers have gained more choices and digital convenience. But that creates a new challenge: people often use different channels at different times, switching between calls, web chat, digital messaging, and social media. And because those channels are often siloed, customers may get a very impersonal and disjointed experience.
The new demand for personalization requires something significantly better. Consumers now expect seamless experiences across their relationship with a company—and without it, brands will struggle to earn repeat business, let alone loyalty. In fact, nearly 60% of consumers say personalized engagement based on past interactions is very important to winning their business.
Increasing value with a unified channel journey
Knowing your customers means providing seamless continuity wherever they engage with your brand. Typically, the experience is fragmented, and consumers have a right to expect better. They provide a considerable amount of data through various channel interactions, and 83% of consumers are willing to share that data to receive more personalized experiences.
When a company barely knows them from one engagement to the next, how do you think that affects their trust in the brand?
It’s no surprise that 89% of digital businesses are investing in personalization. Cutting edge technologies are eliminating the friction and fragmentation of multi-channel journeys, by meeting customers with full context however they make contact. With a unified, AI-powered platform for customer experience, companies can seamlessly integrate voice and digital capabilities—and ensure customers are greeted every time with an understanding of their history with the company, where they’ve been and what happened in previous interactions It gives customers greater flexibility and ease for engaging using their preferred channels, which can dramatically improve satisfaction ratings and NPS scores.
Another powerful benefit of multi-channel integration is that it enables contact centers to think in terms of conversations instead of cases. A unified platform weaves together voice and digital messages into a cohesive thread for a given customer. Any agent can easily step in and join that conversation, having all the right knowledge about the situation and visibility into previous interactions. That continuity enables agents to provide more personalized attention that helps ensure the customer feels known and valued.
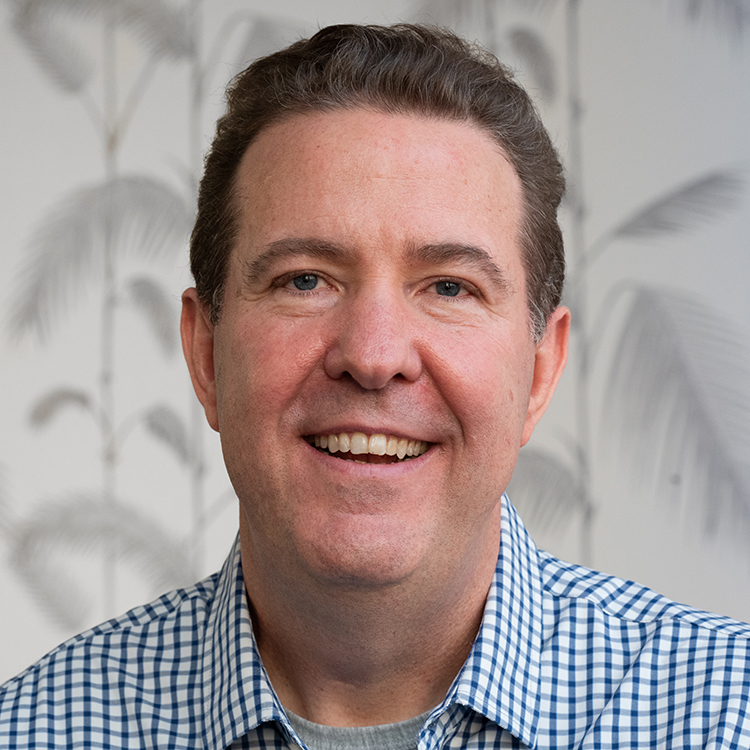
Customer service needs to be about conversations, not cases. Creating intelligent, personalized continuity across all engagement channels shows customers you know and value them—and that’s the great CX that wins loyalty.
Michael Lawder
Improving proactive engagement with personalization
Tapping into a wealth of customer data from many different channels, companies can take customer experience to the next level. Using AI and machine learning, you can build more comprehensive customer histories and serve up predictive, personalized action plans specifically relevant for each customer.
I’m talking about gaining a holistic picture of when, why, and how each customer has engaged over their lifecycle with your company. That opens up significant opportunities, such as:
- Improve customer experience and earn loyalty
- by providing highly personalized support each time someone reaches out.
- Increase customer lifetime value
- with more relevant and timely proactive engagement that is more like personalized conversations, all based on data-driven insights.
- Boost marketing ROI
- using customer data to develop persona-based segmentation strategies and nuanced messaging driven by sentiment analysis and a deeper understanding of intent.
Most consumers now expect companies to know them better and see that reflected in their communications. And the demand for personally relevant experiences isn’t just about marketing—it’s across the journey, including customer service. That’s why ASAPP technology is so compelling.
Support interactions are often the defining moments that dictate how people feel about a brand. The more you can personalize those customer service moments, the more you will earn loyalty, and even word-of-mouth referrals as your happy customers become brand advocates.
Mapping the Agent Journey is more than just a time saver for agents
Designing AutoCompose
ASAPP researchers have spent the past 8+ years pushing the limits of machine learning to provide contact center agents with content suggestions that are astonishingly timely, relevant, and helpful. While these technological advancements are ground-breaking, they’re only part of the success of AutoCompose. Knowing what the agent should say or do next is only half the battle. Getting the agent to actually notice, trust, and engage with the content is an entirely different challenge.
While it may not be immediately noticeable to you as a customer, contact center agents often navigate a head-exploding mash-up of noisy applications and confusing CRMs when working towards a resolution. Beyond that, they juggle well-meaning protocols and policies that are intended to ensure quality and standardize workflows, but instead quickly become overwhelming and ineffective—if not counter-productive.
The ASAPP Design team took notice of this ever-growing competition for attention and sought to turn down the noise when designing AutoCompose.
Instead of getting bigger and louder in our guidance, we focused on a flexible and intuitive UI that gives agents the right amount of support at exactly the right time—all without being disruptive to their natural workflow.
Min Kim
We had several user experience imperatives when iterating on the AutoCompose UI design.
Be convenient, but not disruptive
Knowing where to showcase suggestions isn’t obvious. We experimented with several underperforming placements until landing on the effective solution: wedging the UI directly in the line of sight between the composer input and the chat log. The design was minimalist, keeping visual noise to a minimum and focusing on contrast and legibility.

The value of AutoCompose stems from recognition rather than recall, which takes advantage of the human brain’s ability to digest recent and contextual information at a time. Instead of memorizing an infinite number of templates and commands, AutoCompose includes suggestions in multiple locations where an agent can recognize and choose. When the agent is in the middle of drafting a sentence, Phrase Auto-Complete prompts the suggested full sentence inline within the text input. As an agent types words and phrases, AutoSuggest gives the most relevant suggestions at the given context, located between the chat log and composer, so that the agent can stay informed about the chat context. By placing suggestions where they need it, agents can immediately recognize and utilize them with maximum efficiency.
Just the right amount
In UI design, there is often a fine line between too much and too little. We experienced this when evaluating the threshold for how many suggestions to display. AutoSuggest currently displays up to three suggestions that update in real-time as an agent types. We’ve been intentional about capping suggestions to a maximum of three, and do our best effort to make them relevant. The model only shows confident, quality-ensured suggestions above a set threshold. With this, the UI shows the right amount of suggestions that optimize for the cognitive load that agents can handle at a time.

Speed matters
Another critical component to the design is latency. To fit within an agent’s natural workflow, the suggestions must update within a fraction of a second—or risk the agent ignoring the suggestions altogether.
Specifically, a latency of less than 100ms ensures the agent feels a sense of direct manipulation associated with every keystroke. Beyond that, the updating of suggestions can fall behind the pace of conversation, making the experience painfully disjointed.
Support the long tail of use cases
In contact centers, when agents encounter complex issues, they may choose to resolve them differently depending on their tenure and experience. In these scenarios, we may not have the right answer, so we instantly shift our UX priorities to make it easy for the agents to find what they’re looking for.
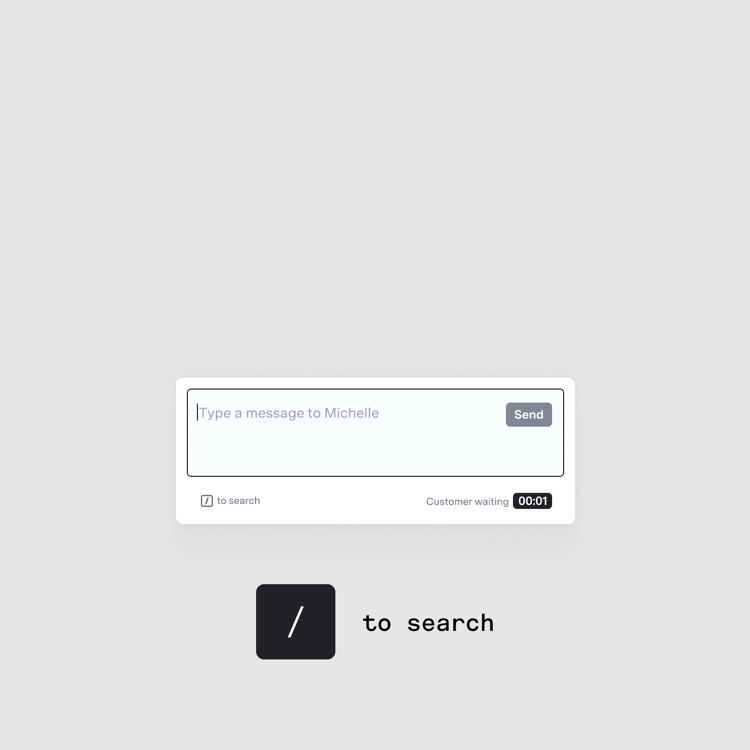
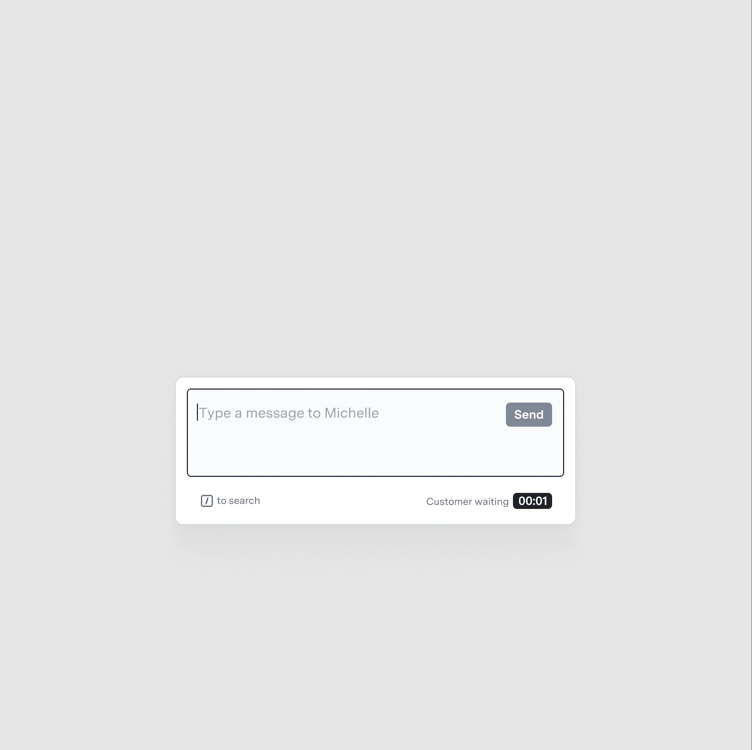
We focused on integrating search and other browsing (and use of shortcuts), all in a compact, but extremely dynamic UI. Experienced agents may need to pull effective responses that they built on their own. Meanwhile, novice agents need more handholding to pull from company-provided response suggestions, also known as global responses. To accommodate both, we experimented with ways to introduce shortcuts like a drawer search inline within the text field, and a global response search that is prompted on top of AutoSuggest. AutoCompose now accommodates these long tail use cases with our dynamic, contextual UI approach.
What might seem like a simple UI is actually packed with details and nuanced interactions to maximize agent productivity. With subtle and intentional design decisions, we give the right amount of support to agents at the right time.
How to start assessing and improving the way your agents use their tools
Customer care leaders tasked with improving customer satisfaction while also reducing cost often find it challenging to know where to begin. It’s hard to know what types of problems are ideal for self-serve, where the biggest bottlenecks exist, what workflows could be streamlined, and how to provide both the right training and targeted feedback to a large population of agents.
We talk with stakeholders from many companies who are charged with revamping the tools agents have at their disposal to improve agent effectiveness and decrease negative outcomes like call-backs and handle time.
Some of the first questions they ask are:
- Where are my biggest problem areas?
- What can I automate for self-service?
- What needs to be streamlined?
- Where are there gaps in my agents’ resources?
- Are they using the systems and the tools we’re investing in for the problems they were designed to help solve?
- What are my best agents doing?
- Which agents need to be trained to use their tools more effectively? And on which tools?
These questions require an understanding of both the tools that agents use and the entire landscape of customer problems. This is the first blog in a series that details how an ASAPP AI Service, JourneyInsight, ties together what agents are saying and doing with key outcomes to answer these questions.
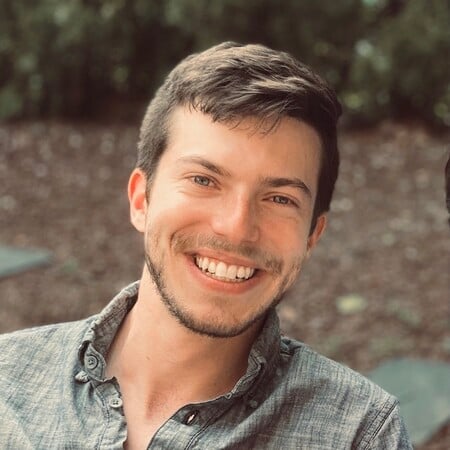
Using JourneyInsight, customer care leaders can make data-driven, impactful improvements to agent processes and agent training.
Most customers start with our diagnostic reports to identify problem areas and help them prioritize improvements. They then use our more detailed reports that tie agent behavior with outcomes and compare performance across agent groups to drive impactful changes.
These Diagnostic reports provide visibility and context behind KPIs that leaders have not had before.
Our Time-on-Tools report captures how much time agents spend on each of their tools for each problem type or intent. This enables a user to:
- Compare tool usage for different intents,
- Understand the distribution of time spent on each tool,
- Compare times across different agent groups.
With this report, it’s easy to see the problem types where agents are still relying on legacy tools or how the 20% least tenured agents spend 30% more time on the payments page than their colleagues do for a billing related question.
Our Agent Effort report captures the intensity of the average issue for each problem type or intent, This enables a user to:
- Compare how many systems are used,
- See how frequently agents switch between different tools,
- Understand how they have to interact with those tools to solve the customer’s problem.
With this report, it’s easy to identify which problem types require the most effort to resolve, how the best agents interact with their tools, and how each agent stacks up against the best agents.
These examples illustrate some of the ways our customers have used these reports to answer key questions.
What can I automate for self-service?
When looking for intents to address with self-serve capabilities, it is critical to know how much volume could be reduced and the cost of implementation. The cost can be informed by how complex the problem-solving workflows are and which systems will need to be integrated with.
Our diagnostic reports for one customer for a particular intent showed that:
- Agents use one tool for 86% of the issue and agents switch tabs infrequently during the issue
- Agents copy and paste information from that primary tool to the chat on average 3.2 times over the course of the issue
- The customer usually has all of the information that an agent needs to fill out the relevant forms
- Agents consult the knowledge base in only 2.8% of the issues within that intent
- There is very little variation in the way the agents solve that problem
All of these data points indicate that this intent is simple, consistent, and requires relaying information from one existing system to a customer. This makes it a good candidate to build into a self-serve virtual agent flow.
Our more detailed process discovery reports can identify multiple workflows per intent and outline each workflow. They also provide additional details and statistics needed to determine whether the workflow is ideal for automation.
Where are there gaps in my agents’ resources?
Correct resource usage is generally determined by the context of the problem and the type or level of agent using the resource.
Our diagnostic reports for one customer for a particular intent showed that:
- A knowledge base article that was written to address the majority of problems for a certain intent is being accessed during only 4% of the issues within that intent
- Agents are spending 8% of their time during that intent reaching out to their colleagues through an internal web-based messaging tool (e.g. Google Chat)
- Agents access an external search tool (e.g. Google search) 19% of the time to address this intent.
- There are very inconsistent outcomes, such as AHT ranging from 2 minutes to 38 minutes and varying callback rates by agent group
These data points suggest that this intent is harder to solve than expected and that resources need to be updated to provide agents with the answers they need. It is also possible that agents were not aware of the resources that have been designed to help solve that problem.
We followed up to take a deeper look with our more detailed outcome drivers report. It showed that when agents use the knowledge base article written for that intent, callback rates are lower. This indicates that the article likely does, in fact, help agents resolve the issue.
In subsequent posts, we’ll describe how we drive more value using predictive models and sequence mining to help identify root causes of negative outcomes and where newer agents are deviating from more tenured agents.