Blog
Webinar Highlights: Three reasons you're not seeing the value you were promised from your digital chat platform.
Are you not getting the value that you were hoping for from your digital chat platform?
If so, this blog and our recent on-demand webinar are for you.
We recently had a great webinar featuring Melissa Price, VP, CX Digital Self-Service at Altice USA and the experts at ASAPP. In it, we talked about what is holding people back from seeing the value they want out of their existing digital chat platform, what they should look for in a new one, and how they can make that migration as painless as possible.
The conversation is ripe with solutions for some of the widespread pain points CX leaders are experiencing with their digital chat platforms.
Here are some key takeaways from the webinar:
3 Underlying Challenges Holding Platforms Back
In the webinar, we discussed three critical issues that often prevent customer engagement platforms from succeeding:
- Fragmented Customer Journeys: Disconnected touchpoints lead to inconsistent customer experiences. Automation on many platforms is not customer-friendly or efficient, often leading to frustration rather than satisfaction.
- Lack of Adaptability: Many platforms suffer from a lack of ongoing innovation and adaptability. Without continuous improvement loops and the support to keep up with evolving needs, platforms become outdated quickly and fail to deliver the optimized experiences necessary for customer retention and satisfaction. This static approach that many platforms offer contrasts sharply with the dynamic nature of customer service needs.
- Limited Effectiveness of Bolted-on AI: Many legacy chat providers rely on bolted-on AI solutions rather than being AI-native, resulting in subpar suggested responses, ultimately failing to deliver the additional efficiencies, predictive insights, and automation that AI should provide.
How Modern Chat Platforms, Like ASAPP, Address These Problems
Moder, AI-Native® digital chat platforms, like ASAPPMessaging tackles these challenges head-on:
- Seamless customer experience Integration: ASAPP Messaging unifies customer interactions, ensuring a cohesive journey.
- Continuously improving AI-Enhanced Workflows: By automating routine tasks, AI frees agents to focus on complex issues, enhancing both efficiency and satisfaction. The AI continually learns and adapts with use, leading to smarter workflows and ongoing improvements.
- Advanced AI Capabilities: The platform’s AI not only predicts customer needs but also provides real-time support to agents, enhancing decision-making.
What to Look for in a Digital Chat Platform
Choosing the right digital chat platform can significantly impact your operational efficiency and customer satisfaction. Here are some key factors to consider:
- Driving Digital Adoption:
- Encourage Digital Shift: Prioritize a platform that promotes digital engagement over traditional voice channels, which are typically more expensive.
- Cost Efficiency: Digital interactions are far more cost-effective than voice calls.
- Reliability: Ensure the platform has a strong track record with minimal outages.
- Customer Behavior Insights: Research the factors that drive customers to choose digital channels over voice to tailor your strategy accordingly.
- User Experience for Agents and Customers:
- Customer-Focused Design: A platform with excellent user experience (UX) will make customers more aware of and inclined to use chat services.
- Agent Empowerment: Good UX for agents is essential to drive adoption and enhance agent productivity through automation.
- Service-Oriented: The technology should be built to serve both agents and customers, focusing on empowerment rather than control.
- AI-Native and Continuous Innovation:
- Harnessing AI: Choose a platform that is AI-native and continuously innovates, especially given the current surge in AI advancements.
- Custom Insights with GenAI: Platforms that leverage Generative AI can provide customized insights and maintain a competitive edge.
- Experimentation and Adaptability: Opt for providers who constantly experiment with both internal and leading external AI models, rather than relying on standard models driven by financial incentives.
Real Results with Altice
Melissa Price, VP of Customer Experience for Digital Self Serve at Altice, shared compelling results achieved through the implementation of ASAPP’s digital chat platform. She highlighted results, including:
- Increased Efficiency: Significant reduction in handling times and increased resolution rates.
- Enhanced Customer Satisfaction: Improved experiences leading to higher customer satisfaction scores.
- Value of AI-Native Solutions: The AI-native approach of ASAPP was crucial in achieving these outcomes, providing deep insights and automation that traditional platforms couldn’t match.
Switching Platforms
There are short term challenges of switching platforms. But, the long-term benefits—including enhanced operational efficiencies, better customer experiences, and staying ahead of technological advancements—far outweigh the initial transition hurdles.
How to make Migration as painless as possible?
To ensure a smooth migration to a new digital chat platform, start by focusing on thorough planning, selecting the right platform with excellent UX and reliability, and leveraging AI for continuous innovation. Engage stakeholders, provide comprehensive training, and implement in phases to minimize disruptions. For a more comprehensive guide, watch the on-demand recording.
Watch the Webinar On-Demand
Curious about how Altice and ASAPP are transforming customer engagement? Watch our on-demand webinar to gain valuable insights into their groundbreaking strategies and real-world successes. It’s a must-watch if your current digital chat platform isn't meeting your expectations.
Best-of-Breed vs. Omnichannel/CCaaS for the Digital Contact Center
Summary
Choosing the right CX software can be difficult. Learn how to think about choosing between a best-of-breed digital channel solution vs. an omnichannel solution.
Choosing the right CX software can be difficult. In this blog series, our goal is to give you helpful frameworks through which to think about how to purchase the right CX software to help your contact center improve customer experience, augment agent productivity, and reduce costs. (For a thorough breakdown of how to choose the right Transcription and Summarization Solutions, read our buyer’s guide here.)
This blog post covers how to think about acquiring the right Digital Customer Interaction Solution (DCIS) for your contact center. Should you choose a best-of-breed solution, specializing in digital channels, or an omnichannel/CCaaS solution, which offers both voice and digital channels?
Let’s dig in.
The Problem with the “All-in-One Solution”
Omnichannel Solutions, also commonly referred to as Contact Center-as-a-Service Solutions, offer an appealing promise: meet all your CX software needs across channels from just one vendor. The only problem? It’s rare to find an omnichannel solution that offers excellent technology for both voice and digital channels.
Most omnichannel solutions were built to optimize for voice channels and tacked on digital support only as an afterthought. As a result, omnichannel solutions tend to deliver subpar digital experiences, such as poor agent UX not optimized for chat workflows, limited bots, and low chat agent usage, frustrating both customers and agents.
It’s difficult to encourage customers to transition to digital support when you don’t have the technology to make their digital experience as useful and delightful as possible.
Of course, the lack of a comprehensive digital solution has significant consequences for your contact center’s operations.
Digital interactions are 1.5x to 4x cheaper than voice interactions. Contact centers understandably want to shift their mix to increase digital support. But without a digital chat platform that can reliably satisfy customers, it can be difficult to change customer behavior and preferences.
In sum, omnichannel solutions present themselves as an all-around answer, but in reality, their digital support options are severely lacking. What they offer in perceived convenience, they often trade in effectiveness.
Below, we break down how to think about best-of-breed vs. omnichannel solutions:
Interested in Learning More About Building a Digital-First Contact Center?
A digital-first contact center is essential to running a modern, efficient, and customer-friendly customer support operation.
Request a demo of ASAPP here to learn how ASAPP customers are:
- Automating Tier 1 interactions
- Experiencing happier, more productive agents
- Improving customer satisfaction
- Increasing revenue with contact center insights
- Drastically lowering operational costs
Conclusion
It’s natural to desire a single platform that can handle voice and digital contacts in one place. But there’s good reason to be skeptical of omni-channel platforms, too: by definition, omni-channel platforms lack specialization. It’s the classic jack of all trades, master of none problem.
Worse, because voice is often more profitable to omni-channel vendors than digital, companies that offer omnichannel platforms often invest the bulk of their development resources in voice over digital, allowing their digital technology to lag behind.
As more customers look to digital to interact with companies, it’s more important than ever that you get digital right. Consider choosing a vendor who specializes in digital channels rather than a broader omni-channel approach.
Who is ASAPP?
ASAPP is the AI-native® software for contact centers.
We help customer service leaders unlock their full value by minimizing costs & inefficiencies, improving agent compliance & productivity, and surfacing actionable insights while helping you deliver a great customer experience.
Our customers are large enterprises who care deeply about leveraging AI to transform CX by delivering unprecedented cost savings and maximizing customer delight.
What do we make?
We make a full suite of AI-native solutions designed specifically for the needs and nuances of the CX industry.
Why we are the right partner?
We strive to be the best technology partner you have ever had.
ASAPP is not new to the AI or the CX space. We have been building AI-native products for the contact center since 2014 and building our own LLMs since 2018. We invest heavily in our products and our workforce to bring our customers the best solutions on the market and the subject matter experts to ensure those customers are getting the maximum benefit.
We offer white-glove service and insight into contact centers’ best practices across industries, and our consultative nature drives transformative results. ASAPP is laser-focused on business outcomes, data usability, and helping you realize your desired customer experience.
If you are interested in how best-of-breed digital software for your contact center can benefit your organization, please click below to schedule a consultation:
Speak to an Expert
Omnichannel solutions present themselves as an all-around answer but in reality their digital support options are severely lacking. What they offer in convenience, they often trade in effectiveness.
Call Center Voice Analytics: How to Understand Your Calls
Summary
From understanding customer issues to analyzing agent performance, voice analytics are crucial to operating an efficient, modern contact center.
From understanding customer issues to analyzing agent performance, voice analytics are crucial to operating an efficient, modern contact center.
But what exactly are call center voice analytics, and how can they be utilized to improve your contact center operations?
This blog post defines voice analytics and describes its potential benefits. Then, it walks through various use cases for voice analytics. Finally, it prescribes a framework for thinking through acquiring technology to improve your analytics.
Let's get started.
What is Call Center Voice Analytics?
Analytics is the process of "discovering, interpreting, and communicating significant patterns in data." In other words, analytics is the art of transforming raw data into usable metrics.
Call Center Voice Analytics, also known as Speech Analytics, is the process of transforming conversational data from voice interactions into meaningful metrics.
Typically, speech analytics software will utilize advanced computing techniques, like machine learning and natural language processing (NLP), to input all conversational data between agents and customers and output digestible metrics.
Voice analytics might include some of the following metrics:
- Average Handle Time
- Average Talk Time
- Average After-Call Work Rate
- Resolution Rate
- Repeat Caller Rate
- Intent Data
- Sentiment Data
- Custom Structured Data Reflecting Contents of Call
- Automated Summaries of Conversations
Real-time speech analytics enable contact center management to intimately understand how well their agents are servicing customers and accomplishing company objectives. In the next section, we break down the power of voice analytics for contact center management.
The Power of Voice Analytics
Voice analytics are to a contact center as the instrument panel is to a pilot.
With high-quality voice analytics, contact center management can keep their finger on the pulse of their organization. Below, we break down some of the use cases for live contact center analytics.
Use Cases for Voice Analytics
Quality Assurance and Performance Management
Voice analytics enable contact center management to understand the quality of the support its agents are providing. Analytics can help create a scorecard for agents, allowing management to see at a glance how agents are performing on a variety of metrics.
For example, a telcom company might need to weigh several variables when determining agents' performance. These variables might include average handle time, resolution rate, call back rate, and truck roll rate (the percentage of time a service truck is dispatched to a customer.) Voice analytics can compile all this data without manual intervention.
In addition, voice analytics can perform automated quality assurance work, like checking to determine if certain promos were offered, if policies around customer ID verification were followed, etc.
In short, voice analytics automates and simplifies quality assurance and performance management, while offering the ability for managers to gain visibility into a wide variety of metrics.

Compliance and Risk Management
Similar to the above, analytics on customer conversations can provide documentation for compliance and help companies manage risk.
For example, if a company is required by law to provide certain information for specific products (like a disclaimer for a financial product), voice analytics can provide evidence of agents' compliance.
In addition, analytics can serve as a powerful risk management tool. By creating flags that monitor automatically for likely fraud, potential discrimination, and more, a speech analytics tool can help companies proactively manage risk.
Analyze Contact Center Trends
Voice analytics can provide detailed information about customer issues, sentiment, satisfaction, and more by leveraging AI to analyze customer conversations.
By understanding exactly why customers are calling, companies can be proactive about resolving problems before they occur. In addition, contact centers might consider bolstering their bot flows to address common issues or creating automated internal processes to handle frequent requests more adeptly.
By utilizing the power of voice analytics, contact centers can identify trends as they occur and design intelligent interventions to improve customer experience.

Identify and Reduce Churn
Voice analytics can do more than report simple metrics; it can also be configured to run complex operations, such as analyzing customer sentiment and intent and categorizing issues.
One of the most powerful ways to configure voice analytics is to leverage the various analyses it provides to automatically identify potential churn.
For example, suppose analysis demonstrates that customers most likely to churn are those who call at least three times in a week, demonstrate negative sentiment, and use profanity in at least one call. Voice analytics software can flag every case with these qualities so management can determine the best way to intervene and reduce potential churn.
Of course, in a real-world application, the analysis can be much more complex. But the power of voice analytics is that it helps to predict problems before they occur, so management can be proactive rather than reactive.
Automate After-Call Work
The best voice analytics software leverages the power of AI to automatically summarize customer interactions. With powerful automated summaries, contact center agents can significantly reduce the amount of after-call work needed to document interactions.
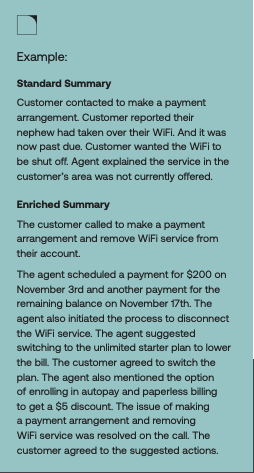
More, automated summaries can provide both structured and free text data. Structured data is standardized data, typically tabular with rows and columns, that clearly define data attributes. Best-in-class voice analytics software will automatically summarize call into defined, structured data that's relevant to operations (like competitors mentioned, whether a certain promo was offered, which products were discussed, etc.)
In addition, best-in-class voice analytics software can also provide informative free text summaries of conversations, enabling managers to quickly understand conversations without needing to dive through a transcript or rely on hurried agent notes.
With automated summaries, agents can significantly reduce the time spent on after-call work, and contact centers can receive enhanced summaries with the information they need to be effective.
How to Think About Voice Analytics Software
Voice analytics software is incredibly powerful. It provides management with a bird' s-eye view of the contact center's operations, quality assurance and performance management, helping to ensure compliance, aiding in identifying and reducing churn, and analyzing customer trends. Meanwhile, it can also improve agents' experiences by helping to automate summaries, enabling them to focus fully on the customer.
But how should contact centers think about purchasing the right voice analytics software for their company? Below, we highlight some key qualities speech analytics software should possess to provide as much insight as possible:
High-Quality Auto-Transcription
Low-accuracy transcription will hold back your voice analytics. Transcription is the core of any voice analytics solution. It is the technology that converts speech to text, so the software can accurately analyze the conversation.
But what is low accuracy?
The roughly 80% accuracy that many transcription solutions tout may sound like it is good enough. However, when 80% of the conversation is composed of simple words, it is the last 20% that holds the most critical, company-specific information you need to fill in the blanks.

If the solution you are considering is only capturing common words and phrases, you are going to miss out on the most valuable information, such as specific products, services, or policies mentioned, technical details, order or serial numbers, and more. With transcription, the real story is in the details. Make sure your solution captures the most important ones.
The right transcription solution will even let you identify and extract the most critical entities that you would like to glean from the transcript, such as order number, product name, date of transaction, dollar amount refunded/charged/credited, last four digits of account number, and competitor name.
Ability to Customize Insights & Dashboards
Most software offers cookie-cutter templates and insights that force companies to adhere to their definitions of what useful information to glean from customer conversations.
But every business is different, with unique use cases, nomenclature, and key issues to track.
The best software should enable your contact center to customize the valuable insights they want to glean from customer interactions.
Custom configurability should also extend to dashboards. Don't settle for pre-configured dashboards that limit your ability to understand what really matters. You should be able to customize dashboards to reflect the particular priorities and needs of management.
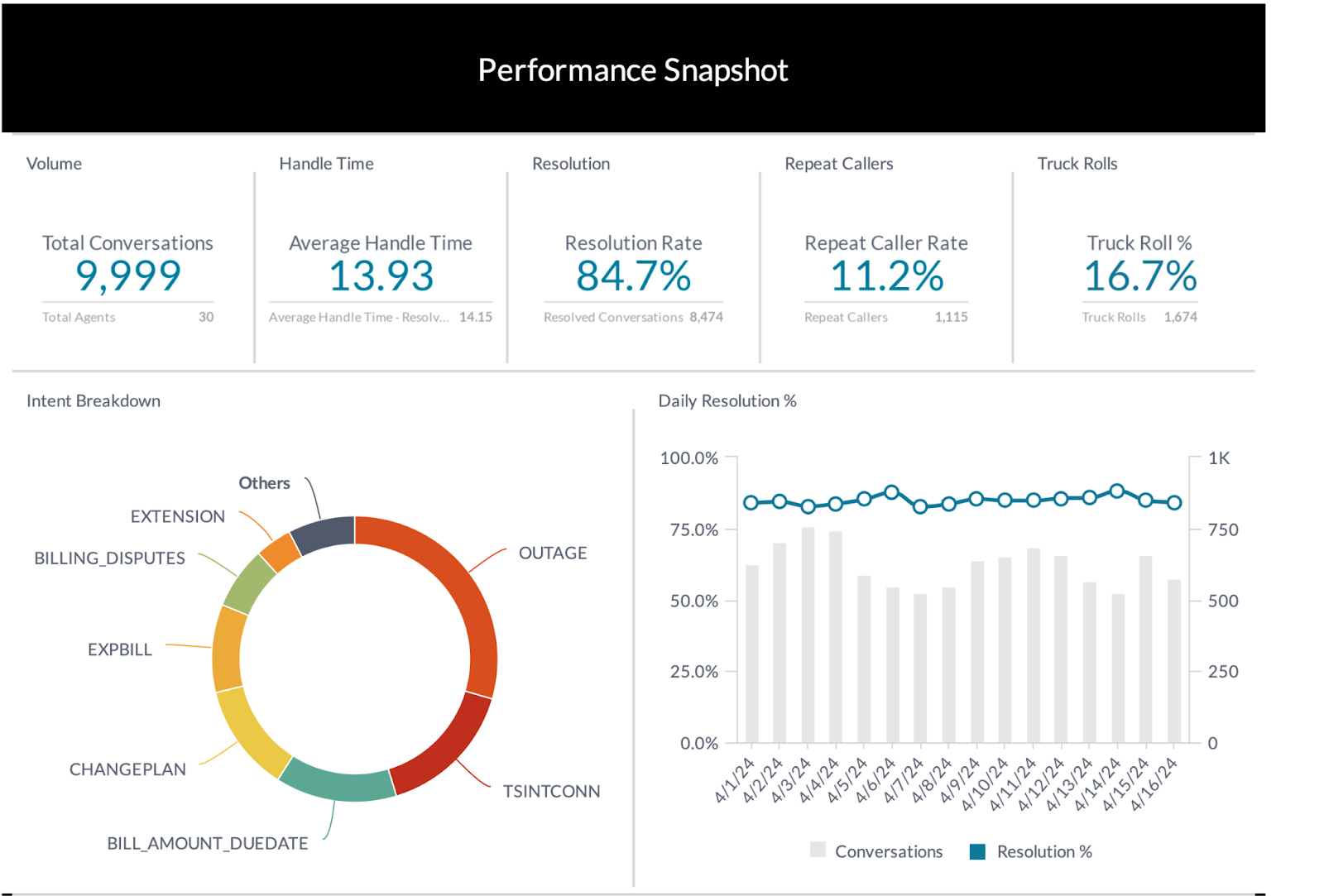
Generate Aggregated and Structured Data for Deep Analysis
Your summary data should guide corporate policymakers, provide insightful analytics, and be used to arm your agents. For it to truly do this, that data needs to be structured in the following categories for optimal use:
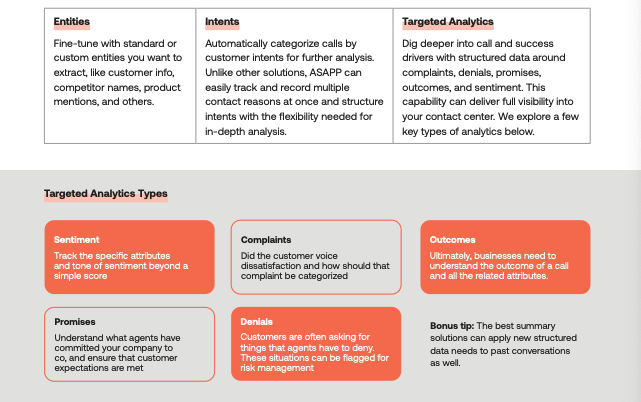
Integrations
Finally, any voice analytics software you choose should be able to integrate with the systems you use every day to access and manage customer data.
At a basic level, the software of your choice should be integrated with your CRM of choice, so it can automatically push automated summary data to the CRM without the need for additional agent actions.
In addition, the software should be compatible with your voice data, including audio via SIP call recording (SIPREC) protocol from contact center session border controllers (SBCs) or from live media stream services offered by major cloud telephony providers such as Amazon Connect (Kinesis), Twilio (Media Streams), and Genesys Cloud CX (Audiohook).
The right software should also be available both on-prem or as software-as-a-service.
In short, the best software is one that can mold itself to your infrastructure instead of forcing you to adapt to it.
Voice Analytics with ASAPP
Your conversation data is more valuable with ASAPP than any other solution. With our industry-leading structured data and enrichment capabilities, you will have more actionable insights and data discoverability than you thought possible - without any additional work for your agents. All this while dramatically reducing AHT, slashing ACW, and building a solid data foundation for all AI solutions to come.
ASAPP AutoSummary automates 100% of agents' after-call work. By combining human-readable and insights-ready summaries, AutoSummary can offload monotonous tasks for your agents and enable consistent, unbiased data across your contact center.
Beyond automatic and accurate summaries at scale, AutoSummary differentiates itself from other solutions by providing industry-leading capabilities around enrichment, structured data, configurable insights and dashboards, and flexible integrations.
In addition, ASAPP AutoTranscribe is the fastest and most accurate generative AI transcription solution purpose-built for CX. Only ASAPP delivers industry-leading speed and accuracy without compromising either.
Want more? Read our exhaustive buyer’s guide.
If you are sold on the need for the most powerful and configurable voice analytics software, then you need to know what to look for (and what to look out for) when evaluating solutions. Just “good enough” isn’t good enough when you are setting the foundation of intelligence for your CX organization.

This guide will help you understand the features and capabilities you need to keep in mind when evaluating solutions and the reasons why they are mission-critical for your organization. Read on to ensure you aren’t adding to your technical debt and that you are building a solid foundation for your AI journey with the right solutions.
Read the guide here.
Call Center ACW (After Call Work) 101: Streamlining Post-Call Processes
Introduction
Before diving into the solutions, let's take a moment to understand why ACW (After Call Work) matters in call center operations. ACW plays a crucial role in ensuring accurate record-keeping, compliance with regulations, and follow-up actions after customer interactions. It's the behind-the-scenes work that agents undertake to maintain high-quality customer service standards.
While these tasks are essential, they pose significant challenges for agents and contact center operations due to their ongoing conflict with the goal of reducing Average Handle Time (AHT). This natural tension between lowering AHT—a critical performance metric—and thoroughly completing all necessary tasks during ACW requires a delicate balance. Managing these competing priorities creates ongoing difficulties in optimizing contact center efficiency and effectiveness.
To address this, let’s explore the unique challenges of ACW, highlight the main contributors to ACW, and showcase how advanced generative AI is alleviating the tension between ACW and AHT.
Defining Call Center ACW
When we talk about ACW, we're referring to the tasks that agents must complete post-call. These tasks include updating customer records, logging call details, completing forms, and ensuring compliance with company policies and regulations.
Main Contributors to Call Center ACW
Now, let's break down the main factors contributing to ACW:
- Agent Notes: Agent notes are vital for capturing essential details of customer interactions. These concise summaries encompass key information discussed during the call, including customer inquiries, issues addressed, and actions taken. By meticulously recording this information, agents ensure continuity in service and provide valuable insights for future interactions.
- Dispositioning: This involves categorizing customer interactions based on predefined criteria. Agents assign disposition codes to each call, indicating its outcome and resolution status. These codes facilitate performance tracking, trend analysis, and data-driven decision-making, contributing to overall operational efficiency.
- Compliance Measures: Compliance measures ensure adherence to regulatory requirements and internal policies. Agents must comply with data privacy regulations, industry standards, and organizational policies when handling customer information. Adhering to compliance protocols maintains trust and integrity in customer interactions.
- Sending Surveys and Questionnaires: Surveys, questionnaires, and follow-up emails solicit feedback from customers post-interaction, gauging sentiment, measuring satisfaction, and fostering engagement. These responses provide actionable insights for refining service delivery and improving agent performance. But, it's important to note that surveys often don't always provide the most accurate picture. Agents can often choose whether to send a follow-up survey, potentially skewing results, and even when surveys are sent to all customers, only the most pleased or displeased typically respond, which can lead to an inaccurate representation of overall customer sentiment.
Common Challenges Go Beyond Time Constraints
One common, well-known challenge in ACW is the time-consuming nature of manual tasks, leading to delays in follow-up actions and reduced agent productivity. However, the challenges extend beyond time constraints and encompass various aspects of data management and analysis. Here are some additional challenges:
- Unstructured Handwritten Notes: Illegible or inconsistent handwritten notes hinder insights extraction.
- Data Silos: Disparate systems lead to data inconsistencies and hinder comprehensive analysis.
- Limited Insights: Traditional methods and low-performing summarization solutions may overlook nuances, limiting actionable insights.
- Manual Error and Compliance Risks: Manual processes increase the risk of errors and compliance breaches, impacting data integrity and regulatory compliance.
Benefits and Applications
Optimizing ACW processes can lead to significant benefits for call centers, including improved agent efficiency, enhanced customer satisfaction, and better compliance with regulatory requirements. By streamlining ACW tasks, call centers can deliver a more seamless and personalized customer experience.
Alleviating the Tension Between ACW and AHT
Advanced generative AI now has the power and nuance to effectively manage the tension between reducing Average Handle Time and completing essential After-Call Work tasks. These elevated automation capabilities raise the ceiling on what's possible with the majority of customer interactions. Generative AI can efficiently handle more complex customer issues with minimal human oversight, enabling agents to concentrate on addressing more intricate issues and engaging in higher-level tasks.
Generative AI's capacity to streamline processes not only reduces AHT but also ensures the thorough completion of crucial ACW tasks. This balanced solution optimizes contact center efficiency and effectiveness with proper escalation management, empowering agents to operate more efficiently with the support of generative AI.
Introducing AutoSummary: Slashing the ACW Processes
ASAPP's summarization solution, AutoSummary, is making a big impact in streamlining post-call tasks and creating rich information for analytics and operational enhancements. AutoSummary automates the summarization of customer interactions, reduces ACW processes, provides real-time operational feedback, and empowers agents to work more efficiently while solving customer problems faster.
Key Benefits of AutoSummary
- Real-time Summarization: By analyzing interactions in real-time, AutoSummary quickly extracts key insights as customer interactions unfold, enabling prompt response and improved service delivery.
- Seamless Integration: Summarized information seamlessly integrates with the call center's CRM system, eliminating manual data entry and ensuring smooth information flow across the organization.
- Customization Options: AutoSummary offers extensive customization options, allowing call centers to tailor the summarization process to their specific needs, whether by prioritizing specific metrics or keywords or customizing summarization criteria based on industry-specific requirements.
- Scalability and Reliability: Designed for scalability, AutoSummary ensures consistent and dependable performance even amidst fluctuating call volumes or evolving business needs, providing uninterrupted support for critical ACW tasks.
- Unprecedented Business Insights: AutoSummary enables you to understand why your customers are calling, giving you the ability to identify automation opportunities, analyze promotion and cross-sell effectiveness, and respond to anomaly detection.
Conclusion
Call Center ACW (After Call Work) is a critical aspect of call center operations, and understanding it is essential for success. With the right tools and knowledge, call centers can streamline ACW processes, improve agent efficiency, and deliver exceptional customer experiences.
Ready to dive deeper into the world of ACW and learn how to optimize your call center operations? Check out our eBook, “The Modern CX Guide to Summaries.” In it, we explore advanced strategies for reducing ACW and improving overall efficiency and unpack how modern solutions to summaries and dispositioning can slash ACW.
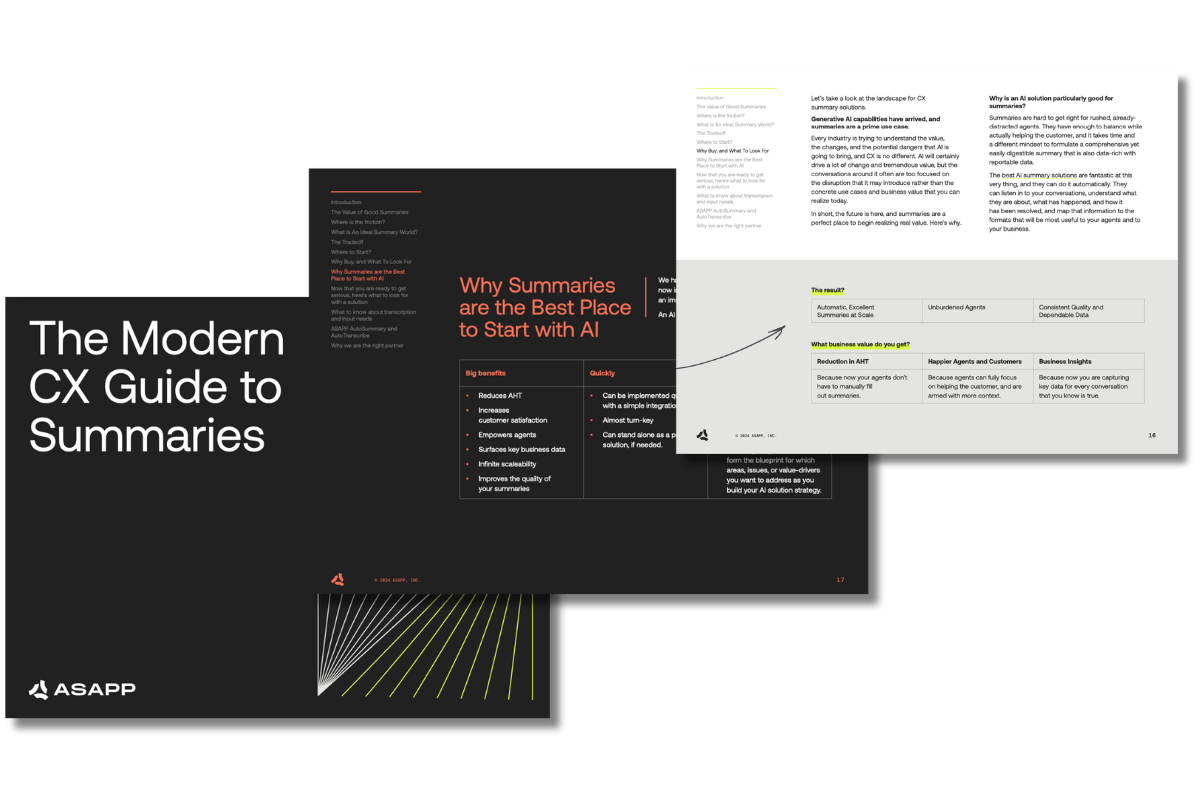
Read the eBook
AHT Meaning: What is Average Handle Time?
What is AHT and How Does It Impact Your Business?
Author Name: LANE HARTMAN
Summary
Understand AHT Meaning (Average Handle Time) and its impact on contact center efficiency and customer satisfaction. Learn how to calculate AHT and how to reduce it.
Average Handle Time (AHT) is a key contact center metric. In short, AHT measures the average time of a customer interaction.
AHT provides crucial information about contact center efficiency, staffing needs, and customer experience. And as such, AHT is one of the essential metrics for contact centers to track.
In this blog post, we define AHT and show you how to calculate it. Then, we discuss six impacts reducing AHT can have on your organization. Finally, we provide recommendations for how to reduce AHT while optimizing customer experience.
What is AHT?
Average Handle Time (AHT) is a core call center KPI that measures the average time for an agent to handle a customer interaction from start to finish.
AHT encompasses the entirety of an agent's interaction with a customer issue, including total talk time, hold time, and after-call work (ACW).
How to Calculate AHT
The standard formula to calculate AHT is adding total talk time, hold time, and after-call work and dividing by the total number of interactions.
Talk Time: The time an agent spends actively engaging with a customer
Hold Time: The time an agent spends while a customer is on hold
After-Call Work: The time spent on work that needs to be completed after a call or interaction before the next interaction can start.
In formula form, AHT is calculated as:
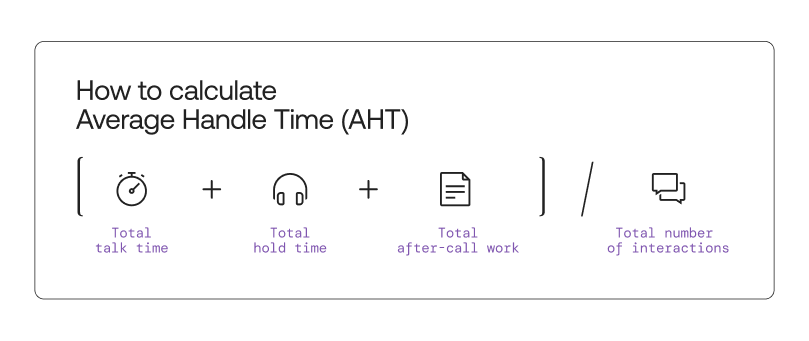
Why is AHT Important?
Average Handle Time is one of the most important KPIs for any contact center to track because it impacts both contact center efficiency and customer experience.
Contact Center Efficiency
Average Handle Time distills the time spent across interactions and averages it by the amount of interactions. This one metric impacts the entire contact center organization. Understand AHT and understand how to do the following:
Determine Staffing Loads
Understand how many agents are necessary to handle expected case loads. The lower your AHT, the higher the total number of calls your agents can handle in a given time period.
Benchmark Within the Industry
Compare your AHT to standards across your industry to understand your relative performance.
Benchmark Internal Improvement
Measure your AHT over time to understand if your contact center is more efficiently handling issues, satisfying customers, and effectively utilizing resources.
Understand Individual Performance
AHT can be helpful for analyzing both overall contact center performance and individual performance. While other call center metrics, like customer satisfaction and containment rate are essential to contextualizing the entire picture, AHT can be helpful to understand an agent's capacity and efficiency.
Analyze Gaps
If your AHT is higher than expected, it can be helpful to analyze the individual components of the metric (talk time, hold time, after-call work) to determine where your contact center might need the most improvement.
With this information in hand, you can invest in additional training or update your technology stack with modern software that improves agent efficiency and reduces hold time and after-call work.
Customer Experience
Customers want their issues to be resolved quickly and efficiently. AHT is one of several metrics that helps inform management about how quickly customer issues are being handled. A low AHT implies the following for customers:
Shorter Wait Times
Nobody likes waiting on hold. Reducing AHT by reducing the time customers spend on hold or in a transfer improves customer experience.
Faster Issue Resolution
AHT is a proxy for the time it takes to resolve a given issue. Reducing AHT means customer's issues are being resolved more quickly.
Heightened Customer Satisfaction
Shorter wait times and faster issue resolution contribute to greater customer satisfaction. Work to improve AHT to ensure your customers are having their issues meaningfully resolved quickly.
How to Reduce AHT
Monitor AHT
What you pay attention to grows.
If you want to reduce AHT, the first step is to monitor AHT.
Then, set realistic goals, supported by process and technology changes, to reduce AHT over time.
Modern CX software, like ASAPP, can help you automatically track metrics like AHT over time.
Enhance Self-Service with Modern Virtual Agents
The most efficient way to reduce AHT is to help customers resolve an issue without needing an agent’s support.
But true self-service is more than simply putting a manual’s worth of information online and making it searchable through a help function.
Modern self-service design should leverage modern virtual agents that go beyond simplistic chatbots to handle a broader array of issues, provide more realistic responses, and solve more customer issues before an agent ever needs to get involved.
Augment Agents with the Information They Need
To reduce AHT, contact centers should provide agents with the tools they need to facilitate resolutions quickly and meaningfully.
Digital features that can augment agents include:
- Surfacing Meaningful Information for Agents Automatically
- Automatic Processes Agents Can Leverage to Save Time
- Concurrency Management Tools
- Auto Complete and Auto Suggestion Functions to Help Finish Thoughts Faster
- Automated Workflows to Quicken Common Processes
- Automated Check-Ins to Ensure Clients in the Queue are Still Present
Implement Auto Summaries to Free Up Time
One of the largest contributors to talk time and after-call work is time spent taking notes and writing call summaries.
The time required to take these notes can often be around 10% of the actual call duration and is one of the greatest contributors to high AHT.
With such a large percentage of time consumed by agents taking notes, contact centers are put between a rock and a hard place. If they press agents to reduce their ACW by taking notes during a phone call, it can often lead to prolonged pauses that reduce customer satisfaction. On the other hand, if they ask agents to reduce overall time spent taking notes, it can lead to gaps in summaries that might impact other agents down the road and make it difficult for supervisors to have good visibility.

In short, traditionally, AHT has been difficult to reduce without reducing customer satisfaction. In particular, attempts to reduce ACW by shifting notes into phone calls can either increase talk time or reduce customer satisfaction to an extent that can undermine the initiative altogether.
But now, with modern CX technology, like ASAPP, contact centers can both reduce AHT and increase customer satisfaction.
ASAPP AutoSummary leverages AI to automatically take notes and summarize calls, so agents can focus on the clients. Importantly, ASAPP AutoSummary creates accurate, data-rich, and easily accessible summaries that contact centers and their agents can trust.
With AutoSummary, companies can significantly reduce their AHT and ACW while enhancing the customer experience. In fact, in one case study, ASAPP AutoSummary reduced after-call work from 10% of call duration to just 3% of call duration.
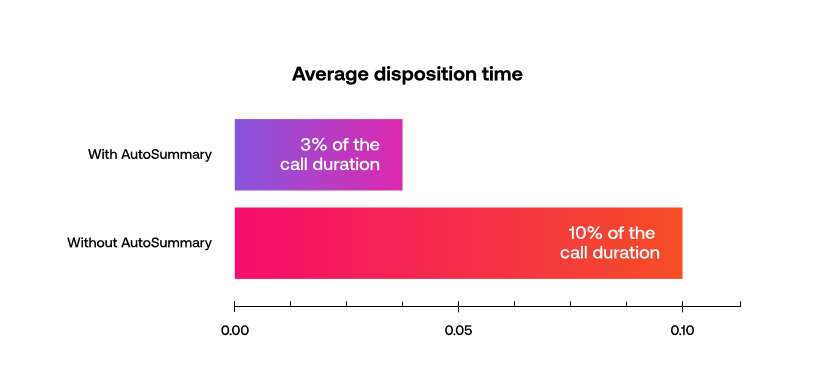
Interested in Learning More About How New Technology is Impacting CX Summaries?
Struggling with agent notes, lengthy after-call work, questionable questionnaires, cumbersome compliance checklists, lack of context for calls, and a lack of definitive understanding of WHY your customers are calling you?
The answer to all of these struggles is the ability to have good summaries at scale.
Unfortunately, you are likely not getting all your business needs from them. Worse yet, they distract your agents from customer conversations and add crucial time to your AHT. This eBook is here to help.
This eBook will cover the best practices and technologies that will deliver the value that good summaries promise without undue agent distraction. Readers will learn:
- What good summaries look like and contain.
- Best practices for summarization - regardless of your methods.
- How new technologies, like generative AI, can both eliminate the need for manual entry and enrich summaries with all necessary vital details.
- What to look for when evaluating summarization technologies.
- How to ensure you are collecting valuable business insights from your summarization efforts.
Download eBook Now
Consider Leveraging Generative Agents
The future is here.
ASAPP GenerativeAgent™, AI-powered chat and voice agents, can empathize with customers, understand complex problems, and access internal APIs and processes to provide helpful answers or initiate actions to solve user problems (with company approval, of course.)
Use GenerativeAgent™ to resolve issues before an agent needs to become involved. GenerativeAgent™ can substantially reduce AHT by drastically reducing the time agents spend on interactions and minimizing after-call work. By enabling greater concurrency, Generative Agents increase the number of calls handled in a given period.
Interested in how GenerativeAgent™ could transform CX? Read more about GenerativeAgent™ here.
Reducing AHT with ASAPP
Who is ASAPP?
ASAPP is the AI-native software for contact centers, and ASAPP exists to end bad customer service.
We help customer service leaders unlock their full value by minimizing costs & inefficiencies, improving agent compliance & productivity, and surfacing actionable insights while helping you deliver a great customer experience.
Our customers are large enterprises who care deeply about leveraging AI to transform CX by delivering unprecedented cost savings and improving customer satisfaction.
What do we make?
We make a full suite of AI-native solutions designed specifically to help companies reduce AHT and improve customer experience.

Conclusion:
Mastering Average Handle Time (AHT) is essential to operate efficient contact centers and enhance customer experience. By understanding AHT and implementing key strategies to reduce it, contact centers can drive efficiency and delight customers.
Want to learn more?
Transcription and Summarization solutions are not only the foundation of CX intelligence; they are a foundational capability for your AI strategy as well, so you better choose the right ones.
This guide is here to help.
Not all transcription and summarization solutions are built the same. While some may seem “good enough,” those same products can introduce crippling technological debt, may hamper the effectiveness of your other technology solutions, and may fail to surface the discoverable and actionable insights that you were hoping to achieve.
If you are serious about finding the right transcription and/or summarization solution to reduce AHT, this guide will help you understand:
- Exactly what features and capabilities you need in a transcription and summarization solution, and why they are important
- The specific business value the right solution will bring to your organization - from foundational capabilities to reduced AHT and improved customer satisfaction
- A clear and actionable buyer’s checklist to help you with your search
Download eBook Now
GenerativeAgent™ can substantially reduce AHT by drastically reducing time agents spend on interactions and minimizing after-call work.
AutoTranscribe is Best-in-Class Transcription: Here's Why That Matters
AutoTranscribe is Best-in-Class Transcription: Here’s Why That Matters
To deliver exceptional customer experiences at scale, it's critical to recognize the indispensability of accurate, fast, automated transcription. Serving as the cornerstone for comprehending customer needs and preferences at scale, high-quality transcription captures the nuances of every interaction, ensuring no critical insights are lost in translation. This foundational capability empowers contact centers to make well-informed decisions, boost operational efficiency, and provide unmatched customer satisfaction.
As enterprises grow and customer expectations evolve, the need for top-notch transcription solutions becomes even more pronounced. Quality transcription improves data integrity and enables organizations to extract actionable insights from vast amounts of customer interaction data. With the right transcription solution, contact centers can transform data swamps into navigable data lakes, laying the groundwork for future AI endeavors.
ASAPP AutoTranscribe
In recognizing the critical importance of quality transcription for driving superior customer experiences, ASAPP developed AutoTranscribe with a keen focus on accuracy, speed, and scalability. By understanding that precise transcription forms the bedrock of effective customer interactions, ASAPP AutoTranscribe stands out as a solution meticulously crafted to meet the unique demands of modern CX environments. With its commitment to high accuracy, unparalleled performance, tailored CX models, and rigorous evaluation processes, AutoTranscribe ensures that organizations can harness the power of transcription to elevate their contact center operations and deliver exceptional customer satisfaction.
Why Accuracy and Speed are Paramount
When you are thinking about the performance of transcription solutions, the two metrics that matter the most are accuracy and speed. Here’s why.
Every Word Counts
Most transcription services handle basic conversations well, capturing over 80% of conversations that involve simple words and phrases. However, the real challenge lies in the remaining 20%—the complex, company-specific information critical to understanding and action. AutoTranscribe shines here, offering unparalleled accuracy in transcribing the nuanced and evolving language found in live, human-to-human interactions.
Every Second Counts
In dynamic live interactions, whether it's a call or chat, every second counts. ASAPP AutoTranscribe stands out as the fastest transcription service, enabling agents to quickly access transcribed content and provide real-time support to customers. This translates into instantaneous responses and seamless information processing, ensuring that interactions progress seamlessly and efficiently.
The most accurate transcription
So you won’t miss the key details showing you exactly what happened in every call
89%+ Accuracy
The fastest transcription
Because real-time problem-solving requires real-time transcription
60ms Latency
Best-in-Class Performance
Focusing on contact centers, AutoTranscribe is built on a foundation of real-world data, utilizing actual calls between agents and customers in its training data. This specialized approach allows for best-in-class performance, significantly outpacing generic transcription services.
Each deployment is fine-tuned to the client's specific domain, achieving a word error rate (WER) of less than 11% with continuous improvements. The technology boasts low-latency performance, generating text in less than 60ms from the end of an utterance, ensuring conversations are captured seamlessly and accurately.
Industry-leading accuracy
Low-accuracy, high-latency transcription is holding back your contact center.
80% accuracy may sound like it is good enough. However, when 80% of the conversation is composed of simple words, it is the last 20% that holds the most critical, company-specific information you need to fill in the blanks.
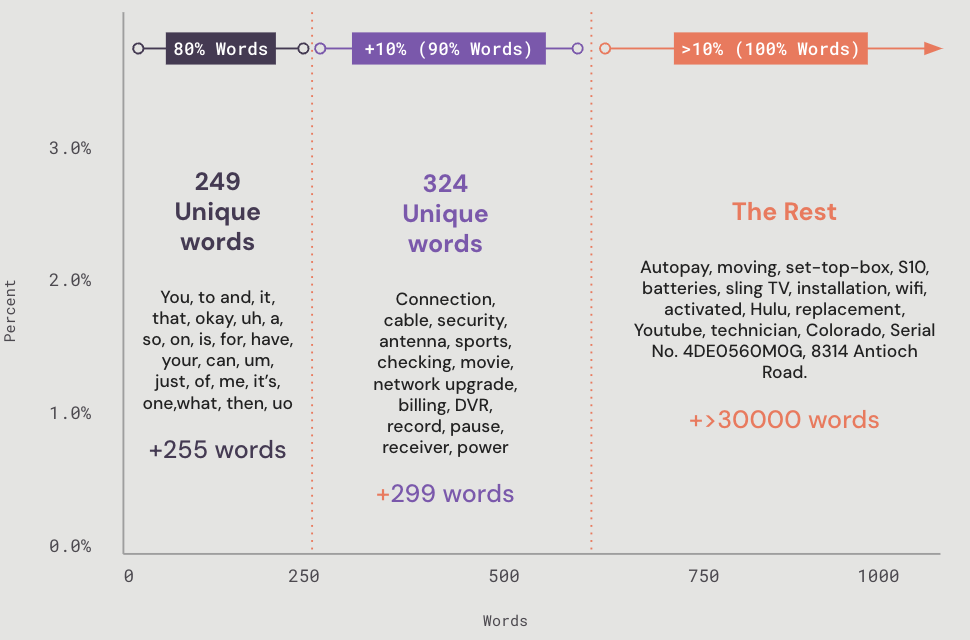
Tailored Models for Your Business
The practical benefits of adopting AutoTranscribe are numerous and impactful. For contact centers, the solution's real-time audio processing capability integrates smoothly with standard telephony systems and supports live audio streams, simplifying implementation. By creating and maintaining models that are uniquely tailored to each business's specific needs, AutoTranscribe ensures that critical vocabulary and phrases are accurately captured, enhancing the customer service experience.
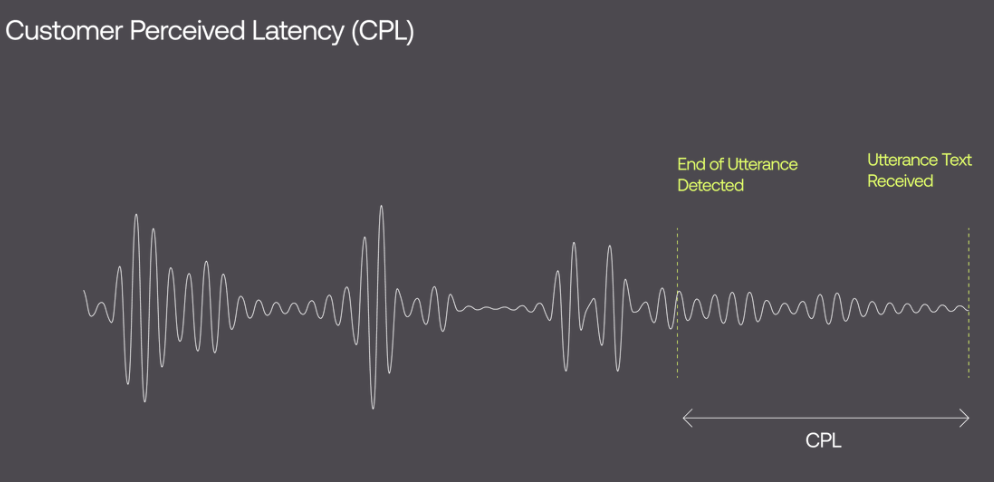
Real-time transcription
Real-time problem-solving requires real-time transcription.
In a live call or chat scenario, speed is of the essence. ASAPP AutoTranscribe impressively achieves remarkably low latency, enabling human and virtual agents to access transcribed content, swiftly enhancing their efficiency, enabling real-time responses and information processing.
Rigorous Evaluation
ASAPP's commitment to ongoing research and development means that AutoTranscribe clients benefit from the latest advances in speech recognition technology, continually improving accuracy and performance. This dedication to excellence and customization makes AutoTranscribe not just a tool but a strategic asset for enhancing the quality and effectiveness of contact center operations.
Your CX future depends on having the right transcription solution.
To realize the value of CX AI technology, highly accurate, real-time transcription should be your first step. Otherwise, your agents, AI solutions, and bots won’t have the information they need when they need it, and the benefits you may be expecting from generative AI solutions will be stunted.
If you have plans to evolve the effectiveness and efficiency of your CX organization, then only AutoTranscribe will do.
Ready to Dive Deeper?
For comprehensive insights into CX transcriptions and their transformative potential, download our eBook "The Modern CX Guide to Transcription." Explore best practices, technologies, and solutions to revolutionize CX with accurate transcription. Elevate agent efficiency, gain valuable business data, and harness the full potential of customer interactions.
Transform your CX strategy with the right transcription solution.
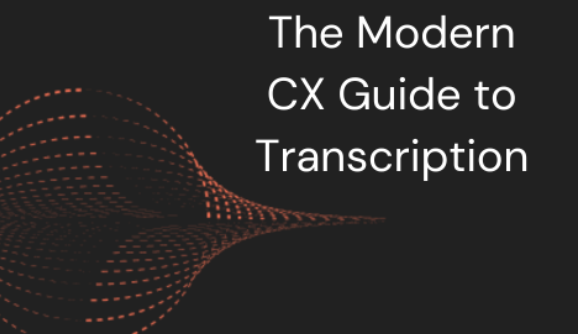
About ASAPP
ASAPP is the AI-native software for contact centers, and ASAPP exists to end bad customer service. We help customer service leaders unlock their full value by minimizing costs & inefficiencies, improving agent compliance & productivity, and surfacing actionable insights while helping you deliver a great customer experience. Our customers are large enterprises who care deeply about leveraging AI to transform CX by delivering unprecedented cost savings and maximizing customer delight.
Want to learn more about ASAPP and how they can help your team? Request a Demo
What to Look for in a CX Transcription Solution
What to Look for in a CX Transcription Solution
Excellent, automatic transcription is mission-critical for digital CX transformation if you are looking to:
- Build the foundation to automate summaries or agent notes
- Capture business intelligence from all of your customer interactions
- Augment your agent capabilities in both digital and voice channels
- Better understand and address agent effectiveness
- Easily extract certain phrases, intents, and entities from all of your transcripts
- Lay the foundation for all future CX AI initiatives
Speed and Accuracy Matter Most
If you’re aligned with those aims, then the number one thing you need to look for is a transcription solution with no tradeoff between speed and accuracy. Here’s why:
High speed: Transcriptions can help both during and after the call. Your agents need to be able to see exactly what is said as it’s being said, clearing up confusion or accounting for different accents. They also need to be able to use that real-time data in other AI solutions like agent augmentation tools. So don’t compromise. High-speed, low-latency transcription is essential.
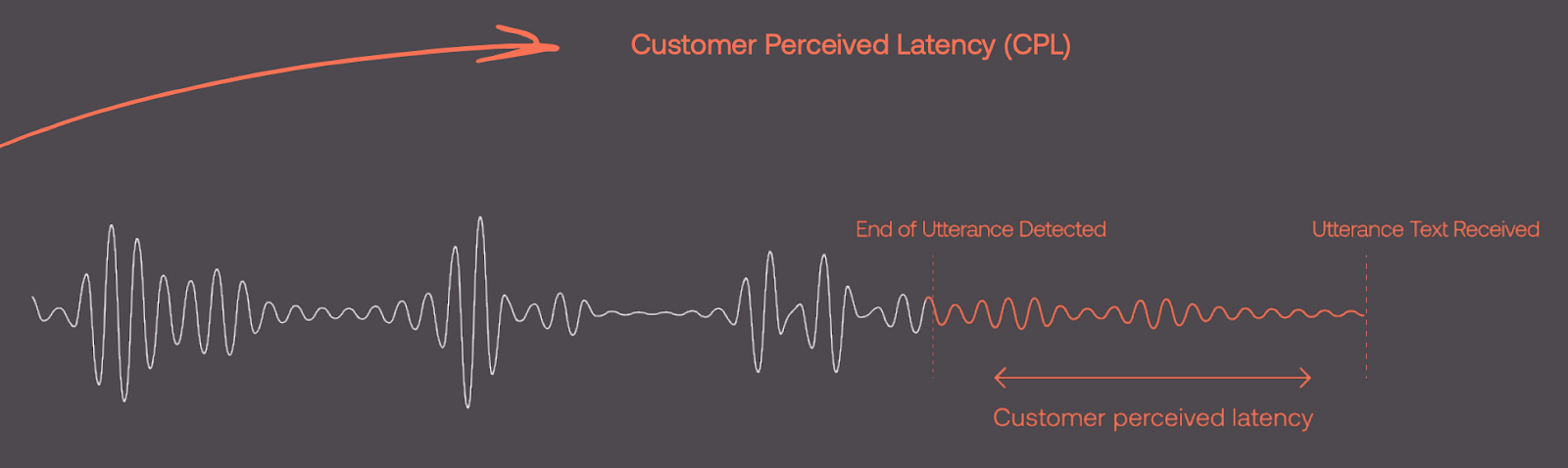
High accuracy: Most transcriptions get the easy stuff right and can capture over 80% of single words and phrases, but what is left over is the most complex and critical information. Make sure your transcription solution can track not only exactly what was said but also who said it.
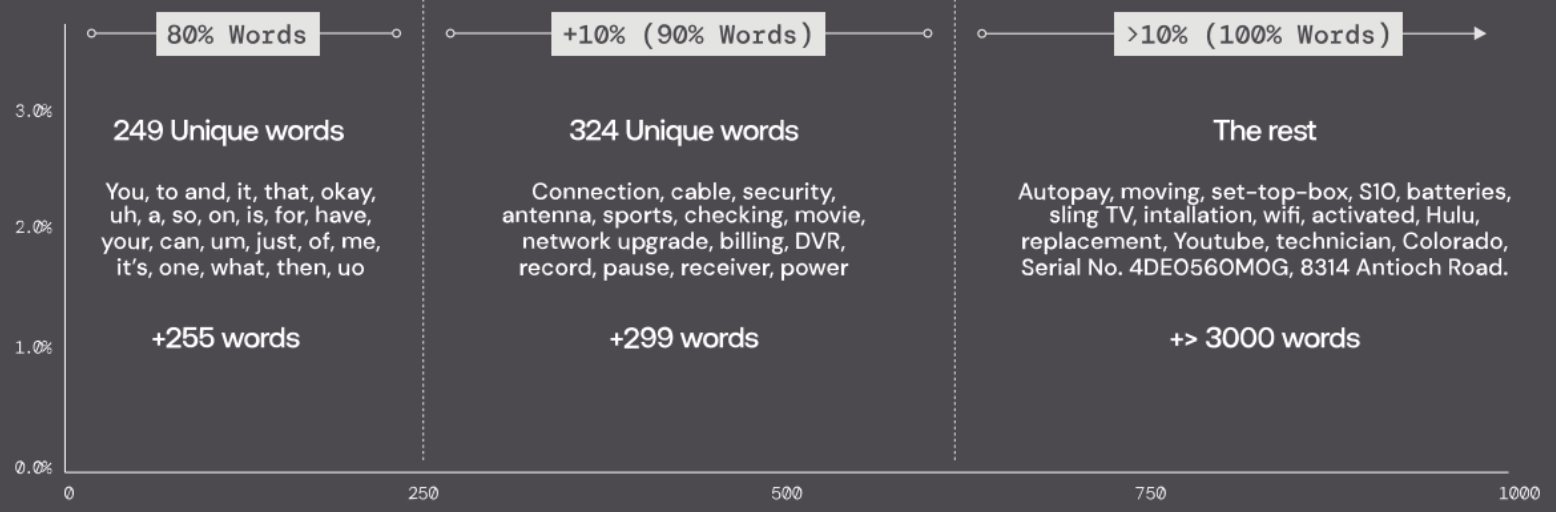
Understanding the critical significance of both speed and accuracy, we purposefully engineered AutoTranscribe to excel in both aspects.
Customization Capabilities
Speed and accuracy are paramount, but don't overlook the importance of customization capabilities. Once you have optimal speed and accuracy, focus on the solution's ability to adapt to the unique needs of your business. This ensures that your transcription solution remains efficient and effective, even as requirements evolve over time. Since transcription forms the bedrock of much of your CX technology stack, invest in a solution that's future-proof and won't require frequent replacements.
Introduction to AutoTranscribe
ASAPP AutoTranscribe is unmatched in the transcription landscape, offering high accuracy, best-in-class performance, tailored models, and rigorous evaluation.
With accuracy exceeding 89% and a latency of just 60ms, AutoTranscribe ensures no detail is missed in every call, which is crucial for both understanding and action.
Built on real-world data, AutoTranscribe achieves a word error rate (WER) of less than 11%, far surpassing generic services. Its tailored models adapt to each business's needs, capturing critical information accurately.
Real-time transcription capabilities enable swift problem-solving, enhancing agent efficiency and enabling real-time responses. ASAPP's commitment to excellence ensures continuous improvement, making AutoTranscribe a strategic asset for contact center operations.
Ready to Dive Deeper?
For comprehensive insights into CX transcriptions and their transformative potential, download our eBook "The Modern CX Guide to Transcription." Explore best practices, technologies, and solutions to revolutionize CX with accurate transcription. Elevate agent efficiency, gain valuable business data, and harness the full potential of customer interactions.
Transform your CX strategy with the right transcription solution.
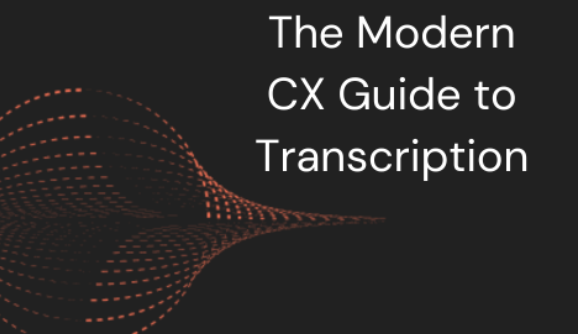
About ASAPP
ASAPP is the AI-native software for contact centers, and ASAPP exists to end bad customer service. We help customer service leaders unlock their full value by minimizing costs & inefficiencies, improving agent compliance & productivity, and surfacing actionable insights while helping you deliver a great customer experience. Our customers are large enterprises who care deeply about leveraging AI to transform CX by delivering unprecedented cost savings and maximizing customer delight.
Want to learn more about ASAPP and how they can help your team? Request a Demo
CX Transcription 101
CX Transcription 101
The importance of highly accurate, fast, and automated transcription cannot be overstated. It enables organizations to unlock invaluable insights from customer interactions and is the bedrock for effectively leveraging AI technologies.
What is Transcription?
In the context of CX, transcription is the process of converting spoken interactions between customers and agents into accurate, textual representations. This entails capturing not just the words spoken but also the nuances and subtleties of each conversation, including tone, emotion, and context. These nuances are essential for understanding the intricacies of customer interactions, allowing businesses to tailor their responses and CX strategies effectively.
Good Transcriptions Create Value
Good transcription transcends the mere conversion of speech to text, which is essential for effective communication, customer satisfaction, and detailed analytics. Every customer interaction holds valuable insights that drive improvement and innovation. Accurate transcription captures the nuances of conversations, ensuring data integrity and empowering your organization to confidently make informed decisions.
Good data leads to good decisions, which pave the way for effective strategies and strong customer relationships. When organizations have confidence in the data underlying their decisions, it leads to more focused actions, better customer experiences, and streamlined operations.
Key Concepts and Insights to Get You Started

Transcription as the Base for Future AI Endeavors
Your CX success hinges on selecting the right transcription solution. Without highly accurate, real-time transcription, your agents, AI solutions, and bots will lack the timely information necessary for effective operation. For instance, a low word error rate (WER) is crucial for precise entity extraction, vital in tasks like customer service chats or financial transactions. Entities such as order numbers, product names, and sensitive information require a WER of <10% for accurate extraction, ensuring reliable data processing.
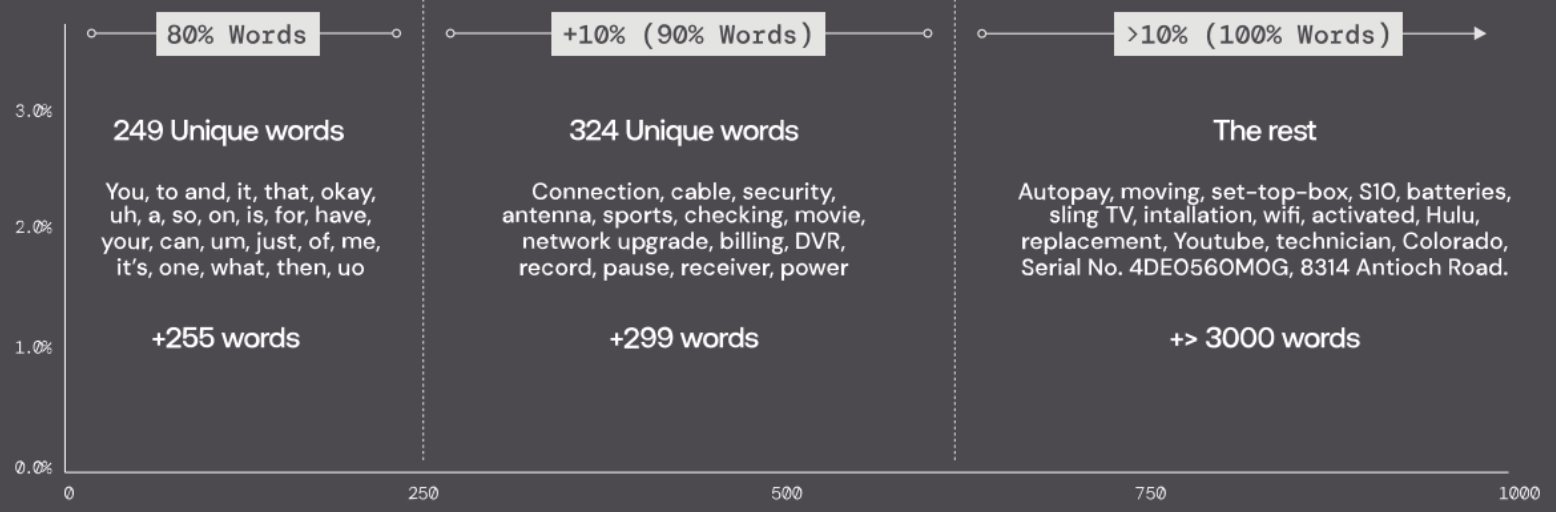
Quality transcription forms the cornerstone for advanced AI applications like natural language processing (NLP), sentiment analysis, and predictive modeling. Without accurate transcription, your AI solutions will lack the necessary data to function effectively, hindering their ability to automate responses, predict trends, and tailor interactions with customers.
Ready to Dive Deeper?
For comprehensive insights into CX transcriptions and their transformative potential, download our eBook "The Modern CX Guide to Transcription." Explore best practices, technologies, and solutions to revolutionize CX with accurate transcription. Elevate agent efficiency, gain valuable business data, and harness the full potential of customer interactions.
Transform your CX strategy with the right transcription solution.
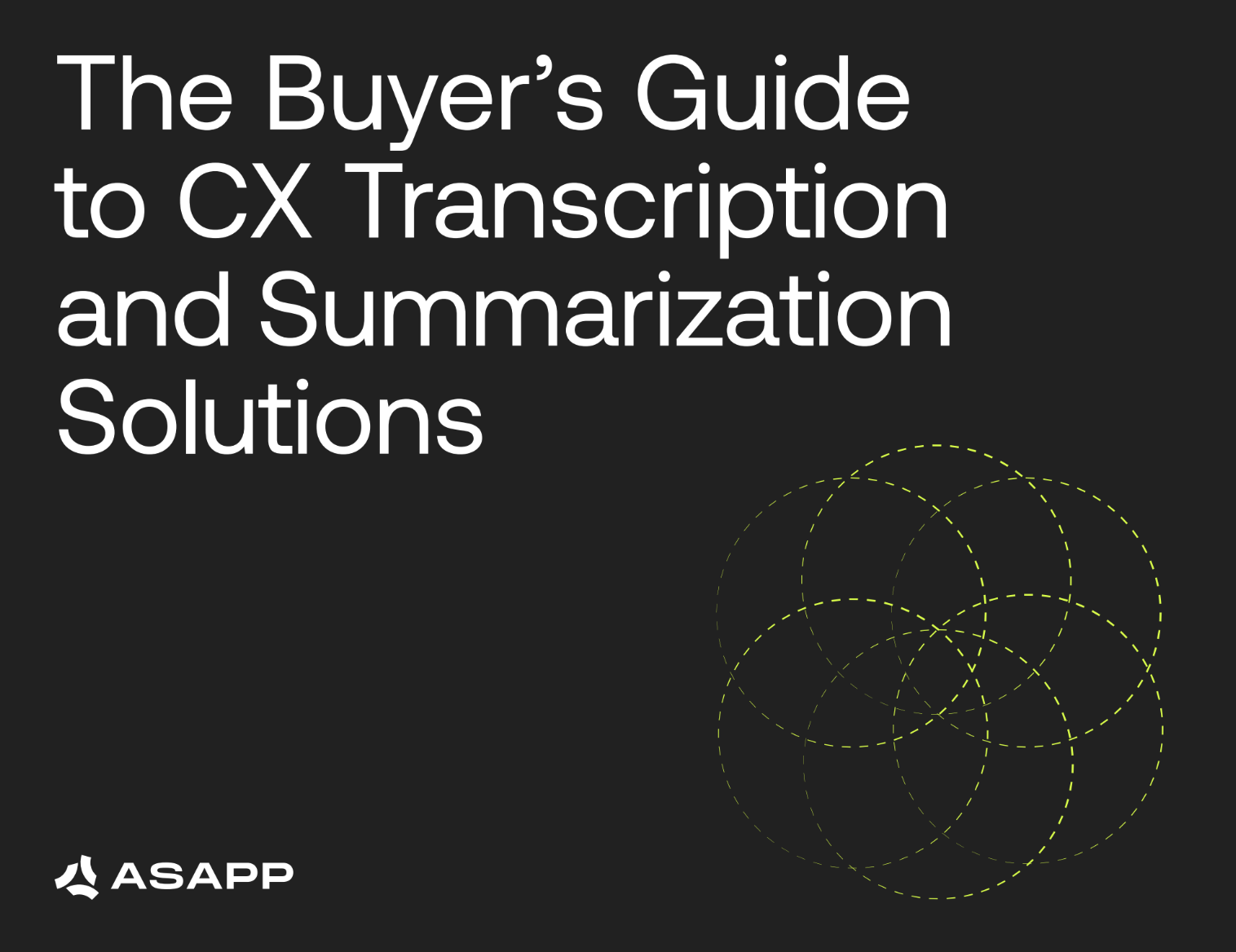
About ASAPP
ASAPP is the AI-native software for contact centers, and ASAPP exists to end bad customer service. We help customer service leaders unlock their full value by minimizing costs & inefficiencies, improving agent compliance & productivity, and surfacing actionable insights while helping you deliver a great customer experience. Our customers are large enterprises who care deeply about leveraging AI to transform CX by delivering unprecedented cost savings and maximizing customer delight.
Want to learn more about ASAPP and how they can help your team? Request a Demo
Introducing ASAPP GenerativeAgent™, the future of CX
We are proud and thrilled to announce a revolutionary new product the likes of which enterprises have never seen before, GenerativeAgent™, a product that automates what was previously impossible to automate with our fully conversational generative AI voice and chat agent.
GenerativeAgent™ is a generative AI product that can automate 90%+ of contact center interactions. This revolutionary technology can handle complex interactions, helping customers more efficiently and effectively than ever before. Furthermore, it unleashes a new era of productivity by allowing agent concurrency for voice interactions–introducing a new paradigm for contact centers.
"This product isn't just groundbreaking—it's revolutionary, redefining what we thought was possible in what is likely the largest automation opportunity in the world today," said Gustavo Sapoznik, Founder and CEO, ASAPP.
GenerativeAgent™ delivers:
Dramatic business savings:
- Greatly reduces the call volume to your agents
- Raises the ceiling of what you can automate
- Accurate, flexible, and secure
Increased customer satisfaction with:
- No time wasted
- No channel switching
- No transfers, for a seamless experience
GenerativeAgent™ is always online and ready to speak with your customers.
What does GenerativeAgent™ solve?
For far too long, companies have been spending fortunes that result in the alienation and frustration of customers; all the while, the agents in these contact centers have one of the highest attrition rates of any job. Technology so far has provided a few band-aids to aid in the problem of delivering excellent customer service at scale and at cost. Still, the reality is that nothing but incremental value has been delivered for the better part of the past forty years.
These incremental gains in capability over the years have resulted in an industry that is stuck in an unfortunate tradeoff where companies have to place themselves on a spectrum ranging from cost-effective yet poor customer service to prohibitively expensive and excellent customer service. All the while, frontline agents have been crushed beneath the sheer volume of interactions and tasks to accomplish, resulting in some of the highest turnover rates of any occupation. The truth is that employees were not built to thrive in this environment, and customers were not built to endure this environment.
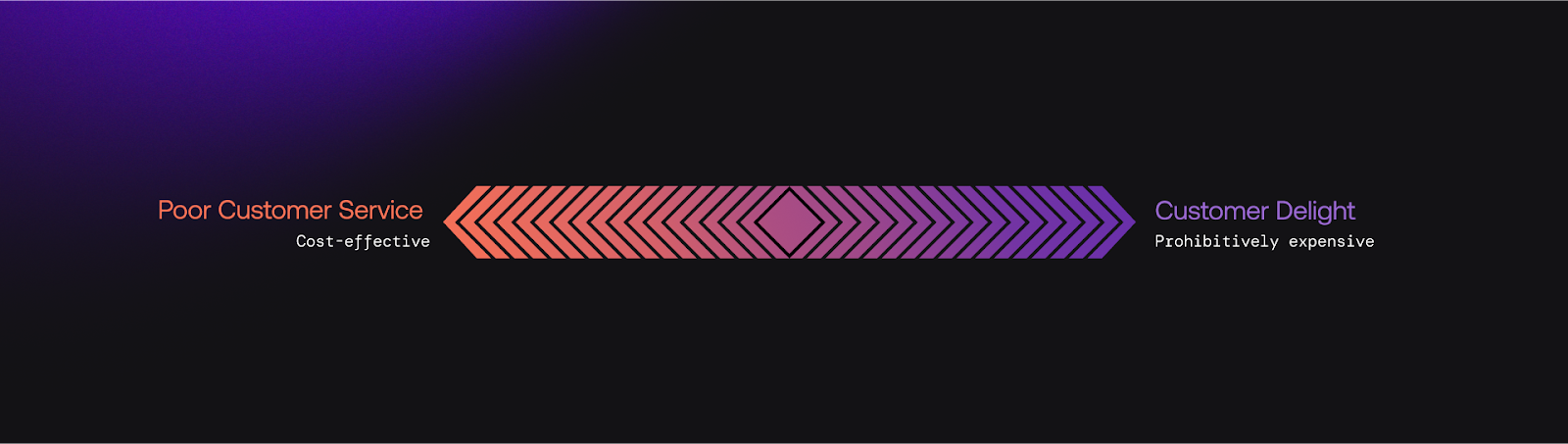
GenerativeAgent™ finally breaks this tradeoff by allowing companies to deliver excellent customer service at scale while freeing up human agents to focus more fully on higher-tiered interactions and problem-solving.
“Organizations no longer have to make a trade-off between great customer experience and saving money. With ASAPP, doing what’s best for the company is now doing what’s best for the customer.” - Gustavo Sapoznik, Founder and CEO, ASAPP
What is GenerativeAgent™?
GenerativeAgent™ is a fully capable generative AI voice and messaging agent. It is a completely autonomous system that automates interactions currently assigned to human agents. This results in a radically improved customer experience across all interaction channels and substantial business savings.
ASAPP's decade-long AI research and creation of state-of-the-art products has culminated in the breakthrough that is GenerativeAgent™. The product is:
- Fully generative: The system is grounded on the content of your APIs and knowledge systems. No manual dialogue design is needed as GenerativeAgent™ autonomously interacts with an enterprise's backend systems.
- Actively learning: This includes a human-in-the-loop interface for escalations, allowing GenerativeAgent™ to learn from agents' actions when their involvement is required while enabling voice agent concurrency.
- Safe and secure: It is grounded in your policies, procedures, and desired controls, satisfying the strictest enterprise security and compliance requirements.
- Seamlessly implemented: GenerativeAgent™ integrates natively into existing IVR, messaging, and telephony systems.
With GenerativeAgent™, customers will be immediately connected with a capable, conversational, and flexible helper that will solve many of their questions or issues dependably and efficiently. Agents will be able to oversee multiple interactions at once, and can handle all escalations in real-time through GenerativeAgent™.
Want to learn more and see it in action? Watch the full-length keynote presentation here.
Safety and security with GenerativeAgent™
None of these groundbreaking capabilities matter if you cannot trust it to deliver the right information to your customers, stay within your policies, and faithfully reflect your brand.
We built GenerativeAgent™ to be not only the most capable generative AI agent on the market but also the safest and most trustworthy. Read on to see just some of the steps that we have taken and the guardrails that we have built to ensure that GenerativeAgent™ can respond reliably, effectively, and safely to your customers.
Grounded in your knowledge center and APIs
GenerativeAgent™ leverages integrations into your APIs to retrieve data and take actions that are specific to each customer, enabling hyper-personalized automation. Your knowledge center is used to answer all questions about policies, products, or services and technical issues while maintaining up-to-date responses.
Cutting-Edge Language Models (LLMs)
ASAPP utilizes state-of-the-art Language Learning Models (LLMs) renowned for their exceptional performance in (1) adhering to prescribed instructions, (2) deciphering potentially conflicting or vague customer input, and (3) mitigating the risk of generating inaccurate or unintended responses, ensuring utmost privacy and data integrity.
Built-in Safety Protocols
ASAPP integrates robust safety protocols designed to assess the safety of incoming inputs. Any queries deemed potentially unsafe are promptly flagged and rejected, safeguarding user privacy and security.
Thorough Testing Procedures
ASAPP subjects GenerativeAgent™ to internal and external evaluations conducted by both ASAPP personnel and trusted customers, ensuring that all interactions yield safe and reliable outcomes, thereby upholding our commitment to privacy and safety.
Agent Connectivity and Human Oversight (Human in the Loop)
ASAPP's infrastructure facilitates seamless connectivity between agents and human operators, ensuring that GenerativeAgent™ receives timely assistance and oversight whenever encountering queries beyond its scope, thereby enhancing the accuracy and privacy of responses, with all escalations being handled through GenerativeAgent™.
Legal Assurance
GenerativeAgent™ has been developed using, and will continue to be updated using, and will function consistent with, the present and future standards, guidelines, protocols, and policies applicable to, governing, or addressing AI, offering assurance to users regarding the protection and responsible handling of their data
Want to learn more about GenerativeAgent™?
Visit the product page here.
Meet GenerativeAgent™ - request a demo here.
About ASAPP and our journey to GenerativeAgent™
ASAPP is an artificial intelligence cloud provider committed to solving how enterprises and their customers engage. Inspired by large, complex, and data-rich problems, ASAPP creates state-of-the-art AI technology that covers all facets of the contact center. Leading businesses rely on ASAPP's AI Cloud applications and services to multiply Agent productivity, operationalize real-time intelligence, and delight every customer.
GenerativeAgent™ is the latest in ASAPP's suite of generative AI customer experience products, including AutoSummary, AutoTranscribe, and the ASAPP Messaging platform. Fortune 500 organizations, including four of the top five cable providers, 50% of the leading wireless companies, two of the four largest airlines, and other leading enterprises across banking, insurance, retail, and technology, currently use ASAPP's state-of-the-art products and technology.
ASAPP’s journey to GenerativeAgent™ is the result not of following the technology trend and hastily incorporating “AI” on top of or into legacy products but of envisioning what products and technology should be and then doing the required hard work to make it happen. This work has resulted in over 100 patents, dozens of publications in top AI journals, and a continued development of the technology required to make our vision come to life over the past 10 years.